PABAFT: Channel Prediction Approach Based on Autoregression and Flexible TDD for 5G Systems
ELECTRONICS(2022)
摘要
To achieve high gains from multiple antennas in 5G systems, the base station (gNB) constructs precoders using channel measurements obtained based on pilot signals. For high user mobility, the measurements quickly become outdated, which is especially crucial for ultra-reliable low latency communications (URLLC) traffic because it increases channel resource consumption to provide highly reliable transmissions and, consequently, reduces system capacity. Frequent pilot transmissions can provide accurate channel estimation and high-quality precoder but lead to huge overhead. Fortunately, 5G systems enable flexible time division duplex (TDD), which allows the gNB to dynamically change the configuration of downlink and uplink slots and tune the period of channel measurements. The paper exploits this feature and designs a new prediction approach based on autoregression and flexible TDD (PABAFT) that forecasts the channel between consequent pilots transmissions. To learn fine-grained channel properties, the gNB configures uplink pilot transmission in each slot. When the training data are collected, and the model is fitted, the gNB switches back to the regular slot configuration with a long pilot transmission period. Extensive simulations with NS-3 in high-mobility scenarios show that PABAFT provides the signal-to-noise ratio (SNR) close to that with the ideal knowledge of the channel at the gNB. In addition, PABAFT significantly reduces channel resource consumption and, thus, increases capacity for URLLC traffic in comparison to the existing solutions.
更多查看译文
关键词
massive MIMO, URLLC, channel adaptation
AI 理解论文
溯源树
样例
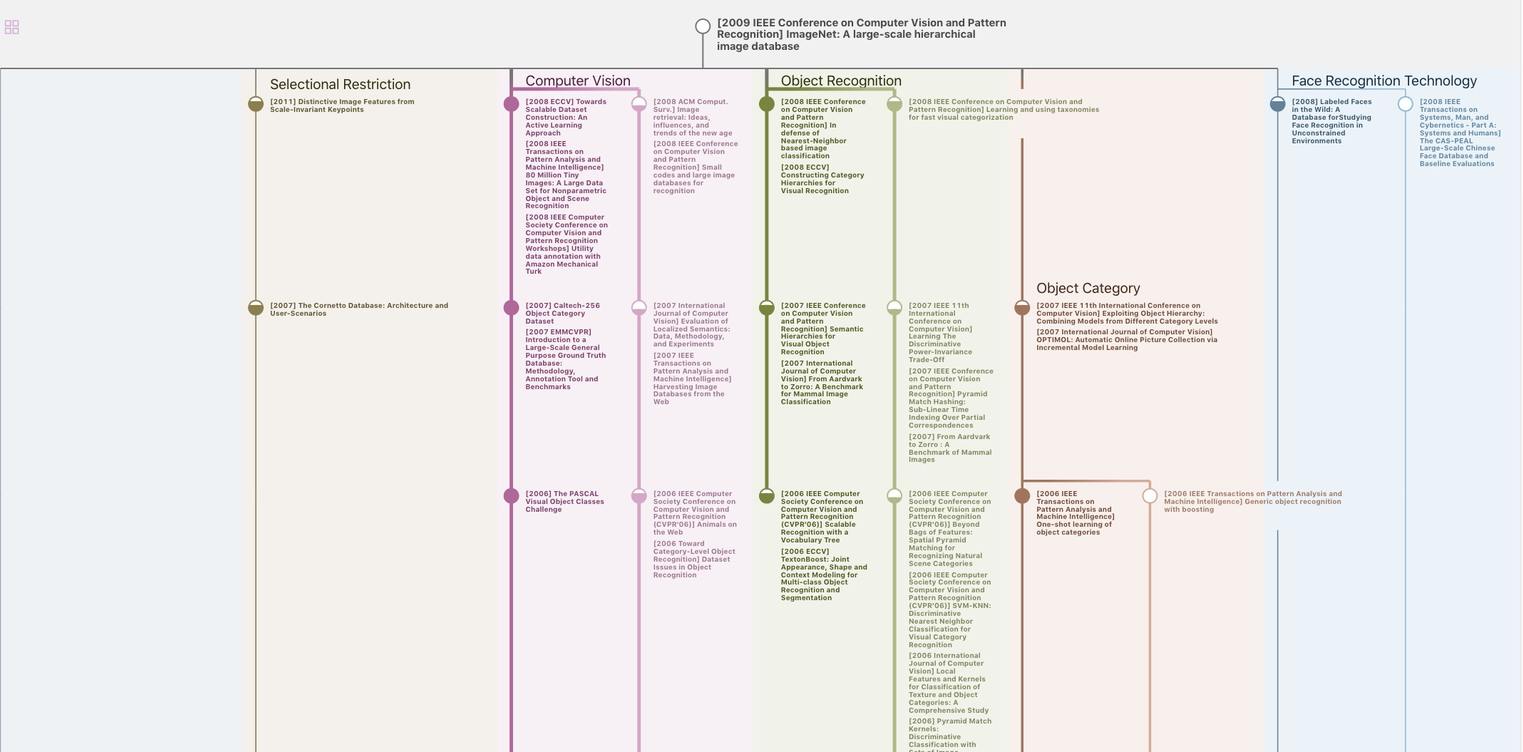
生成溯源树,研究论文发展脉络
Chat Paper
正在生成论文摘要