State-of-health estimation of lithium-ion batteries based on improved long short-term memory algorithm
Journal of Energy Storage(2022)
摘要
Long short-term memory network (LSTM) is a popular deep learning network method for estimating the state of health (SOH) of lithium-ion batteries. However, the hyperparameters in the network are usually difficult to pre-define, which degrades the estimation accuracy in applications. To address this challenge, this paper proposes a data-driven estimation method based on the improved LSTM, where the network topology is estimated by the particle swarm optimization (PSO) algorithm. First, four health indicators in the charging and discharging process are selected. Grey relational analysis is further employed to quantify their correlations with the battery SOH. Then, an LSTM model is established to map the relationship between health factors and battery SOH. To tackle the parameter determination and slow convergence problems of the classical LSTM method, the particle swarm optimization algorithm is adopted to determine the key hyperparameters in the neural network, and the RMSProp training method and dropout technique are introduced to accelerate the convergence speed and avoid over-fitting problems. The experimental results show that the prediction accuracy is improved by at least 5 % when compared with the classical LSTM method.
更多查看译文
关键词
Lithium-ion battery,State of health,Long short-term memory network,Particle swarm optimization,Grey relational analysis
AI 理解论文
溯源树
样例
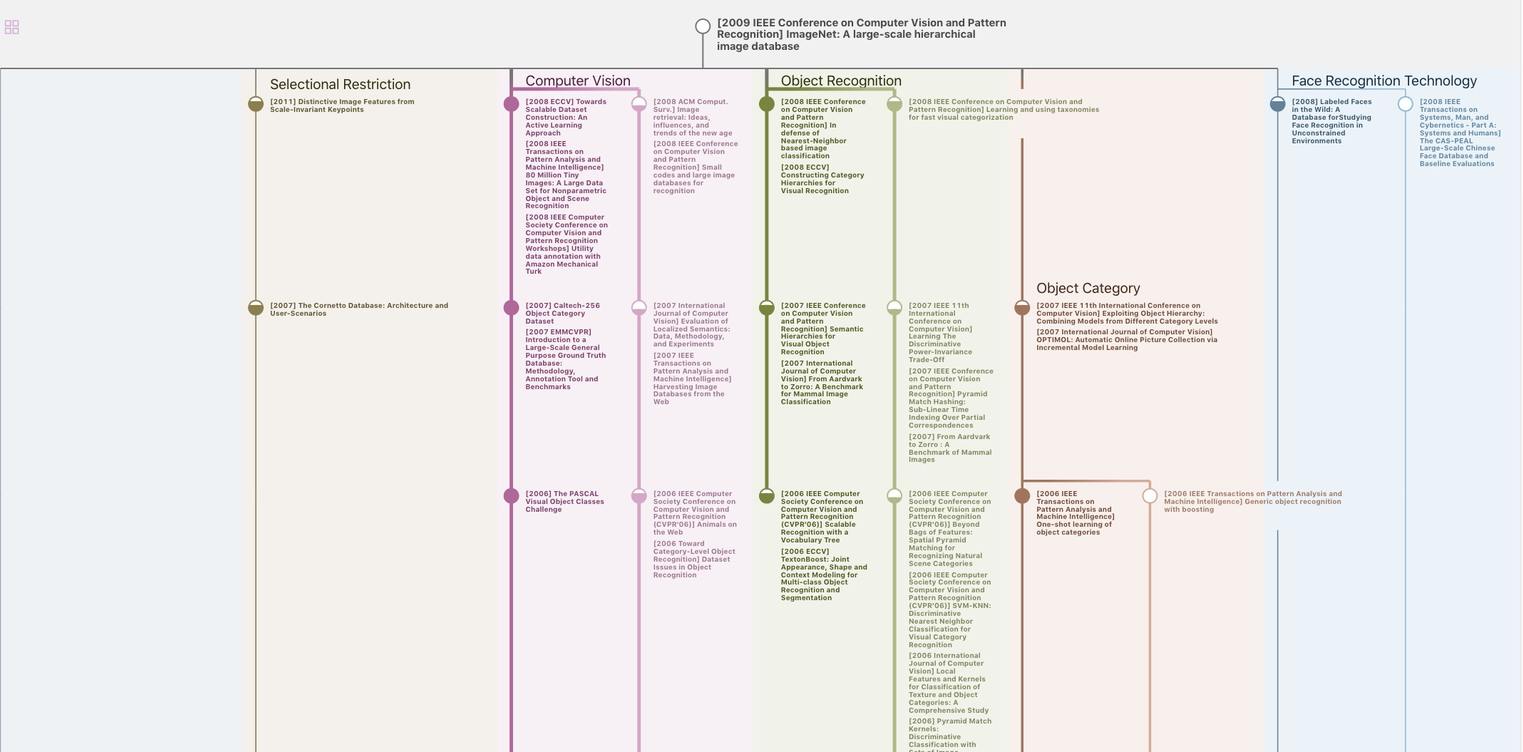
生成溯源树,研究论文发展脉络
Chat Paper
正在生成论文摘要