SigNet: A Novel Deep Learning Framework for Radio Signal Classification
IEEE Transactions on Cognitive Communications and Networking(2022)
摘要
Deep learning methods achieve great success in many areas due to their powerful feature extraction capabilities and end-to-end training mechanism, and recently they are also introduced for radio signal modulation classification. In this paper, we propose a novel deep learning framework called SigNet, where a signal-to-matrix (S2M) operator is adopted to convert the original signal into a square matrix first and is co-trained with a follow-up CNN architecture for classification. This model is further accelerated by integrating 1D convolution operators, leading to the upgraded model SigNet2.0. The simulations on two signal datasets show that both SigNet and SigNet2.0 outperform a number of well-known baselines. More interestingly, our proposed models behave extremely well in small-sample learning when only a small training dataset is provided. They can achieve a relatively high accuracy even when 1% training data are kept, while other baseline models may lose their effectiveness much more quickly as the datasets get smaller. Such result suggests that SigNet/SigNet2.0 could be extremely useful in the situations where labeled signal data are difficult to obtain. The visualization of the output features of our models demonstrates that our model can well divide different modulation types of signals in the feature hyper-space.
更多查看译文
关键词
Deep learning,modulation recognition,convolutional neural network
AI 理解论文
溯源树
样例
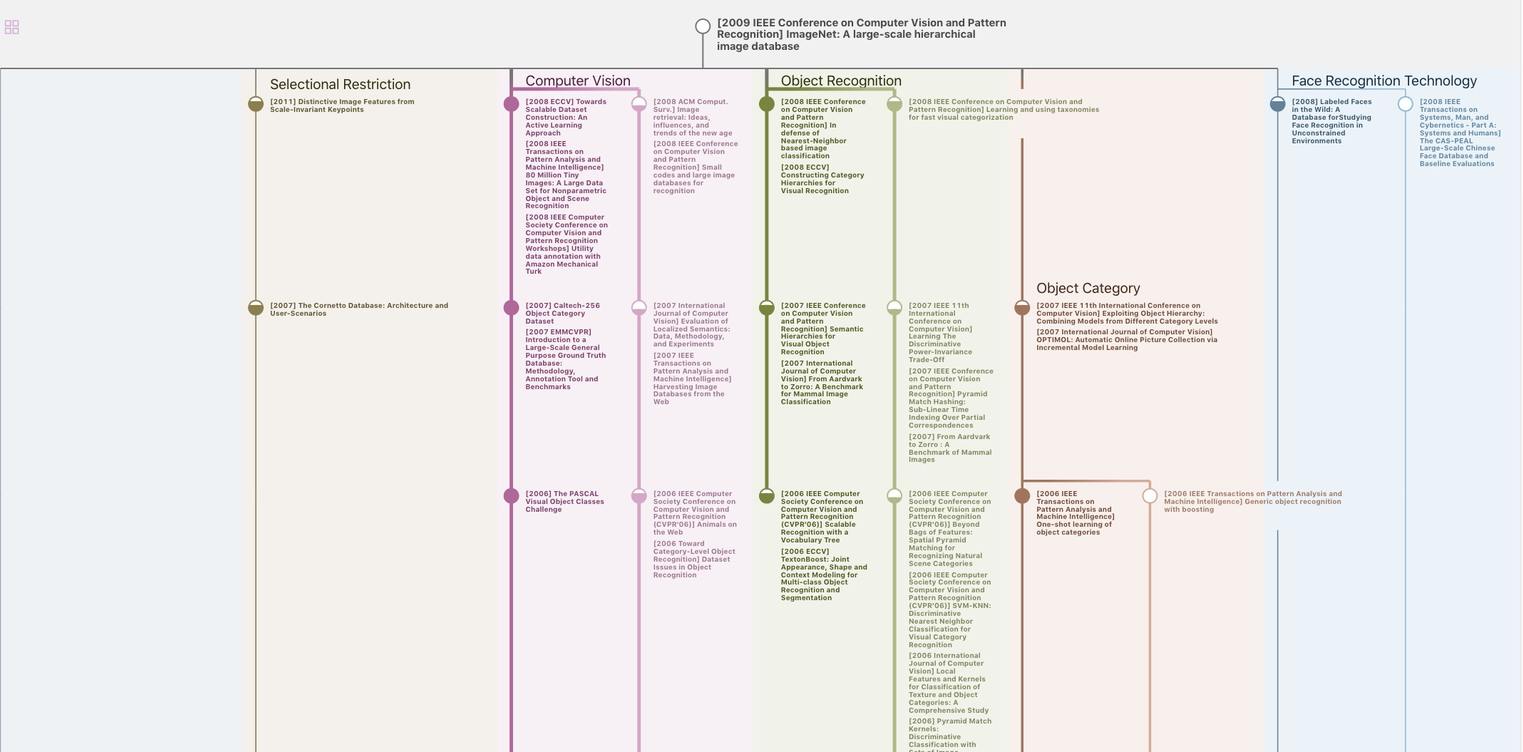
生成溯源树,研究论文发展脉络
Chat Paper
正在生成论文摘要