Special issue: Recent advances in deep learning, biometrics, health informatics and data science
EXPERT SYSTEMS(2022)
摘要
Deep learning is a growing scientific research trend in machine learning and artificial intelligence due to its better performance compared to other machine learning techniques. This special issue focuses on recent advances in deep learning for human health care applications such as biometrics, medical imaging, and data science. The following articles were carefully reviewed and selected for this special issue: Bhurane, Dhok, Sharma, Yuvaraj, Murugappan and Acharya, ‘Diagnosis of Parkinson's disease from electroencephalography signals using linear and self-similarity features’. In this paper, the authors propose a natural (time) domain technique for diagnosing Parkinson's disease (PD). The presented computer-aided diagnosis system can act as an assistive tool to confirm the finding of PD for the clinicians. They demonstrate that using the support vector machines (SVM) classifier, the feature ranking, and the principal component analysis technique, the proposed system can detect the PD signals automatically with maximum accuracy of 99.1% ± 0.1%. Khan, Sharif, Raza, Anjum, Saba and Shad, ‘Skin lesion segmentation and classification: A unified framework of deep neural network features fusion and selection.’ This paper addresses the problem of automated skin lesion diagnosis from dermoscopic images overcoming challenges such as hairs, irregularities, lesion shape, and irrelevant feature extraction. The authors propose a hybrid approach combining optimized colour feature of lesion segmentation improved by an existing saliency approach fused with a novel pixel-based method and deep convolutional neural network (DCNN)-based skin lesion classification. Experimental results of the proposed approach demonstrate remarkable performance on three different datasets. Sivan, Sellappa and Peter J, ‘Proximity-based cloud resource provisioning for deep learning applications in smart healthcare.’ Health professionals can use smart mobile devices to convey recordings of patients and use machine learning-based approaches to process results and get predictions through smart mobile healthcare applications. Due to the nature of deep learning techniques, learning and prediction processes are moved to the cloud. This paper proposes a proximity-based resource provisioning technique that guarantees minimal delay in obtaining inference results with a local mobile cloud system. The authors implemented a healthcare cloud-based system that outperforms the state-of-the-art methods in terms of response time, deadline meeting percentage and system utilization. Arshad, Khan, Sharif, Yasmin, Tavares, Zhang and Satapathy, ‘A multilevel paradigm for deep convolutional neural network features selection with an application to human gait recognition.’ This paper proposes an integrated framework for human gait recognition using deep neural network features fusion and fuzzy entropy controlled skewness approach for best feature selection. Pre-trained CNN models (VGG19 and AlexNet) are used, and their information is mixed by the parallel fusion approach. Remarkable results on four gait analysis datasets show that the fusion of multiple CNN frameworks improves the recognition accuracy and the selection of the best features enhances the system accuracy and even minimizes the execution time. Alizadehsani, Roshanzamir, Abdar, Beykikhoshk, Khosravi, Nahavandi, Plawiak, Tan and Acharya, ‘Hybrid genetic-discretized algorithm to handle data uncertainty in diagnosing stenosis of coronary arteries.’ The authors address the uncertainty coming from noise in the data used for automated coronary artery disease (CAD) detection. They propose a novel new feature selection algorithm for CAD prediction. Authors use the genetic algorithm to determine the hyper-parameters of the SVM kernels. The system with the proposed approach demonstrates high accuracy for the stenosis diagnosis of each main coronary artery, which can help the clinicians validate their manual stenosis diagnosis of right coronary artery (RCA), right coronary artery (RCA), left circumflex (LCX) and artery and left anterior descending (LAD) coronary arteries. The results show that discretization and assurance feature selection can significantly improve the efficiency of classification algorithms. Sampathila, Pavithra and Martis, ‘Computational approach for content-based image retrieval of K-Similar images from brain MR image database.’ The task of retrieving medical images from a large image database becomes more tedious due to variations in the size and shape of the images. This paper proposes a system for content-based medical image retrieval that are relevant to a given query image. Various features such as colour, shape, and texture are exploited using the K-nearest neighbour algorithm to find the minimum distance between query and database images. The authors focus on the application of retrieving the brain MRI images of different planes (coronal, sagittal and transverse) from a dataset of normal and demented subjects. The results demonstrate high accuracy of 95%. Such a tool can be helpful in radiology image retrieval and classification.
