USegScene: Unsupervised Learning of Depth, Optical Flow and Ego-Motion with Semantic Guidance and Coupled Networks
arxiv(2022)
摘要
In this paper we propose USegScene, a framework for semantically guided unsupervised learning of depth, optical flow and ego-motion estimation for stereo camera images using convolutional neural networks. Our framework leverages semantic information for improved regularization of depth and optical flow maps, multimodal fusion and occlusion filling considering dynamic rigid object motions as independent SE(3) transformations. Furthermore, complementary to pure photo-metric matching, we propose matching of semantic features, pixel-wise classes and object instance borders between the consecutive images. In contrast to previous methods, we propose a network architecture that jointly predicts all outputs using shared encoders and allows passing information across the task-domains, e.g., the prediction of optical flow can benefit from the prediction of the depth. Furthermore, we explicitly learn the depth and optical flow occlusion maps inside the network, which are leveraged in order to improve the predictions in therespective regions. We present results on the popular KITTI dataset and show that our approach outperforms other methods by a large margin.
更多查看译文
关键词
optical flow,depth,learning,ego-motion
AI 理解论文
溯源树
样例
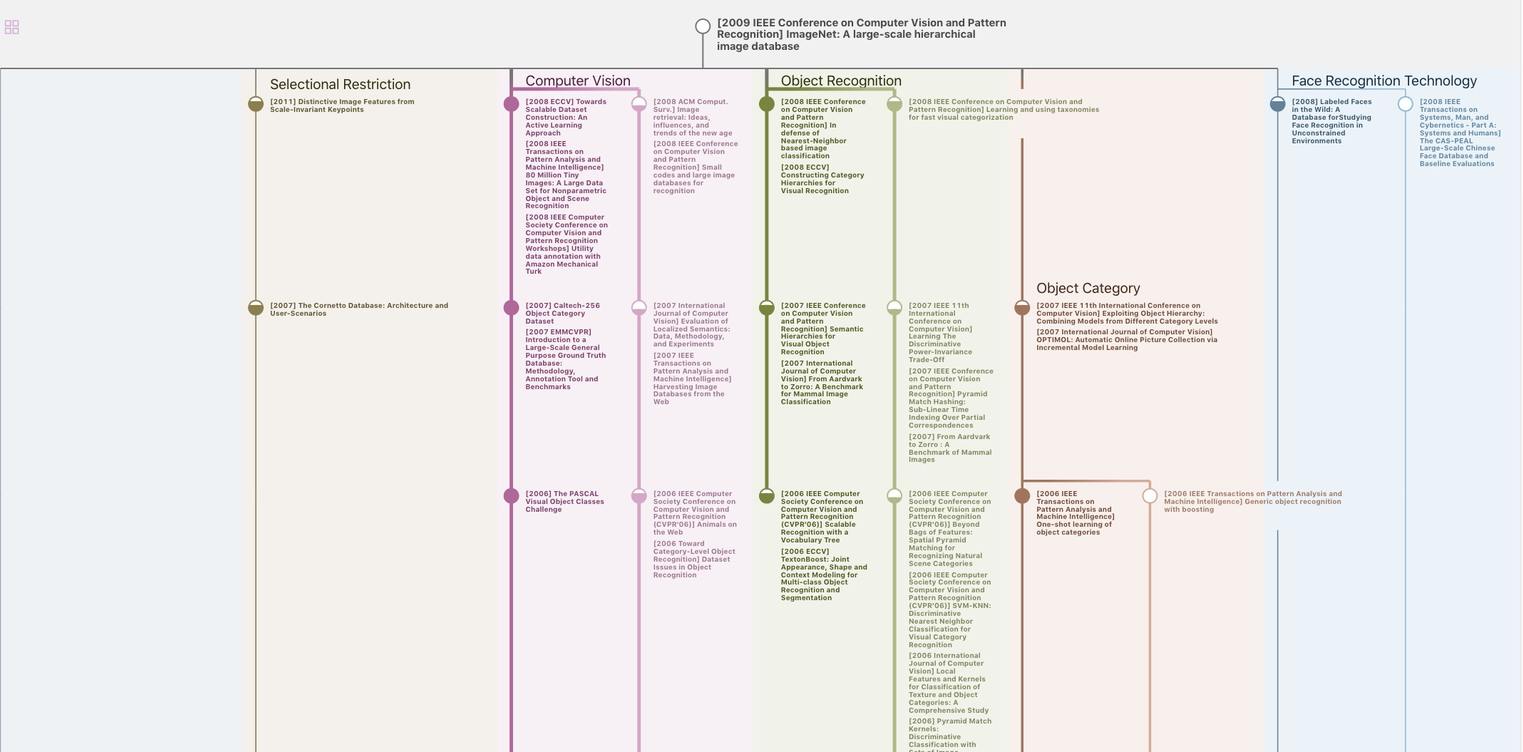
生成溯源树,研究论文发展脉络
Chat Paper
正在生成论文摘要