Sampling diversity driven exploration with state difference guidance
Expert Systems with Applications(2022)
摘要
Exploration is one of the key issues of deep reinforcement learning, especially in the environments with sparse or deceptive rewards. Exploration based on intrinsic rewards can handle these environments. However, these methods cannot take both global interaction dynamics and local environment changes into account simultaneously. In this paper, we propose a novel intrinsic reward for off-policy learning, which not only encourages the agent to take actions not fully learned from a global perspective, but also instructs the agent to trigger remarkable changes in the environment from a local perspective. Meanwhile, we propose the double-actors–double-critics framework to combine intrinsic rewards with extrinsic rewards to avoid the inappropriate combination of intrinsic and extrinsic rewards in previous methods. This framework can be applied to off-policy learning algorithms based on the actor–critic method. We provide a comprehensive evaluation of our approach on the MuJoCo benchmark environments. The results demonstrate that our method can perform effective exploration in the environments with dense, deceptive and sparse rewards. Besides, we conduct sufficient ablation and quantitative analyses to intrinsic rewards. Furthermore, we also verify the superiority and rationality of our double-actors–double-critics framework through comparative experiments.
更多查看译文
关键词
Reinforcement learning,Exploration,Intrinsic rewards,Off-policy,Actor–critic algorithm
AI 理解论文
溯源树
样例
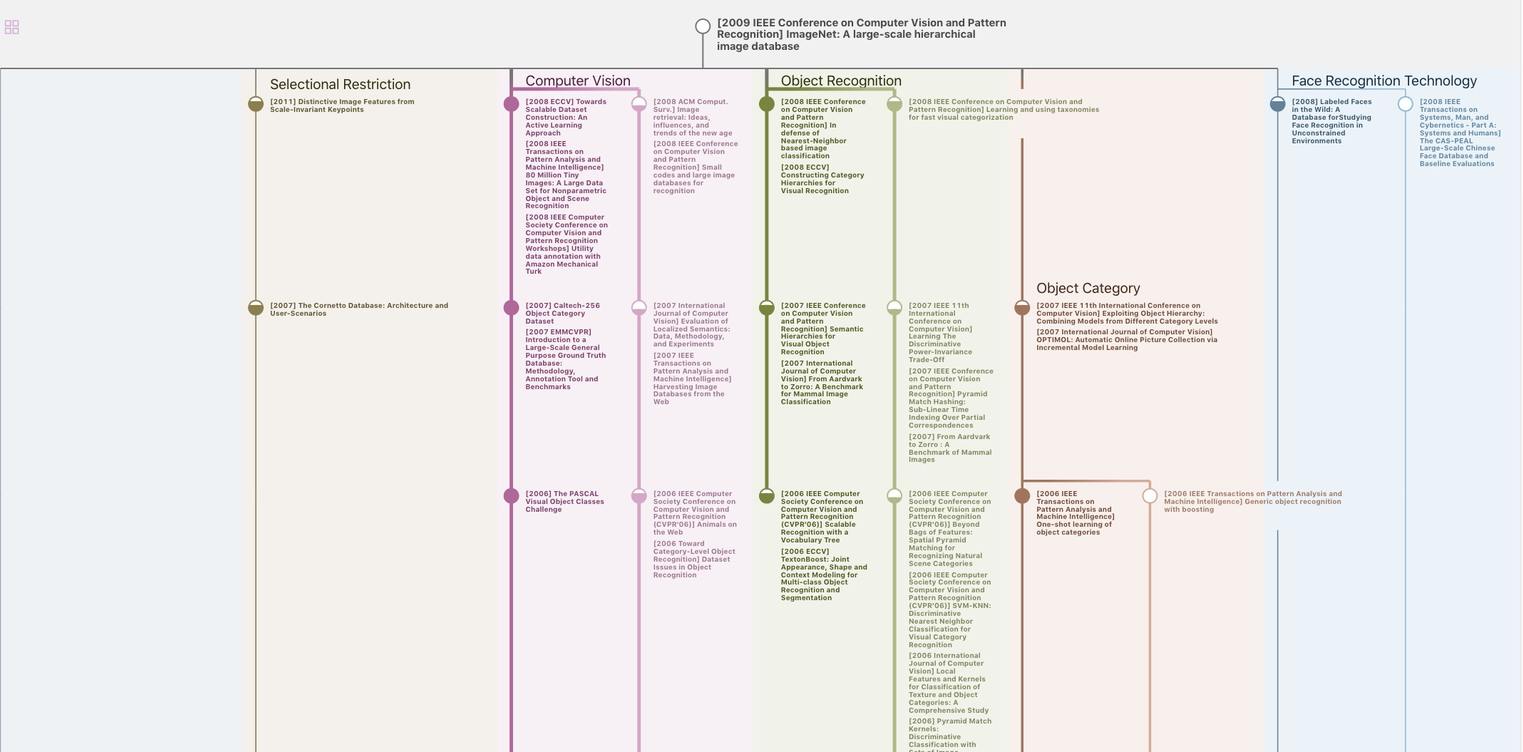
生成溯源树,研究论文发展脉络
Chat Paper
正在生成论文摘要