更多查看译文
关键词
deep learning,biometrics,recent advances,health informatics
AI 理解论文
溯源树
样例
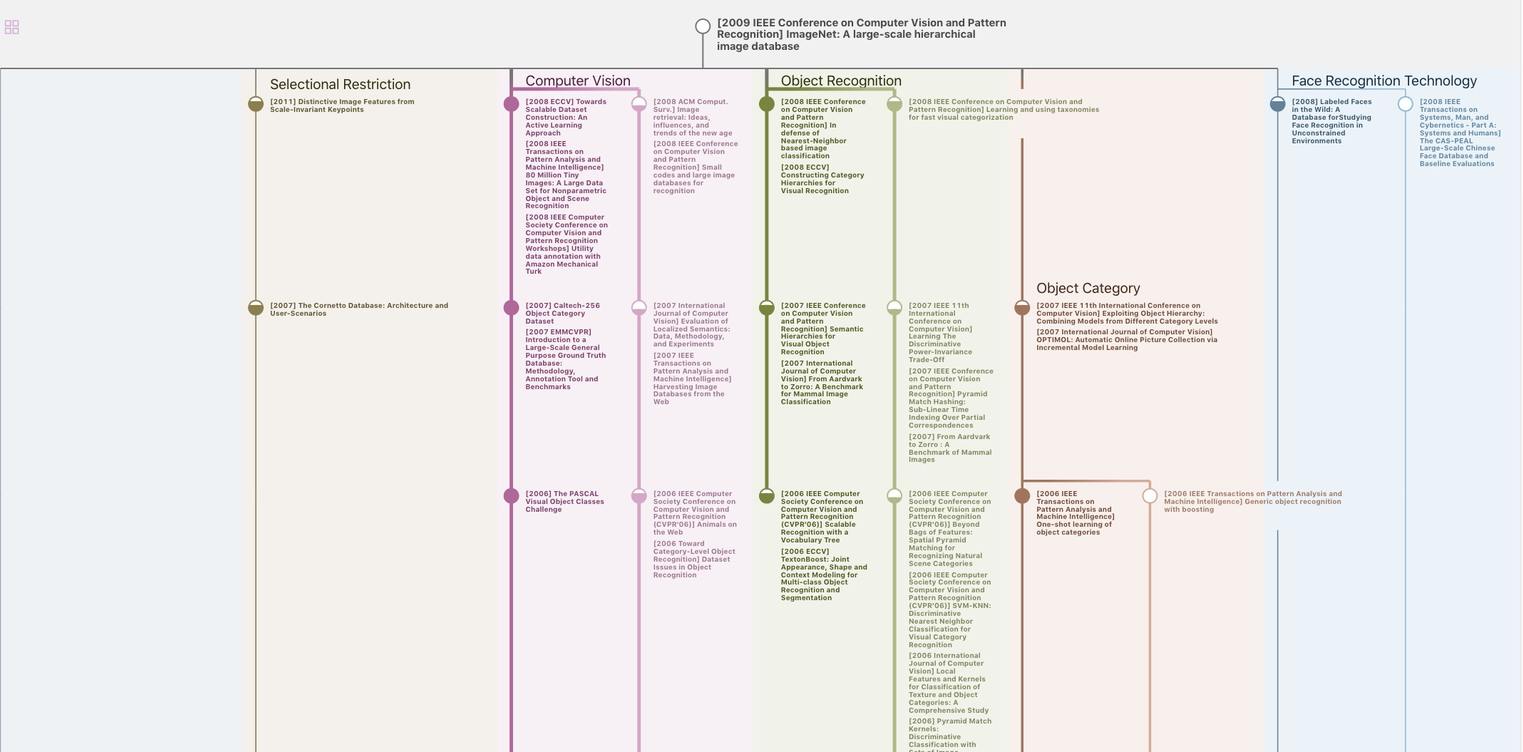
生成溯源树,研究论文发展脉络
Chat Paper
正在生成论文摘要