Bone age assessment and sex determination using transfer learning
Expert Systems with Applications(2022)
摘要
Bone age is an important index in the measurement of the biological maturity in children. Although many machine learning methods have been developed to classify bone radiographs, a number of limitations like the need for large datasets still exists. In this paper, we propose an efficient method based on convolutional neural networks to automatically predict bone age given radiographs. The proposed approach considers the differences between male and female skeletal development in order to classify the sex from radiographs, in conjunction with the use of a bone age assessment (BAA) network to significantly improve the prediction performance of bone age. The dataset used is from the 2017 Pediatric Bone Age Challenge, which contains 12,611 left-hand radiographs. Pre-processing of the radiographs is first performed, followed by detection of key-points of the hand for the application of affine transformation to register the hand pose. Transfer learning is then applied to the sex determination and BAA models with the pre-trained weights on ImageNet, to leverage on the generic features from millions of real-world images. Three BAA models were trained on three separated portions of the dataset, and utilized with the sex information to obtain the bone age. Results indicate that the method outperforms existing BAA methods as the mean absolute error (MAE) obtained was 5.31 months (0.44 years) while an accuracy of 94.7% was achieved for the sex determination. When sex labels were provided for the testing radiographs, the MAE of our method was further reduced to 4.68 months (0.39 years). The advantage of our method is that it eliminates the need for hand crafting features and utilizes sex as an important information in the prediction of bone age.
更多查看译文
关键词
Bone age assessment,Sex determination,Radiographs,Deep learning,Transfer learning
AI 理解论文
溯源树
样例
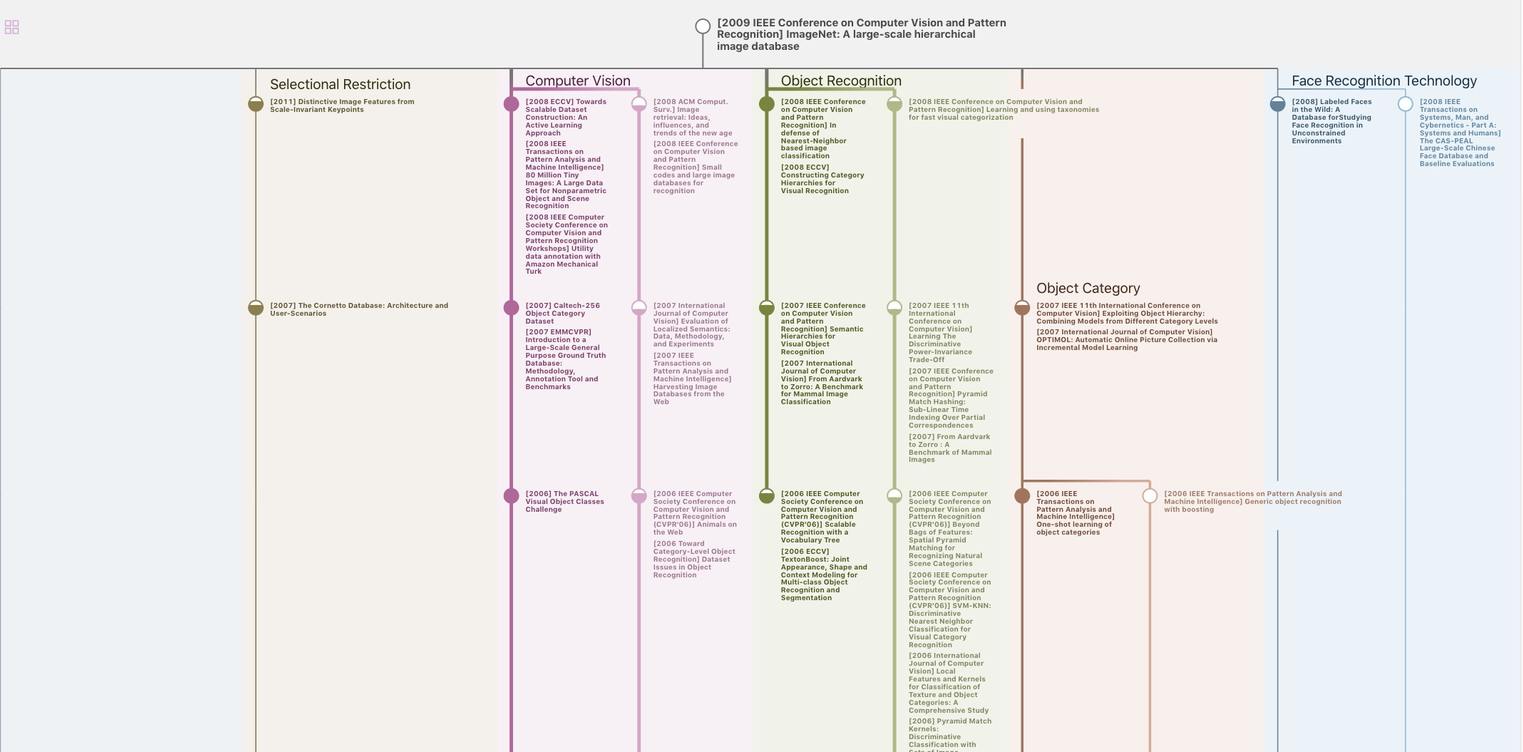
生成溯源树,研究论文发展脉络
Chat Paper
正在生成论文摘要