Genetic Algorithm-Optimized Extreme Learning Machine Model for Estimating Daily Reference Evapotranspiration in Southwest China
ATMOSPHERE(2022)
摘要
Reference evapotranspiration (ET0) is an essential component in hydrological and ecological processes. The Penman-Monteith (PM) model of Food and Agriculture Organization of the United Nations (FAO) model requires a number of meteorological parameters; it is urgent to develop high-precision and computationally efficient ET0 models with fewer parameter inputs. This study proposed the genetic algorithm (GA) to optimize extreme learning machine (ELM), and evaluated the performances of ELM, GA-ELM, and empirical models for estimating daily ET0 in Southwest China. Daily meteorological data including maximum temperature (T-max), minimum temperature (T-min), wind speed (u(2)), relative humidity (RH), net radiation (R-n), and global solar radiation (R-s) during 1992-2016 from meteorological stations were used for model training and testing. The results from the FAO-56 Penman-Monteith formula were used as a control group. The results showed that GA-ELM models (with R-2 ranging 0.71-0.99, RMSE ranging 0.036-0.77 mm center dot d(-1)) outperformed the standalone ELM models (with R-2 ranging 0.716-0.99, RMSE ranging 0.08-0.77 mm center dot d(-1)) during training and testing, both of which were superior to empirical models (with R-2 ranging 0.36-0.91, RMSE ranging 0.69-2.64 mm center dot d(-1)). ET0 prediction accuracy varies with different input combination models. The machine learning models using T-max, T-min, u(2), RH, and R-n/R-s (GA-ELM5/GA-ELM4 and ELM5/ELM4) obtained the best ET0 estimates, with R-2 ranging 0.98-0.99, RMSE ranging 0.03-0.21 mm center dot d(-1), followed by models with T-max, T-min, and R-n/R-s (GA-ELM3/GA-ELM2 and ELM3/ELM2) as inputs. The machine learning models involved with R-n outperformed those with R-s when the quantity of input parameters was the same. Overall, GA-ELM5 (T-max, T-min, u(2), RH and R-n as inputs) outperformed the other models during training and testing, and was thus recommended for daily ET0 estimation. With the estimation accuracy, computational costs, and availability of input parameters accounted, GA-ELM2 (T-max, T-min, and R-s as inputs) was determined to be the most effective model for estimating daily ET0 with limited meteorological data in Southwest China.
更多查看译文
关键词
reference evapotranspiration, extreme learning machine, optimization algorithm, empirical model, solar radiation
AI 理解论文
溯源树
样例
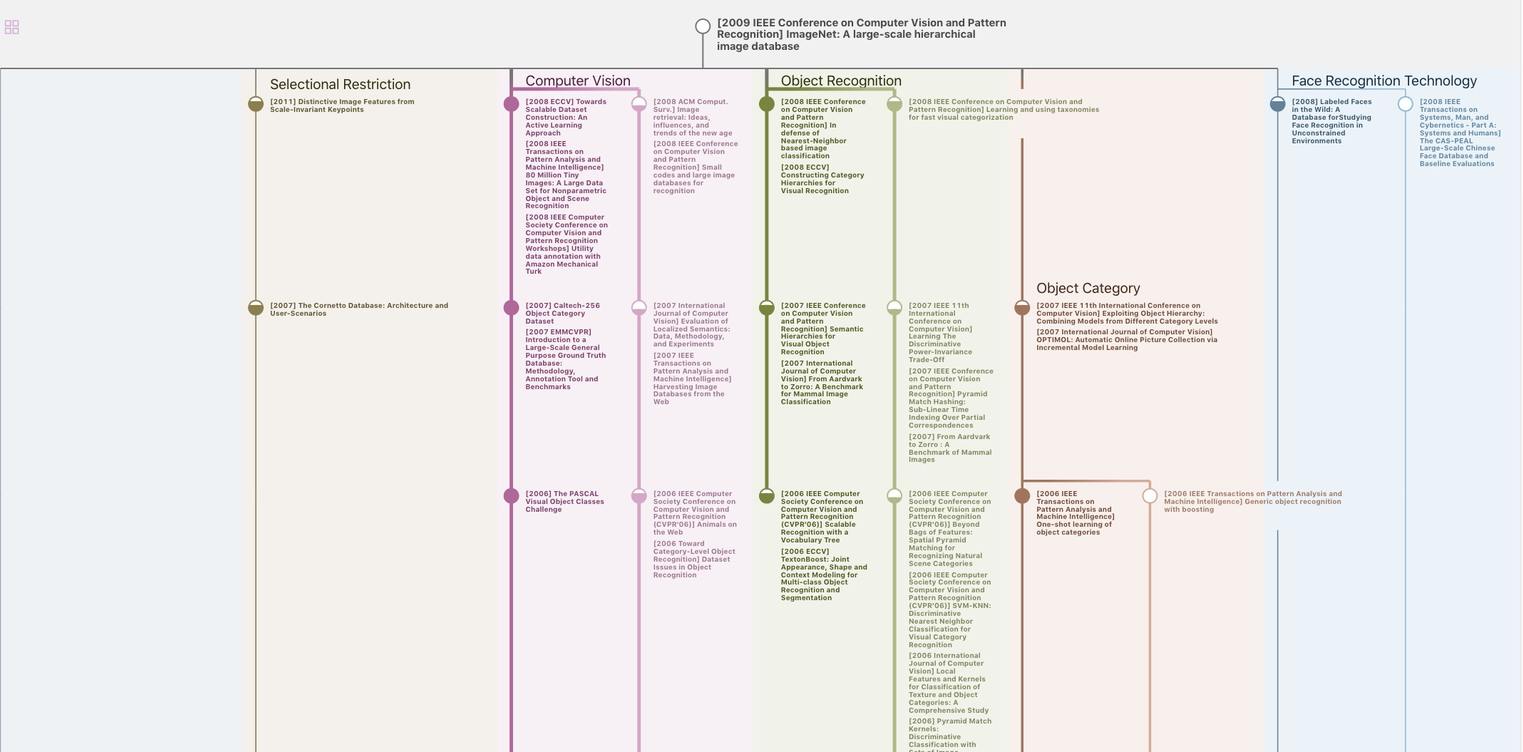
生成溯源树,研究论文发展脉络
Chat Paper
正在生成论文摘要