Efficient Hyperspectral Reconstruction from RGB Images with Line-Pixel Deconvolution
2022 IEEE 14th Image, Video, and Multidimensional Signal Processing Workshop (IVMSP)(2022)
摘要
This paper presents an efficient learning-based model to reconstruct hyperspectral cubes from RGB images. While several deep learning models have been developed for the hyperspectral reconstruction problem, the expensive computation associated with these models discounted their practicality to be implemented in end-user devices, e.g., the smartphone. To this end, we propose a Line-Pixel Deconvolution, mimicking the line scanning mechanism of a pushbroom camera, to first reconstruct the spectral information of each line, before composing the final hyperspectral cube with each reconstructed line. The resulting model achieves satisfactory reconstruction quality comparable to the existing deep learning models used in the hyperspectral reconstruction problem, while requiring lesser parameters and operations. Our proposed model also achieves a faster inference time compared to the existing deep learning models.
更多查看译文
关键词
Line-Pixel Deconvolution,line scanning mechanism,final hyperspectral cube,reconstructed line,satisfactory reconstruction quality,existing deep learning models,hyperspectral reconstruction problem,efficient hyperspectral reconstruction,RGB images,efficient learning-based model,hyperspectral cubes,end-user devices
AI 理解论文
溯源树
样例
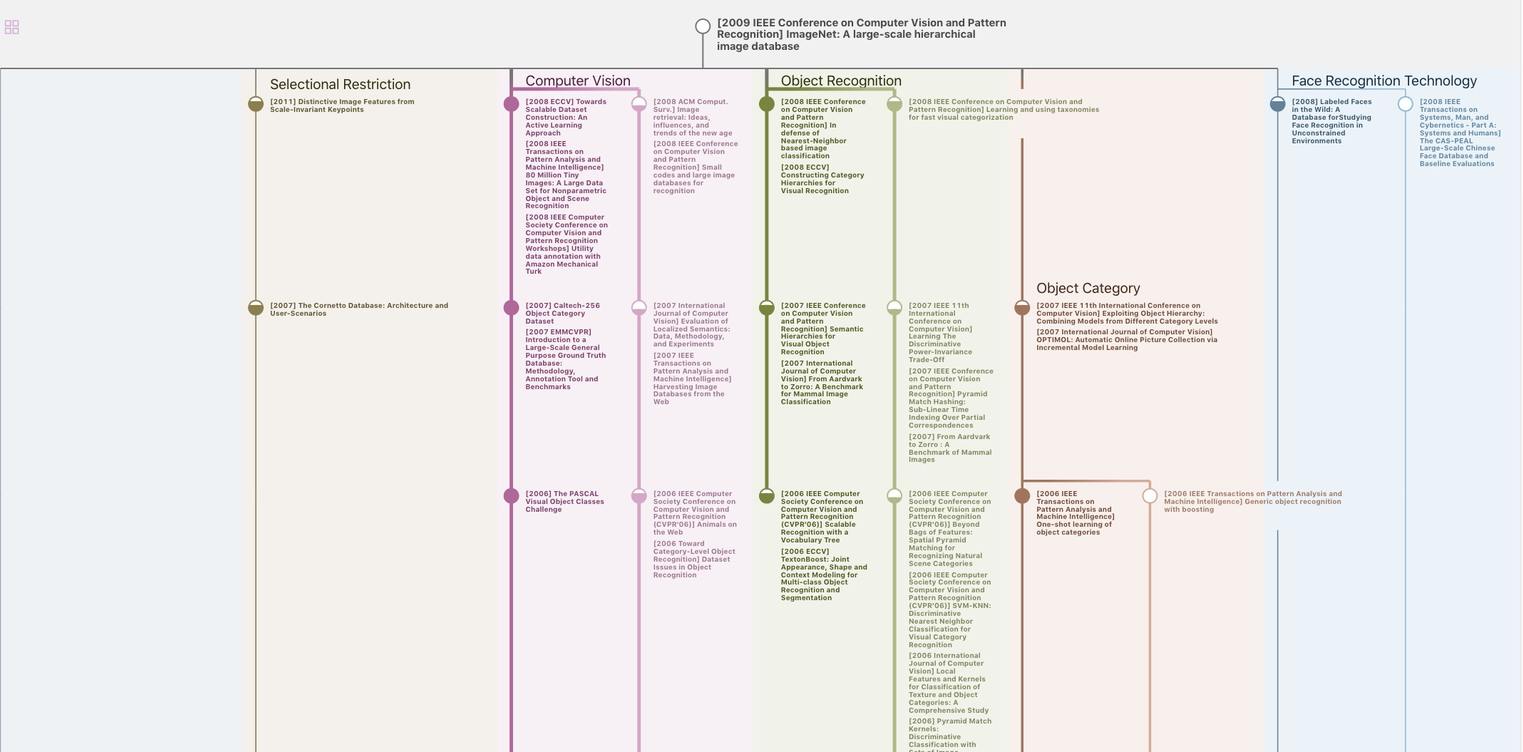
生成溯源树,研究论文发展脉络
Chat Paper
正在生成论文摘要