MEFuse: end-to-end infrared and visible image fusion method based on multibranch encoder
JOURNAL OF ELECTRONIC IMAGING(2022)
摘要
In recent years, deep learning-based image fusion methods have achieved promising fusion performance. However, existing fusion approaches always focus on increasing the network depth to enhance the feature extraction capacity. In addition, fusion is performed in a fixed feature space, which further degrades the performance. To this end, we proposed an end-to-end infrared and visible image fusion method based on a multibranch encoder, coined MEFuse. In principle, MEFuse increased the number of branches of the encoder, thus mapping the source images into different feature spaces, increasing the diversity of the extracted feature maps, and enhancing the learning capacity of the network. In addition, a multistage fusion strategy was designed to achieve multigranularity image fusion. First, the cascaded multimodal images were merged in the input stage; thus the primary visual fusion was realized in the coding process. Second, the feature maps from multiple coding branches were fused to fuse multimodal information comprehensively. Finally, the long-range lateral connection sent the input appearance of each encoding branch to the decoder to guide the image reconstruction process. Experiments on the benchmark data sets demonstrate that, compared with the existing methods, the proposed MEFuse achieved superior performance in both human perception and objective evaluation. (C) 2022 SPIE and IS&T
更多查看译文
关键词
image fusion, multibranch encoder, convolutional neural network
AI 理解论文
溯源树
样例
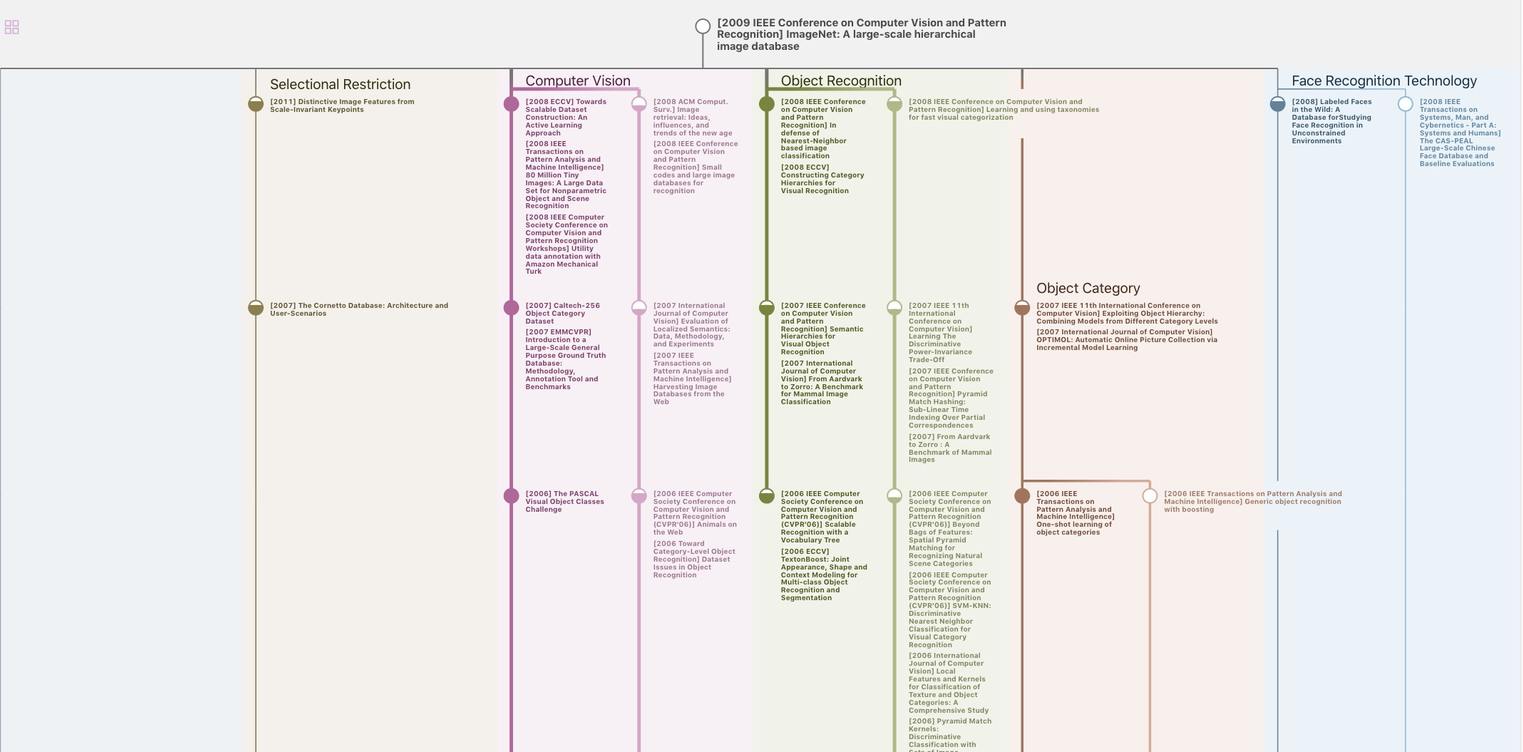
生成溯源树,研究论文发展脉络
Chat Paper
正在生成论文摘要