Analysis of Incremental Learning and Windowing to Handle Combined Dataset Shifts on Binary Classification for Product Failure Prediction.
International Conference on Enterprise Information Systems(2022)
摘要
: Dataset Shifts (DSS) are known to cause poor predictive performance in supervised machine learning tasks. We present a challenging binary classification task for a real-world use case of product failure prediction. The target is to predict whether a product, e.g., a truck may fail during the warranty period. However, building a satisfactory classifier is difficult, because the characteristics of underlying training data entail two kinds of DSS. First, the distribution of product configurations may change over time, leading to a covariate shift . Second, products gradually fail at different points in time, so that the labels in training data may change, which may a concept shift . Further, both DSS show a trade-off relationship, i.e., addressing one of them may imply negative impacts on the other one. We discuss the results of an experimental study to investigate how different approaches to addressing DSS perform when they are faced with both a covariate and a concept shift. Thereby, we prove that existing approaches, e.g., incremental learning and windowing , especially suffer from the trade-off between both DSS. Nevertheless, we come up with a solution for a data-driven classifier, that yields better results than a baseline solution that does not address DSS.
更多查看译文
关键词
Binary Classification,Combined Dataset Shift,Incremental Learning,Product Failure Prediction,Windowing
AI 理解论文
溯源树
样例
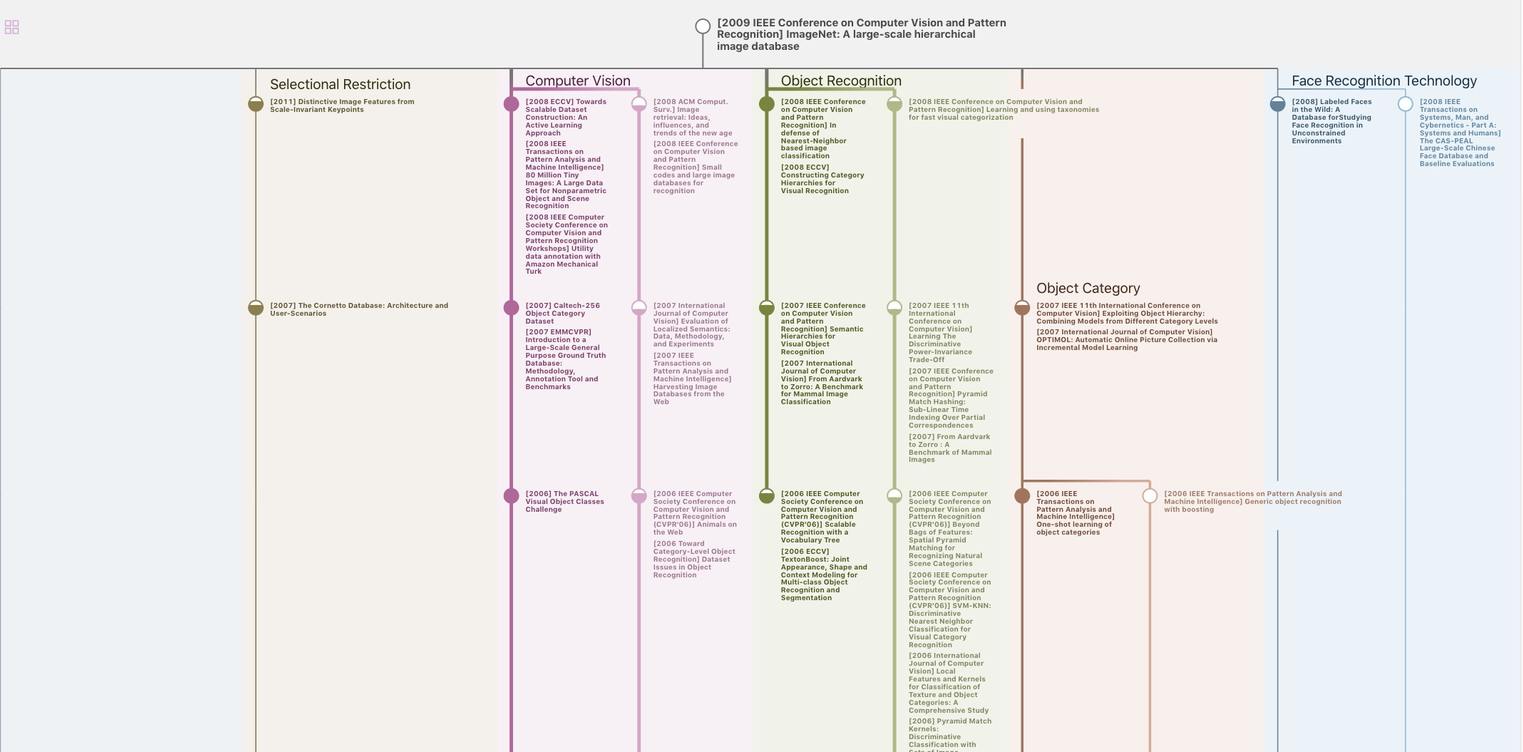
生成溯源树,研究论文发展脉络
Chat Paper
正在生成论文摘要