Design of biased random walks on a graph with application to collaborative recommendation
Physica A: Statistical Mechanics and its Applications(2022)
摘要
This work investigates a paths-based statistical physics formalism, inspired from the bag-of-paths framework, for the design of random walks on a graph in which the transition probabilities (the policy) are biased in favor of some node features. More precisely, given a weighted directed graph G and a non-negative cost assigned to each edge, the biased random walk is defined as the policy minimizing the expected cost rate along the walks while maintaining a constant relative entropy rate. As for the standard bag-of-paths and the randomized shortest paths frameworks, the model assigns a Gibbs–Boltzmann distribution to the set of infinite walks and allows to recover known results from the literature, derived here from a different perspective. Examples of quantities of interest are the partition function of the system, the cost rate, the optimal transition probabilities, etc. In addition, the same formalism allows the introduction of capacity constraints on the expected node visit rates and an algorithm for computing the optimal policy subject to such capacity constraints is developed. Simulation results indicate that the proposed procedure can be effectively used in order to define a Markov chain driving the walk towards nodes having some specific properties, like seniority, education level or low node degree (hub-avoiding walk). An application relying on this last property is proposed as a tool for improving serendipity in collaborative recommendation, and is tested on the MovieLens data.
更多查看译文
关键词
Network data analysis,Biased random walk,Bag-of-paths model,Collaborative recommendation
AI 理解论文
溯源树
样例
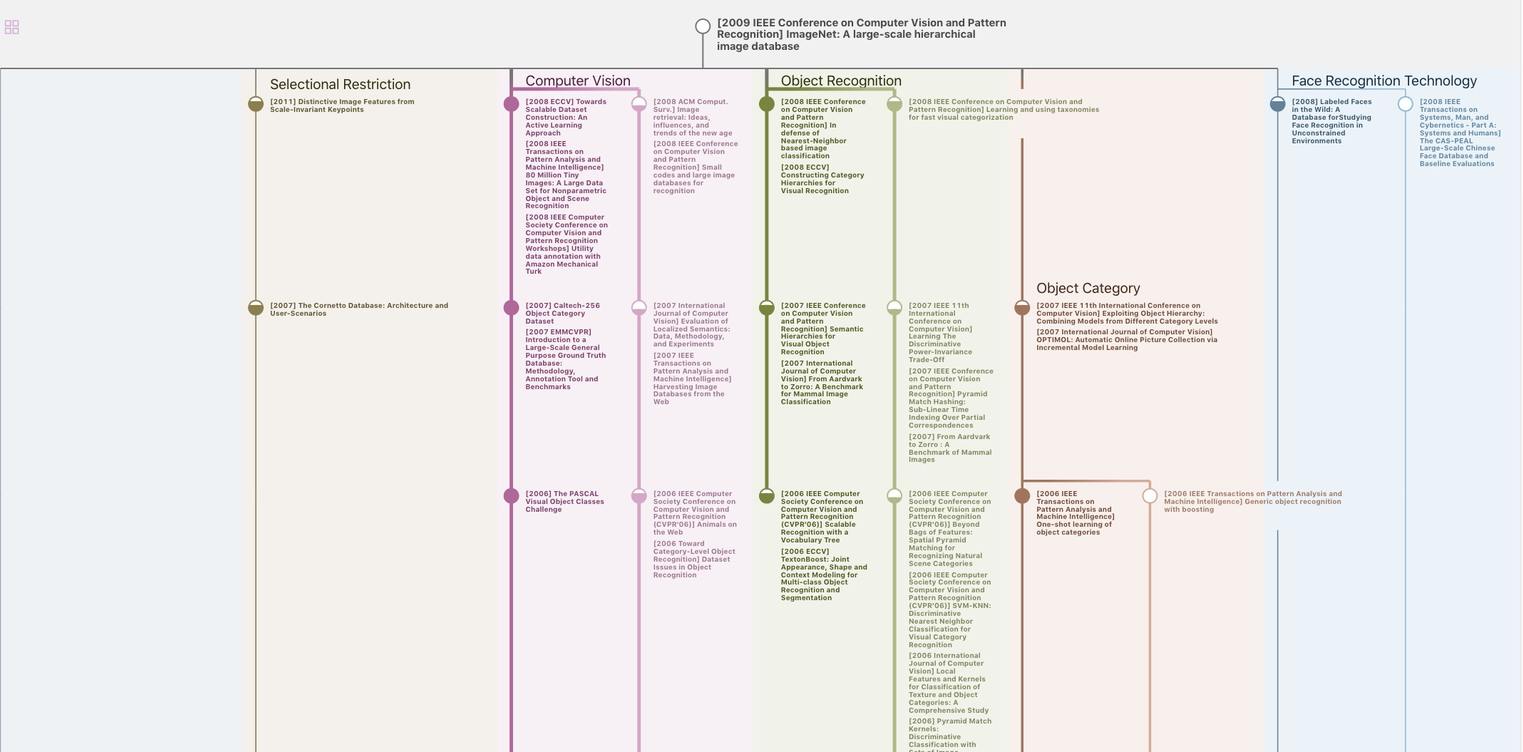
生成溯源树,研究论文发展脉络
Chat Paper
正在生成论文摘要