An Inhomogeneous Grid-Based Evolutionary Algorithm for Many-Objective Optimization
IEEE ACCESS(2022)
摘要
Today, many-objective optimization problems have attracted widespread attention. There are significant advantages of the grid-based algorithm in solving multi-objective problems. Grid-based algorithm could offer a transformation of objectives and further distinguish the non-dominated solutions. However, the advantages of grid have not been fully exploited. For example, the traditional homogeneous grid divisions can't sufficiently reveal the similarity of adjacent solutions. And overemphasizing the selection pressure may cause the diversity decline of grid. To exploit the potentialities of grid, an inhomogeneous grid-based evolutionary algorithm (named IGEA) is proposed. IGEA applies a dynamic inhomogeneous grid division approach and redefining the coordinate assignment of individuals, which makes the dominance relationship more obvious. IGEA also applied the shift-based density estimation (SDE) strategy in discriminating the non-dominated solutions in grid coordinate. SDE can provide a good balance of convergence and diversity. The IGEA compares with several state-of-the-art evolutionary algorithms against the regular and irregular many-objective optimization problems. The experimental results demonstrate that IGEA is very competitive against the peer algorithms in terms of providing a good balance between convergence and diversity.
更多查看译文
关键词
Convergence, Optimization, Nonhomogeneous media, Evolutionary computation, Sociology, Measurement, Estimation, Many-objective optimization, grid-based, inhomogeneous grid, shift-based density estimation
AI 理解论文
溯源树
样例
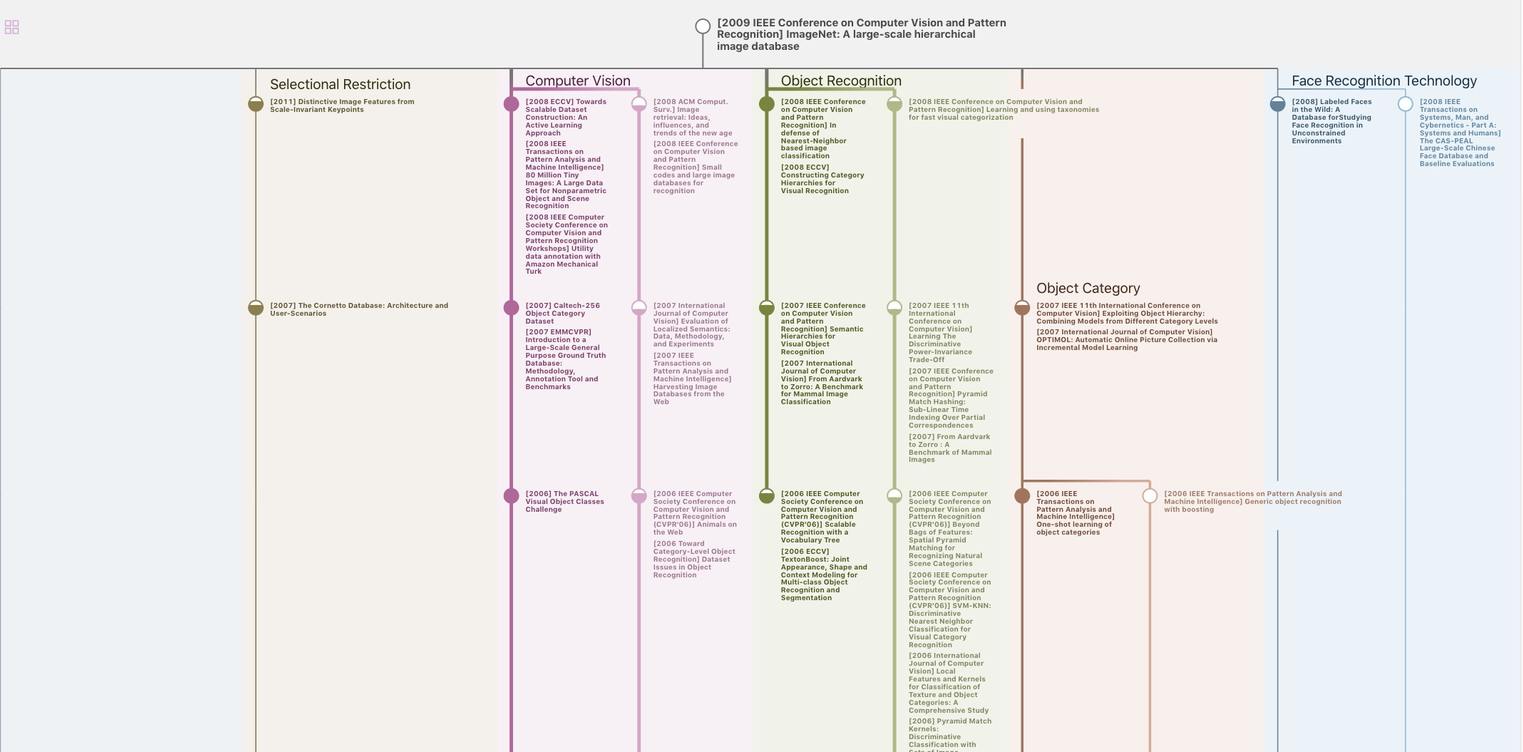
生成溯源树,研究论文发展脉络
Chat Paper
正在生成论文摘要