Case study on quantum convolutional neural network scalability
CoRR(2022)
摘要
One of the crucial tasks in computer science is the processing time reduction of various data types, i.e., images, which is important for different fields -- from medicine and logistics to virtual shopping. Compared to classical computers, quantum computers are capable of parallel data processing, which reduces the data processing time. This quality of quantum computers inspired intensive research of the potential of quantum technologies applicability to real-life tasks. Some progress has already revealed on a smaller volumes of the input data. In this research effort, I aimed to increase the amount of input data (I used images from 2 x 2 to 8 x 8), while reducing the processing time, by way of skipping intermediate measurement steps. The hypothesis was that, for increased input data, the omitting of intermediate measurement steps after each quantum convolution layer will improve output metric results and accelerate data processing. To test the hypothesis, I performed experiments to chose the best activation function and its derivative in each network. The hypothesis was partly confirmed in terms of output mean squared error (MSE) -- it dropped from 0.25 in the result of classical convolutional neural network (CNN) training to 0.23 in the result of quantum convolutional neural network (QCNN) training. In terms of the training time, however, which was 1.5 minutes for CNN and 4 hours 37 minutes in the least lengthy training iteration, the hypothesis was rejected.
更多查看译文
关键词
convolutional neural network scalability,quantum,neural network
AI 理解论文
溯源树
样例
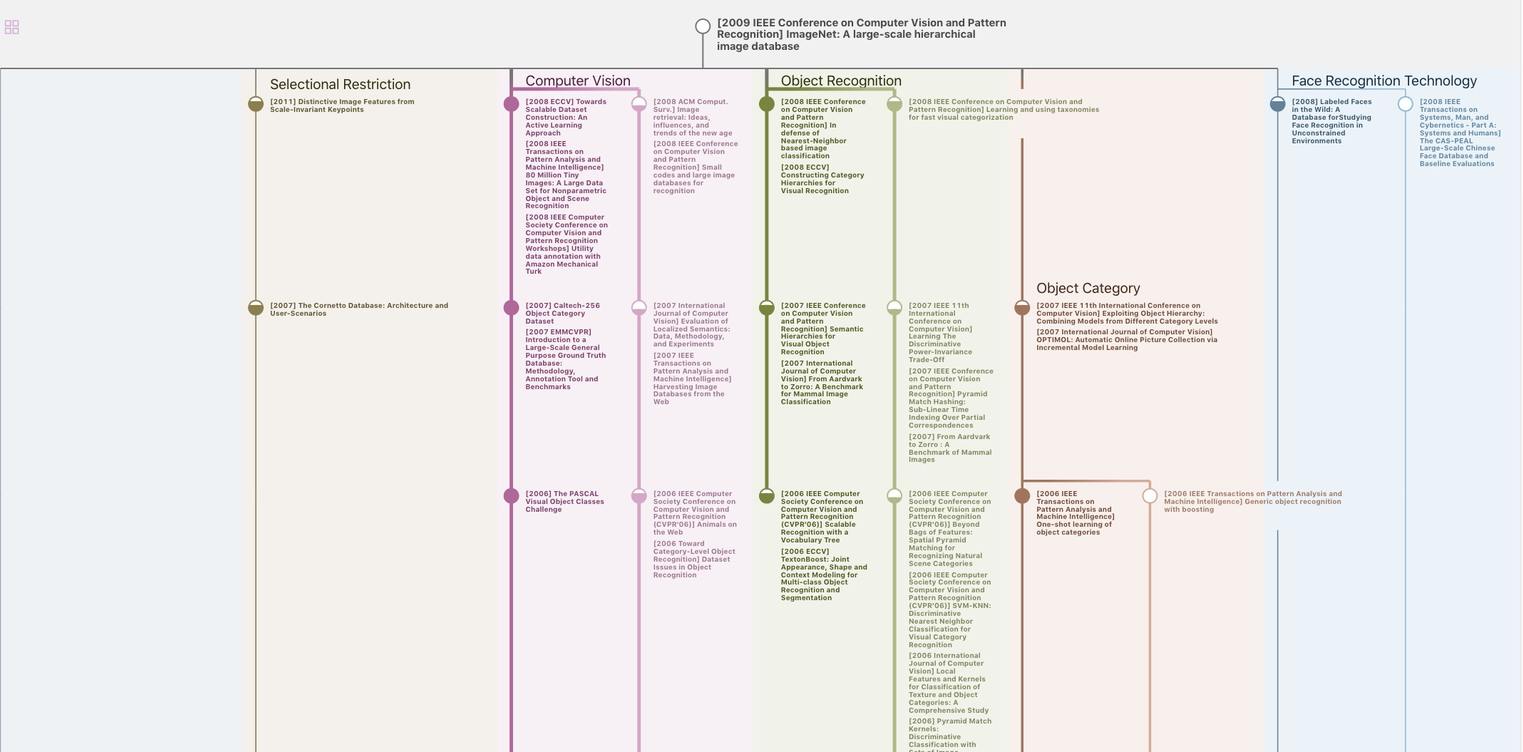
生成溯源树,研究论文发展脉络
Chat Paper
正在生成论文摘要