Gaussian Process-Assisted Active Learning for Autonomous Data Acquisition of Impact Echo
Automation in construction(2022)
摘要
The goal of this study is to pursue sampling efficiency and achieve reasonable accuracy for the quantity of interest (damage) in impact-echo testing. Because the locations of damages are not always obvious, the conventional grid-based measurement requires subjective judgment. The proposed solution is the implementation of Gaussian process (GP)-assisted active learning to enable the sequential sampling of the quantity of interest with autonomous decision-making for data acquisition. Throughout sampling, an automated model selection is employed to select a suitable kernel for GP modeling dynamically. Active learning is then applied to identify potential damage locations without any human assistance. The proposed method iterates the two modules to explore unknown damage and improve the accuracy of the quantity of interest until the maximum sample size is reached. Through numerical and experimental validation, this work demonstrates that the proposed method exhibits more accurate and efficient results.
更多查看译文
关键词
Autonomous data acquisition,Non-destructive testing,Gaussian process,Bayesian optimization,Automated model selection,Active learning,Impact-echo
AI 理解论文
溯源树
样例
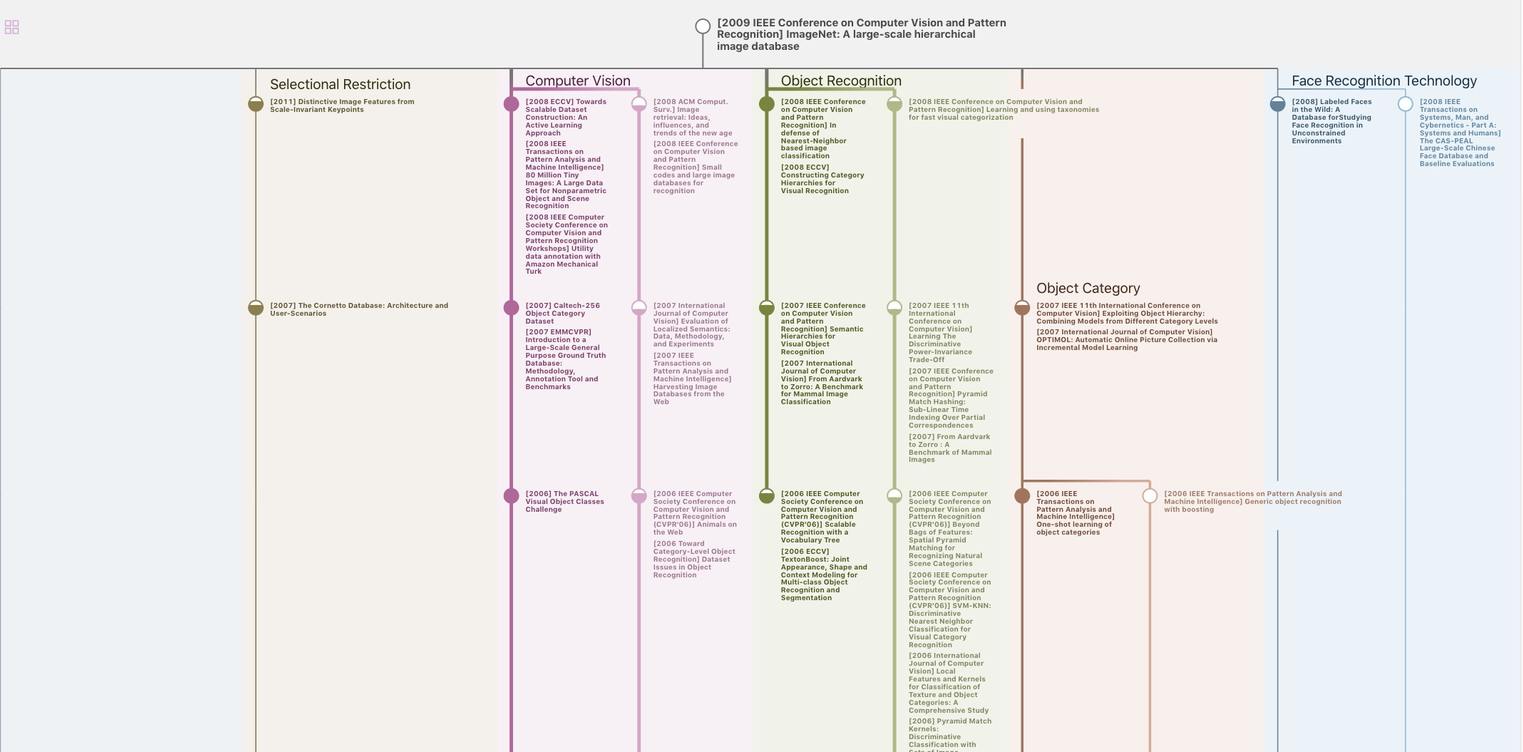
生成溯源树,研究论文发展脉络
Chat Paper
正在生成论文摘要