Semantic-Aware Privacy-Preserving Online Location Trajectory Data Sharing
IEEE TRANSACTIONS ON INFORMATION FORENSICS AND SECURITY(2022)
摘要
Although users can obtain various services by sharing their location information online with location-based service providers, it reveals sensitive information about users. However, existing privacy-preserving techniques in the online scenario suffer from the following shortcomings. First, they model the correlations between the real trajectory and the distorted trajectory as undirected, which makes them unable to accurately quantify the data privacy leakage caused by sharing the distorted trajectory. Second, they are unable to protect semantic privacy, i.e., attackers can obtain the victims' visit purpose by using the Point of Interest information without knowing the real location data. Additionally, they fail to balance semantic-aware data utility and privacy protection. To make the case even worse, compared to the offline scenario, sharing trajectory online in real time does not have access to the overall location trajectory. In this paper, we propose a novel semantic-aware privacy-preserving online location trajectory sharing mechanism, called SEmantic-aware Information-Theoretic Privacy (SEITP), to protect both data privacy and semantic privacy while the semantic-aware data utility can be preserved. In particular, we put forward two new metrics of privacy to capture data privacy leakage and semantic privacy leakage, respectively. Besides, to quantify the semantic-aware trajectory data utility, we propose a semantic-aware utility metric. With those metrics, the shortcoming of failing to guarantee the data utility is avoided naturally through structuring a multi-objective optimization problem. Then, we theoretically prove that the new construction can protect both data and semantic privacy. Finally, the experimental evaluations based on the real-world private vehicle trajectory dataset demonstrate that SEITP outperforms existing mechanisms.
更多查看译文
关键词
Data privacy,Trajectory,Privacy,Semantics,Measurement,Correlation,Optimization,Information-theoretic privacy,semantic privacy,location trajectory privacy,spatio-temporal dataset
AI 理解论文
溯源树
样例
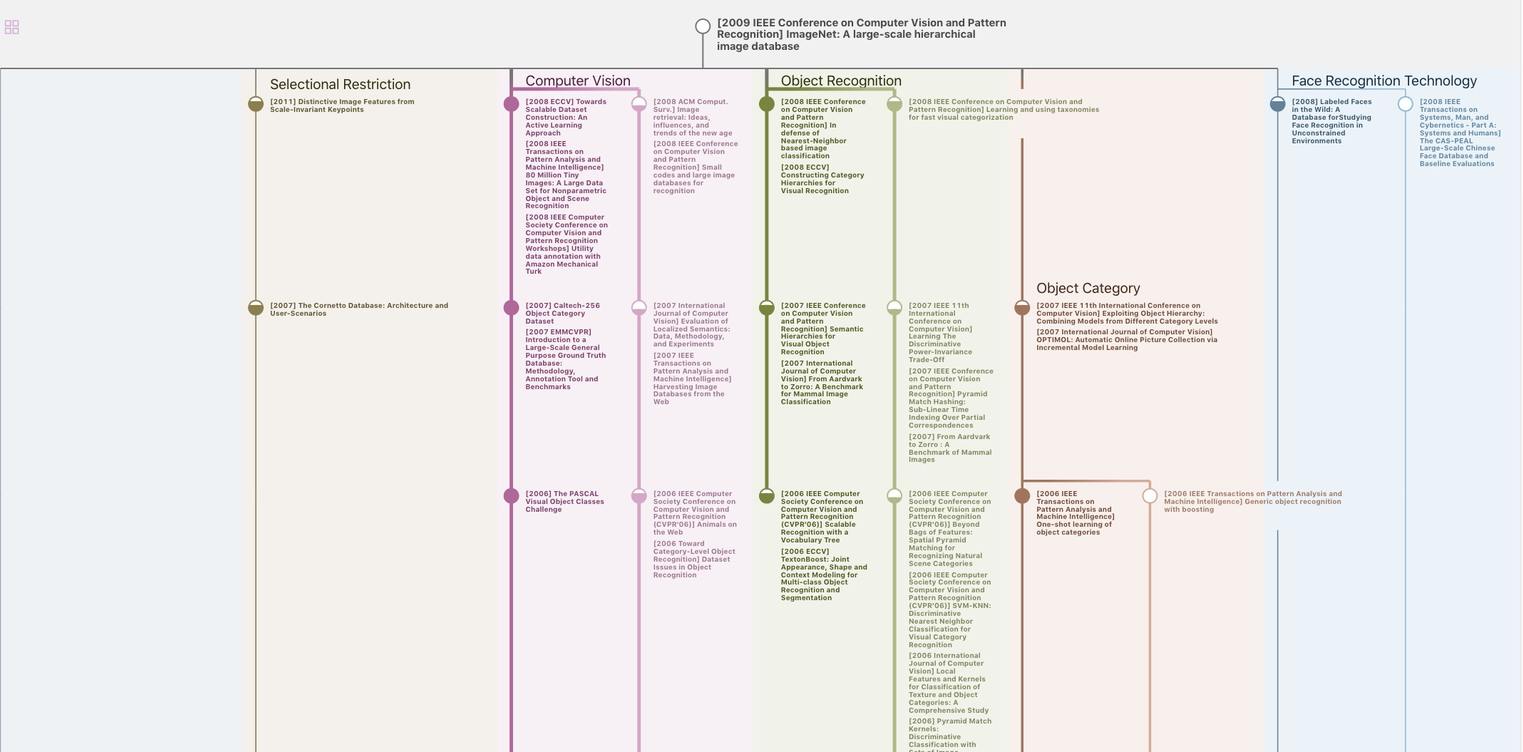
生成溯源树,研究论文发展脉络
Chat Paper
正在生成论文摘要