Few-shot classification of façade defects based on extensible classifier and contrastive learning
Automation in Construction(2022)
摘要
Façade defect classification based on deep learning has made great progresses in recent years. However, deep learning models commonly need abundant labeled data for training, and it could be impractical and expensive to collect sufficient labeled samples for all classes of defects. Sometimes, there are only a few samples in rare classes, which are not able to support the training process. In addition, common classifiers based on deep learning cannot easily extend their recognition classes and thus cannot classify unseen classes with only a few samples. Therefore, to overcome the problem of insufficient data and the extension constraint of the classifier, a few-shot classification method based on an extensible classifier and contrastive learning is proposed to recognize unseen classes with limited (1, 2 or 5) samples. The extensible classifier implemented by imprinting weights can easily extend the model to classify unseen classes with a few samples. Meanwhile, contrastive learning, which is a complementary task in training, is used to enrich the model’s generalization and representation on unseen classes. Besides, a hard negative mining (HNM) method is introduced to address the imbalanced data in contrastive learning and further improve accuracies. Experimental results demonstrate that the proposed method improves the few-shot classification accuracy with only 1 sample from 35.8% to 63.5% on novel and unseen classes, and from 73.1% to 82.1% on all classes, while maintaining a high and comparable accuracy (89.6%) on base classes.
更多查看译文
关键词
Façade defects,Few-shot learning,Extensible classifier,Contrastive learning
AI 理解论文
溯源树
样例
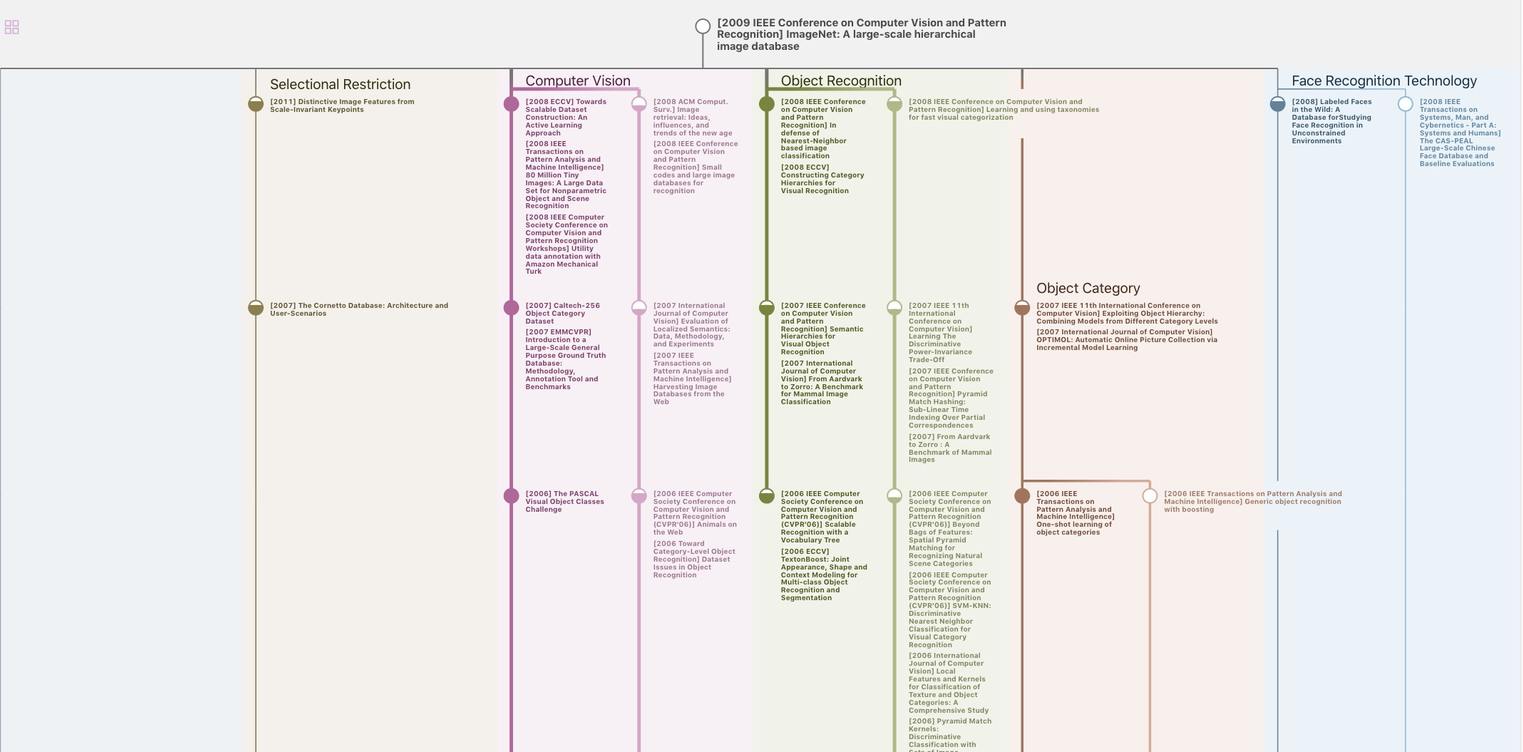
生成溯源树,研究论文发展脉络
Chat Paper
正在生成论文摘要