Evolving action pre-selection parameters for MCTS in real-time strategy games
ENTERTAINMENT COMPUTING(2022)
摘要
Real-Time Strategy (RTS) games are well-known for their substantially large combinatorial decision and state spaces, responsible for creating significant challenges for search and machine learning techniques. Exploiting domain knowledge to assist in navigating the expansive decision and state spaces could facilitate the emergence of competitive RTS game-playing agents. Usually, domain knowledge can take the form of expert traces or expert-authored scripts. A script encodes a strategy conceived by a human expert and can be used to steer a search algorithm, such as Monte Carlo Tree Search (MCTS), towards high-value states. However, a script is coarse by nature, meaning that it could be subject to exploitation and poor low-level tactical performance. We propose to perceive scripts as a collection of heuristics that can be parameterized and combined to form a wide array of strategies. The parameterized heuristics mold and filter the decision space in favor of a strategy expressed in terms of parameters. The proposed agent, ParaMCTS, implements several common heuristics and uses NaiveMCTS to search the downsized decision space; however, it requires a preceding manual parameterization step. A genetic algorithm is proposed for use in an optimization phase that aims to replace manual tuning and find an optimal set of parameters for use by EvoPMCTS, the evolutionary counterpart of ParaMCTS. Experimentation results using the mu RTS testbed show that EvoPMCTS outperforms several state-of-the-art agents across multiple maps of distinct layouts.
更多查看译文
关键词
Game AI, Real-Time Strategy, MCTS, mu RTS, Genetic Algorithms, Parameter Optimization
AI 理解论文
溯源树
样例
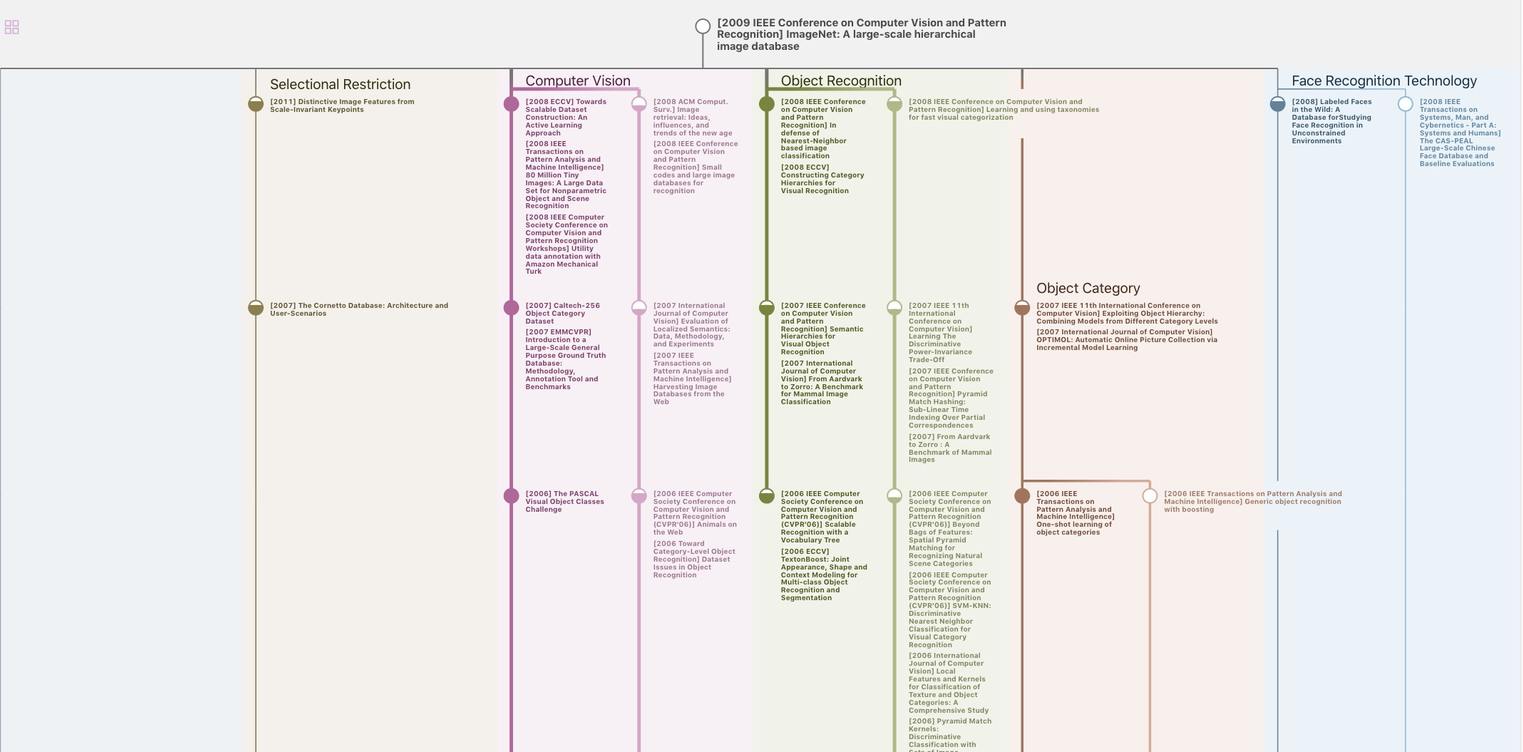
生成溯源树,研究论文发展脉络
Chat Paper
正在生成论文摘要