Multisource Data Integration and Comparative Analysis of Machine Learning Models for On-Street Parking Prediction
SUSTAINABILITY(2022)
摘要
Searching for a free parking space can lead to traffic congestion, increasing fuel consumption, and greenhouse gas pollution in urban areas. With an efficient parking infrastructure, the cities can reduce carbon emissions caused by additional fuel combustion, waiting time, and traffic congestion while looking for a free parking slot. A potential solution to mitigating parking search is the provision of parking-related data and prediction. Previously many external data sources have been considered in prediction models; however, the underlying impact of contextual data points and prediction has not received due attention. In this work, we integrated parking occupancy, pedestrian, weather, and traffic data to analyze the impact of external factors on on-street parking prediction. A comparative analysis of well-known Machine (ML) Learning and Deep Learning (DL) techniques, including Multilayer Perceptron (MLP), Random Forest (RF), Decision Trees (DT), K-Nearest Neighbors (KNN), Gradient Boosting (GA), Adaptive Boosting (AB), and linear SVC for the prediction of OnStreet parking space availability has been conducted. The results show that RF outperformed other techniques evaluated with an average accuracy of 81% and an AUC of 0.18. The comparative analysis shows that less complex algorithms like RF, DT, and KNN outperform complex algorithms like MLP in terms of prediction accuracy. All four data sources have positively impacted the prediction, and the proposed solution can determine the best possible parking slot based on weather conditions, traffic flow, and pedestrian volume. The experiments on live prediction showed an ingest rate of 0.1 and throughput of 0.3 events per second, demonstrating a fast and reliable prediction approach for available slots within a 5-10 min time frame. The study is scalable for larger time frames and faster predictions that can be implemented for IoT-based big data-driven environments for on-street and off-street parking.
更多查看译文
关键词
smart city applications, Internet of Things, predictive analytics, on-street parking prediction
AI 理解论文
溯源树
样例
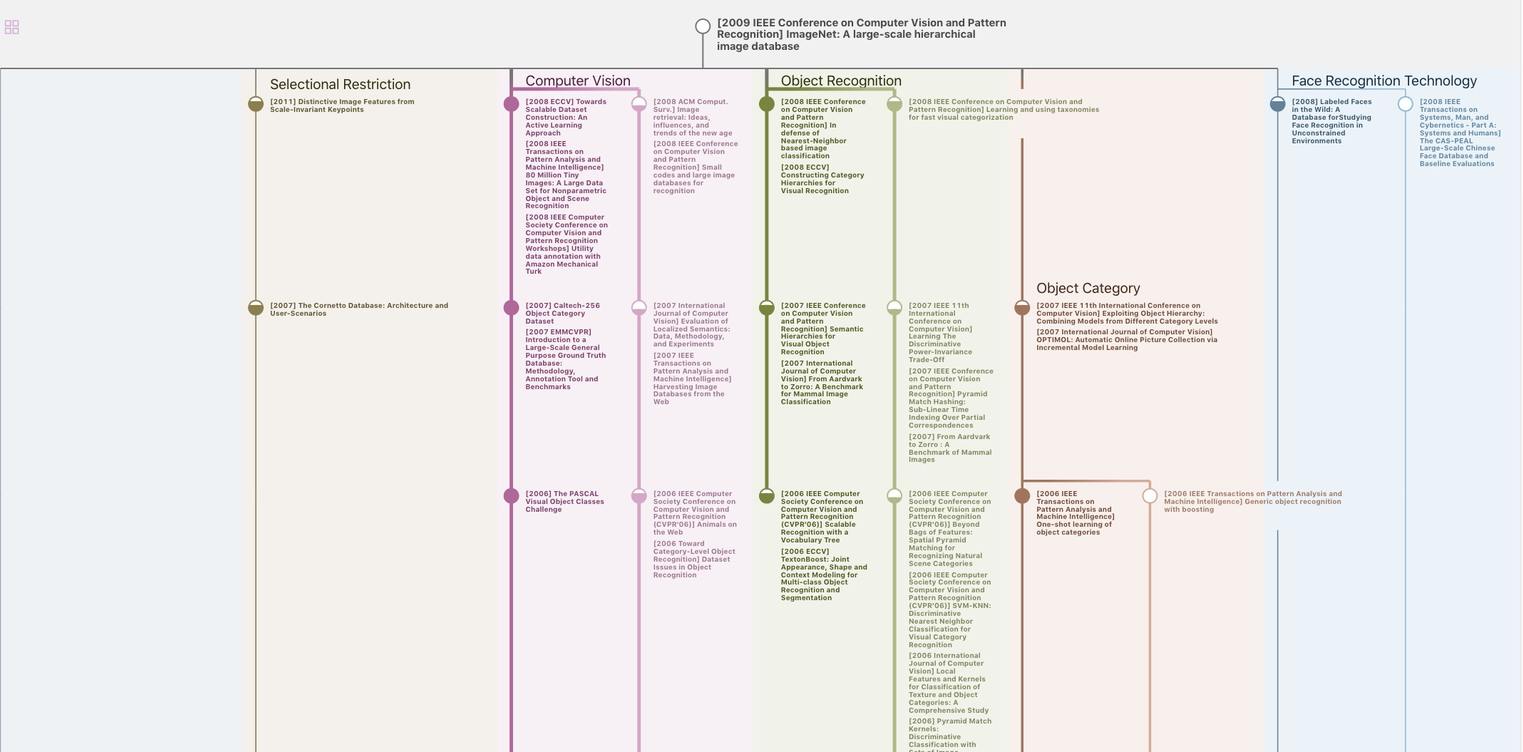
生成溯源树,研究论文发展脉络
Chat Paper
正在生成论文摘要