Fast Independent Component Analysis Denoising for Magnetotelluric Data Based on a Correlation Coefficient and Fast Iterative Shrinkage Threshold Algorithm
IEEE transactions on geoscience and remote sensing(2022)
摘要
Magnetotelluric (MT) sounding data are easily contaminated by various noise sources, especially noise with a long duration (even full-time noise), which makes it difficult to obtain accurate values when calculating the weight factors of response function, resulting in distortion of the response results. Based on blind source separation theory, fast independent component analysis (FastICA) can separate this kind of noise. However, this method is challenged by the number of separated field sources and the unequal signal amplitude before and after processing. We have developed a novel signal noise separation method, improving the traditional FastICA, where a correlation coefficient is used to compute the number of field sources, and the fast iterative shrinkage threshold algorithm (FISTA) is used to adjust the signal amplitude problem before and after FastICA decomposition. Compared with common field source division methods and the traditional FastICA, the experimental results indicate that our method can separate and remove noise with a long duration, increase the signal-to-noise ratio (SNR) of the data, and improve the MT response curves. Meanwhile, case studies of measured data illustrate that our method obtains a more robust MT response than the conventional robust method and FastICA.
更多查看译文
关键词
Entropy,Independent component analysis,Noise reduction,Wavelet transforms,Wavelet analysis,Iterative algorithms,Wavelet domain,Blind source separation technology,correlation coefficient,fast independent component analysis (FastICA),fast iterative shrinkage threshold algorithm (FISTA),magnetotelluric (MT)
AI 理解论文
溯源树
样例
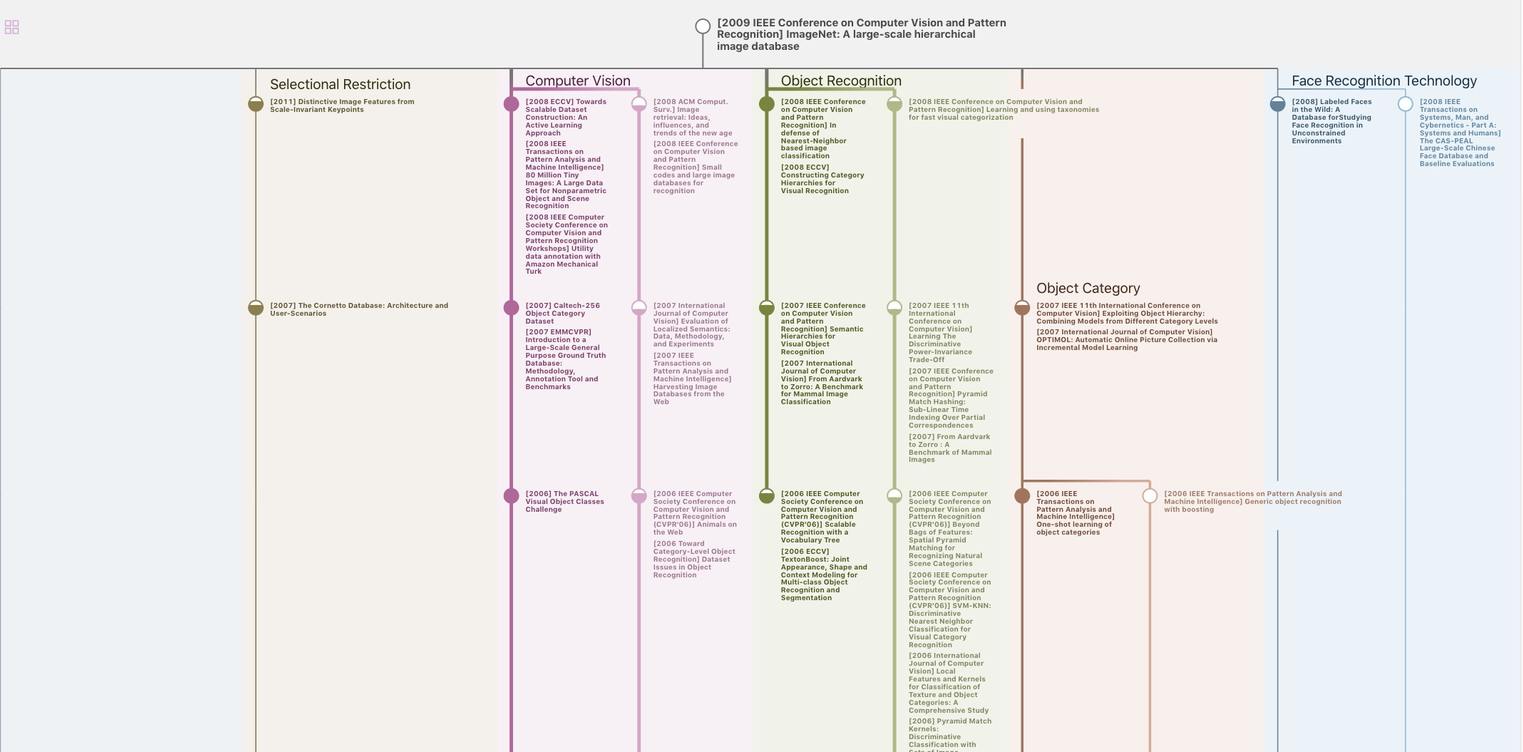
生成溯源树,研究论文发展脉络
Chat Paper
正在生成论文摘要