Artificial neural networks for material parameter extraction in terahertz time-domain spectroscopy
OPTICS EXPRESS(2022)
摘要
Terahertz time-domain spectroscopy (THz-TDS) is a proven technique whereby the complex refractive indices of materials can be obtained without requiring the use of the Kramers-Kronig relations, as phase and amplitude information can be extracted from the measurement. However, manual pre-processing of the data is still required and the material parameters require iterative fitting, resulting in complexity, loss of accuracy and inconsistencies between measurements. Alternatively approximations can be used to enable analytical extraction but with a considerable sacrifice of accuracy. We investigate the use of machine learning techniques for interpreting spectroscopic THz-TDS data by training with large data sets of simulated light-matter interactions, resulting in a computationally efficient artificial neural network for material parameter extraction. The trained model improves on the accuracy of analytical methods that need approximations while being easier to implement and faster to run than iterative root-finding methods. We envisage neural networks can alleviate many of the common hurdles involved in analyzing THz-TDS data such as phase unwrapping, time domain windowing, slow computation times, and extraction accuracy at the low frequency range. Published by Optica Publishing Group under the terms of the Creative Commons Attribution 4.0 License.
更多查看译文
关键词
material parameter extraction,spectroscopy,neural networks,time-domain
AI 理解论文
溯源树
样例
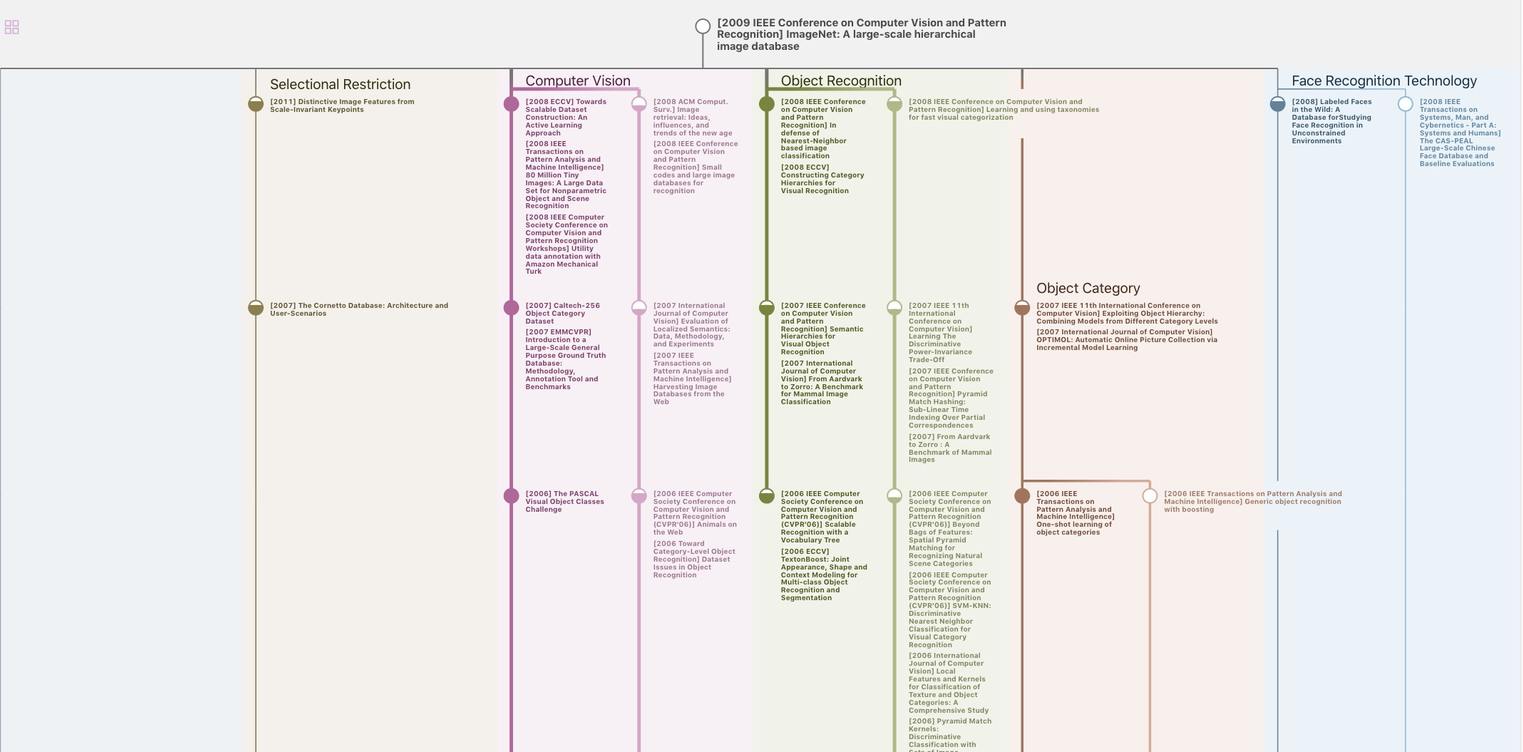
生成溯源树,研究论文发展脉络
Chat Paper
正在生成论文摘要