Evaluating Scale Attention Network for Automatic Brain Tumor Segmentation with Large Multi-parametric MRI Database
Brainlesion: Glioma, Multiple Sclerosis, Stroke and Traumatic Brain Injuries Lecture Notes in Computer Science(2022)
摘要
Automatic segmentation of brain tumors is an essential but challenging step for extracting quantitative imaging biomarkers for accurate tumor detection, diagnosis, prognosis, treatment planning and assessment. This is the 10th year of Brain Tumor Segmentation (BraTS) Challenge that utilizes multi-institutional multi-parametric magnetic resonance imaging (mpMRI) scans for tasks: 1) evaluation the state-of-theart methods for the segmentation of intrinsically heterogeneous brain glioblastoma sub-regions in mpMRI scans; and 2) the evaluation of classification methods to predict the MGMT promoter methylation status at pre-operative baseline scans. We participated the image segmentation task by applying a fully automated segmentation framework that we previously developed in BraTS 2020. This framework, named as scale-attention network, incorporates a dynamic scale attention mechanism to integrate low-level details with high-level feature maps at different scales. Our framework was trained using the 1251 challenge training cases provided by BraTS 2021, and achieved an average Dice Similarity Coefficient (DSC) of 0.9277, 0.8851 and 0.8754, as well as 95% Hausdorff distance (in millimeter) of 4.2242, 15.3981 and 11.6925 on 570 testing cases for whole tumor, tumor core and enhanced tumor, respectively, which ranked itself as the second place in the brain tumor segmentation task of RSNA-ASNR-MICCAI BraTS 2021 Challenge (id: deepX).
更多查看译文
关键词
scale attention network,automatic brain tumor segmentation,multi-parametric
AI 理解论文
溯源树
样例
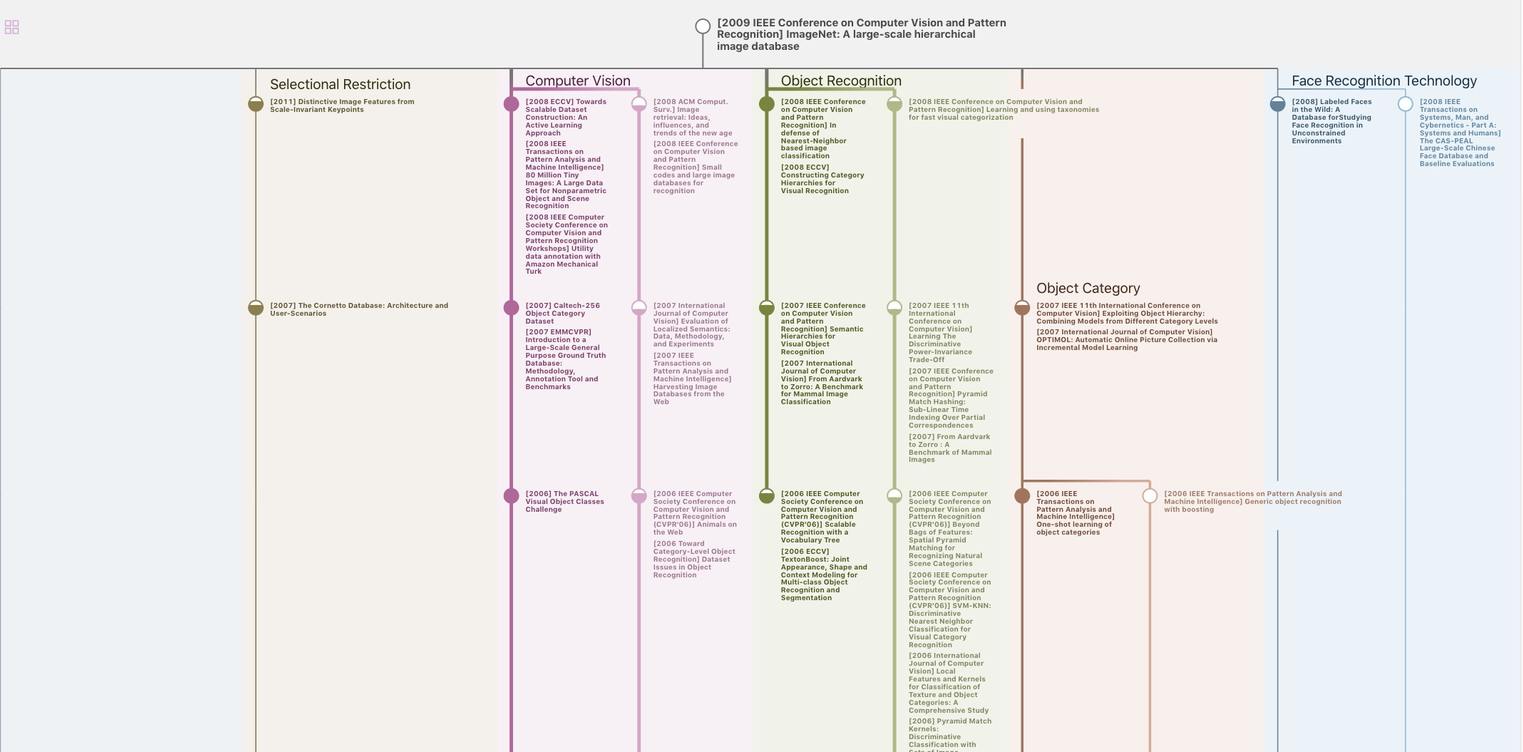
生成溯源树,研究论文发展脉络
Chat Paper
正在生成论文摘要