Load Forecasting of District Heating System Based on Informer
ENERGY(2022)
摘要
Accurate load forecasting of district heating systems (DHSs) is an essential guide to guaranteeing effective energy production, distribution, and rational utilization. Artificial neural networks have been extensively applied to heating energy prediction in DHS. Recently, a new time series prediction model namely Informer was proposed. This study proposes an Informer-based framework for DHS heating load forecasting. To explore the performance of Informer in heating load forecasting tasks, four forecasting models namely Autoregressive Integrated Moving Average model, Multilayer Perceptron, Recurrent Neural Network and Long Short-Term Memory network are established for comparison. The historical heating load, outdoor temperature, relative humidity, wind speed and air quality index of a DHS in Tianjin are used as the input characteristics to comprehensively assess the performance of these five forecasting strategies. The prediction results of the models are evaluated and visualized. The experi-mental results show that the Informer-based forecasting model can achieve the most accurate and stable predictions. Furthermore, a relative position encoding algorithm is introduced to enhance its general-ization and robustness. Overall, the Informer-based framework can report satisfactory testing results. The prediction curve is fitted to the trend of temperature change which can play an excellent guiding role in heating dispatching.(c) 2022 Elsevier Ltd. All rights reserved.
更多查看译文
关键词
District heating system,Heating load forecasting,Informer,Relative position encoding algorithm
AI 理解论文
溯源树
样例
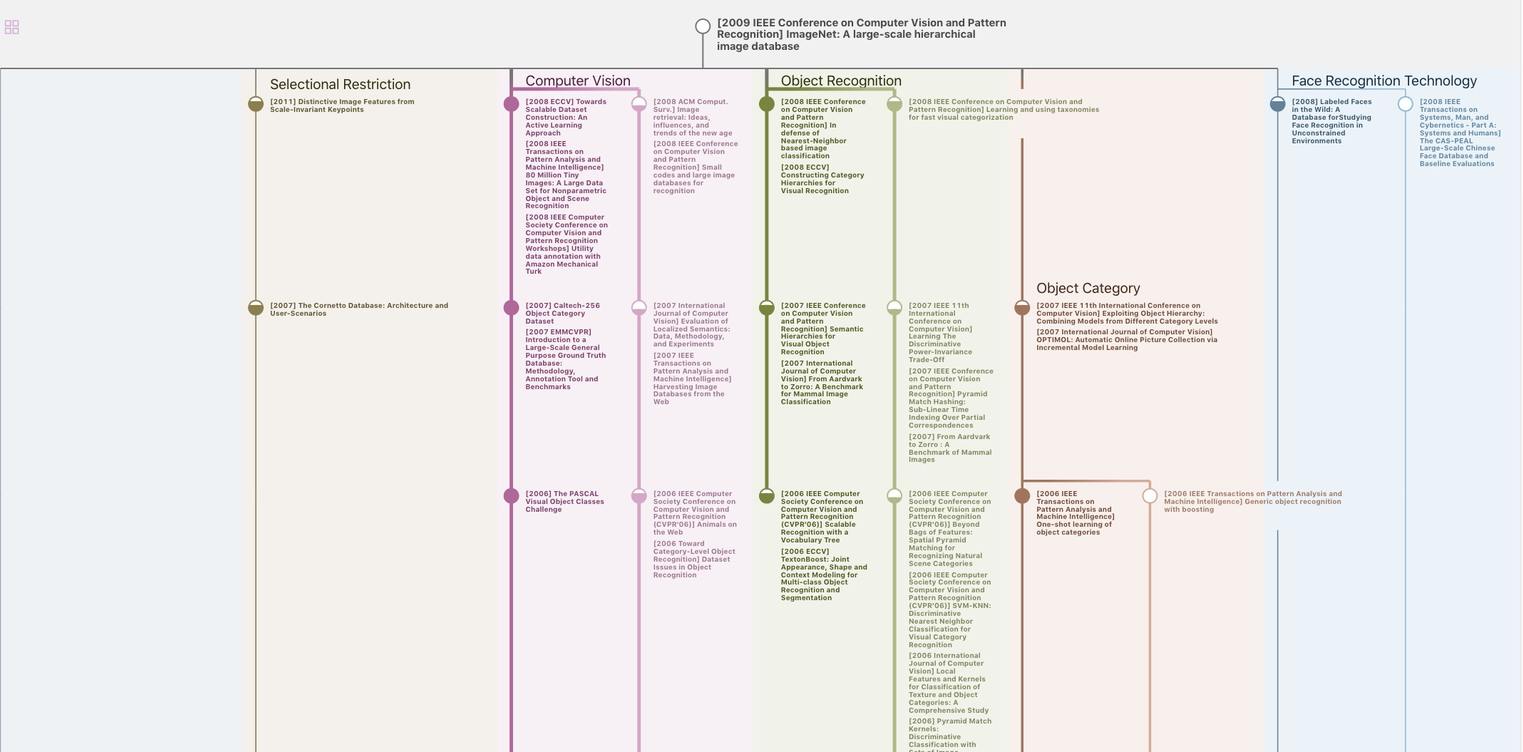
生成溯源树,研究论文发展脉络
Chat Paper
正在生成论文摘要