MFAGCN: A new framework for identifying power grid branch parameters
ELECTRIC POWER SYSTEMS RESEARCH(2022)
摘要
In the parameter identification of power transmission system, deep learning methods stand out because of its effectiveness and robustness. However, deep learning methods usually suffers from two limitations: (1) The power grid topology structure data belongs to non-Euclidean data, so the traditional deep learning methods can't deal with this data, and it is easy to introduce noise. (2) The order of magnitude difference of different identi-fication targets is too large, and it is easy to ignore small targets if they are identified at the same time, which makes the identification accuracy decline. To overcome these limitations, a novel multi-scale folded attention graph convolution network (MFAGCN) is proposed, which uses U-block to sample data from multiple scales in the power grid, and not only fuses the global nodes in the power grid system, but also avoids over-fitting caused by too deep layers of the graph convolution network. In addition, a new loss function is designed for the multi -task module NestedTask-Block, which can balance the parameters of large targets and small targets, and realize multi-branch and multi-parameter simultaneous identification. The experimental results show that MFAGCN can achieve excellent performance through compared with the most advanced identification method.
更多查看译文
关键词
Parameter identification, Multi-level information, Gated attention network, Deep learning, Graph neural network
AI 理解论文
溯源树
样例
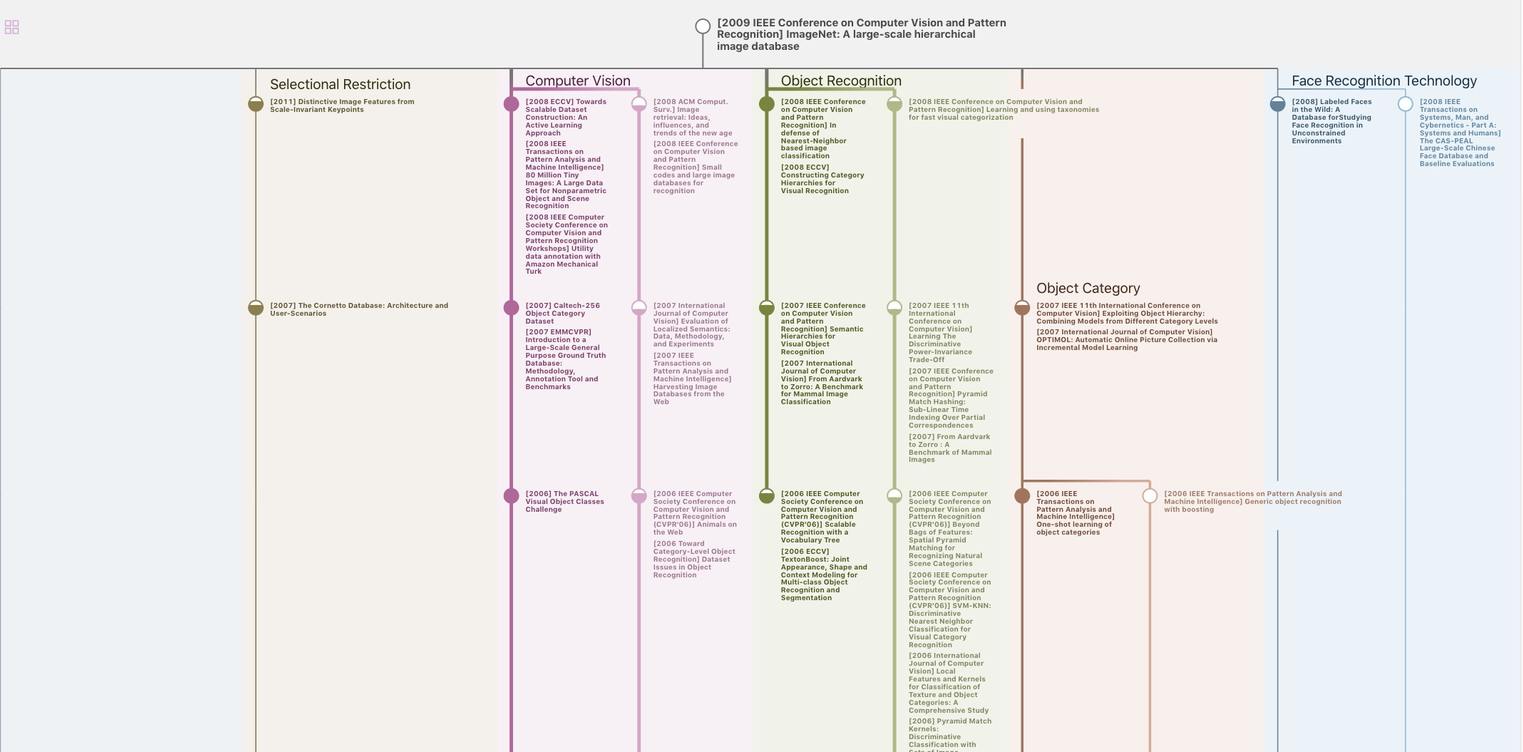
生成溯源树,研究论文发展脉络
Chat Paper
正在生成论文摘要