Deep Learning-Based Approach for the Automatic Quantification of Epicardial Adipose Tissue from Non-Contrast CT
Cognitive Computation(2022)
摘要
Epicardial adipose tissue (EAT) is contiguous with arteries and myocardium. An increase in the volume of EAT may lead to adverse cardiovascular events. Therefore, quantification of EAT is necessary. The purpose of this paper is to employ a more than helpful algorithm for EAT segmentation and quantification. First, we used a simple convolutional neural network to select EAT slices, which significantly reduced oversegmentation. Then, we employed multiscale residual attention Unet (MRA-Unet) to achieve EAT segmentation based on the selected slices. Finally, we calculated the segmented volume to quantify EAT. We used 33/103 patients to test the model. The average Dice score for EAT segmentation was 0.883. For EAT quantification, the Pearson and concordance correlation coefficients reached 0.973 and 0.971, respectively. The results showed that our algorithm had strong agreement and consistency with expert. Our method performed efficient quantification and had strong consistency and agreement with the volume manually marked by experts. This algorithm can be used as a tool to assist in the clinical quantification of EAT. By combining different measurements to predict adverse cardiovascular and heart disease events, it has the potential to be applied for clinical use in the future.
更多查看译文
关键词
UNet,Non-contrast CT,Epicardial adipose tissue,Convolutional neural network,Segmentation and quantification
AI 理解论文
溯源树
样例
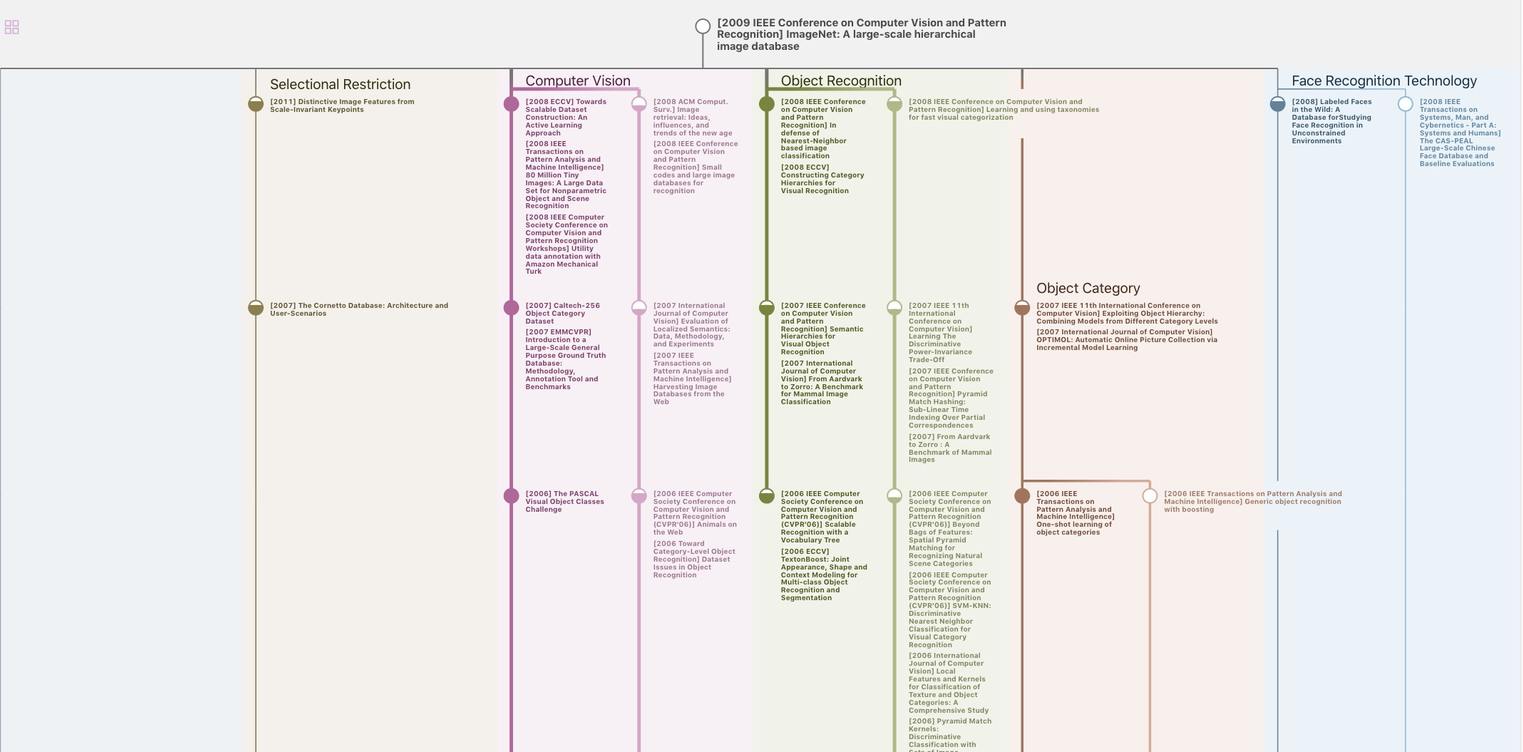
生成溯源树,研究论文发展脉络
Chat Paper
正在生成论文摘要