Subgraph Neighboring Relations Infomax for Inductive Link Prediction on Knowledge Graphs
Proceedings of the Thirty-First International Joint Conference on Artificial Intelligence(2022)
摘要
Inductive link prediction for knowledge graph aims at predicting missing links between unseen entities, those not shown in training stage. Most previous works learn entity-specific embeddings of entities, which cannot handle unseen entities. Recent several methods utilize enclosing subgraph to obtain inductive ability. However, all these works only consider the enclosing part of subgraph without complete neighboring relations, which leads to the issue that partial neighboring relations are neglected, and sparse subgraphs are hard to be handled. To address that, we propose Subgraph Neighboring Relations Infomax, SNRI, which sufficiently exploits complete neighboring relations from two aspects: neighboring relational feature for node feature and neighboring relational path for sparse subgraph. To further model neighboring relations in a global way, we innovatively apply mutual information (MI) maximization for knowledge graph. Experiments show that SNRI outperforms existing state-of-art methods by a large margin on inductive link prediction task, and verify the effectiveness of exploring complete neighboring relations in a global way to characterize node features and reason on sparse subgraphs.
更多查看译文
关键词
inductive link prediction,relations,knowledge,graphs
AI 理解论文
溯源树
样例
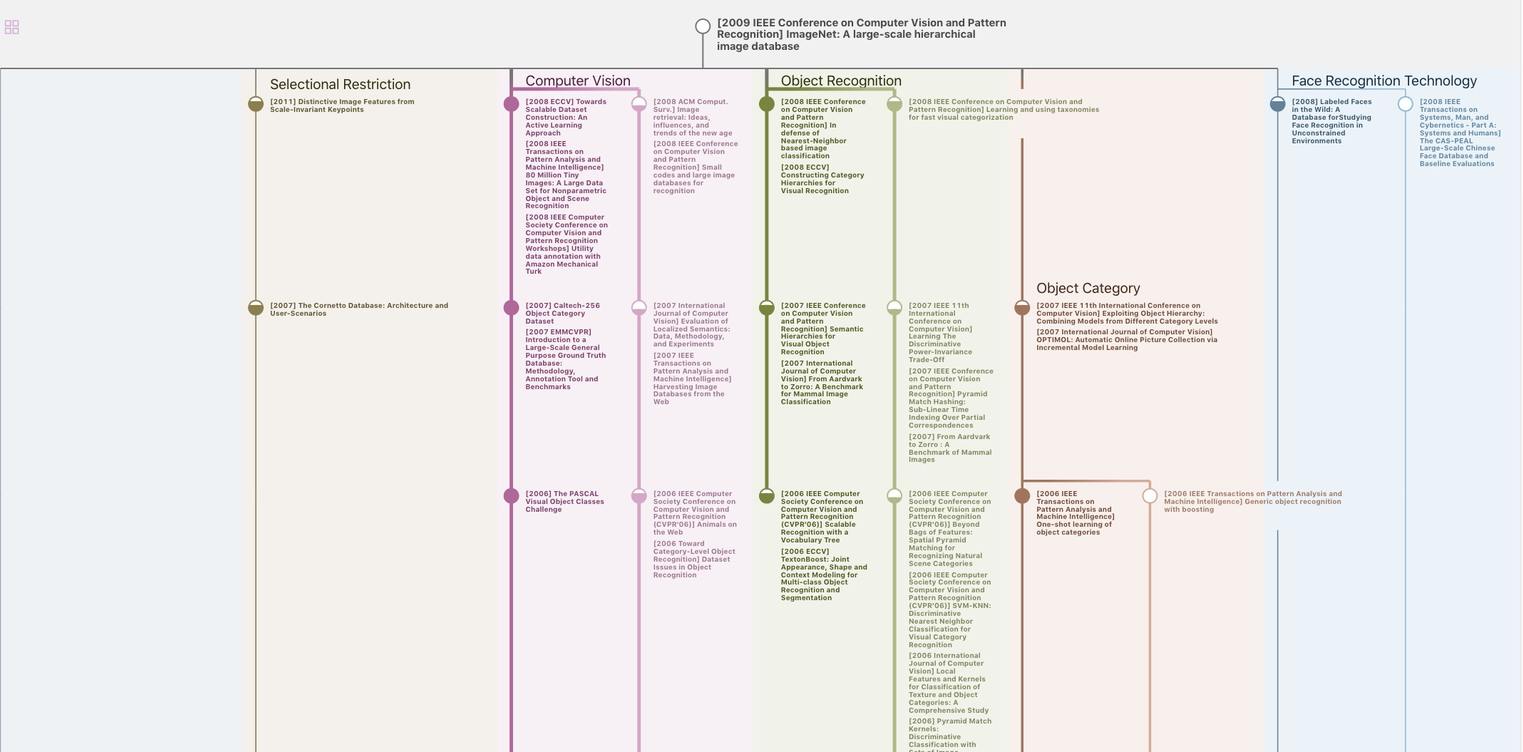
生成溯源树,研究论文发展脉络
Chat Paper
正在生成论文摘要