Remaining useful life prediction using composite health index and hybrid LSTM-SVR model
QUALITY AND RELIABILITY ENGINEERING INTERNATIONAL(2022)
摘要
Remaining useful life (RUL) plays an important role in prognostic and health management to reduce maintenance costs and avoid possible accidents. Massive multi-sensor data makes it challenging to extract degradation features and predict RUL. This paper develops a novel RUL prediction framework consisted of a multi-sensor fusion model and a hybrid prediction model. HI curves are constructed by synthesizing multiple metrics including time correlation, monotonicity, robustness, and consistency, making our data fusion method different from existing methods considering only one metric. In many practical situations, the initial degradation levels of obtained HI curves are different, and treating all curves without classification will result in errors in RUL prediction. The K-means method is used to partition degradation paths into discrete states and the obtained HI curves are grouped by their initial states and then trained separately. A hybrid prediction model combining Long Short-Term Memory (LSTM) network and Support Vector Regression (SVR) is developed to predict the RUL. The dataset of turbofan engines is used to verify the proposed method. The results show that the proposed method performs better than many existing methods.
更多查看译文
关键词
data fusion, health index, long short-term memory, remaining useful life, support vector regression
AI 理解论文
溯源树
样例
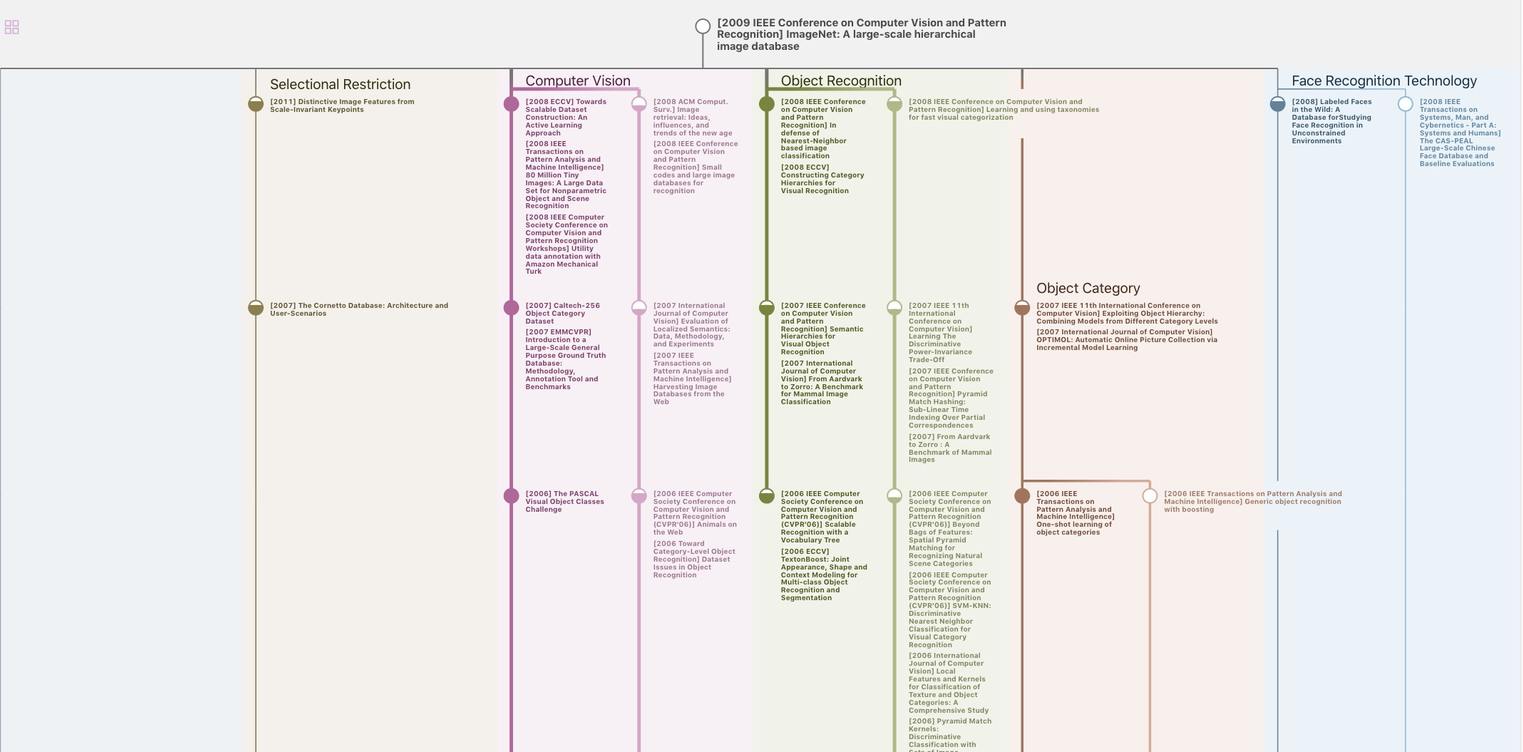
生成溯源树,研究论文发展脉络
Chat Paper
正在生成论文摘要