Domination-Based Selection and Shift-Based Density Estimation for Constrained Multiobjective Optimization
IEEE Transactions on Evolutionary Computation(2023)
摘要
Balancing constraints and objective functions in constrained evolutionary multiobjective optimization is not an easy task. Overemphasis on constraints satisfaction may easily lead to the search to get stuck in local optimal regions, and overemphasis on objectives optimization may lead to substantial search resources wasted on infeasible regions. This article proposes a constrained multiobjective optimization algorithm, called CMOEA-SDE, aiming to achieve a good balance between the above two issues. To do so, CMOEA-SDE presents a strictly constrained dominance relation and a constrained shift-based density estimation strategy. Specifically, the former defines a new dominance relation that considers both constraint satisfaction and the objective functions. It favors good feasible solutions but still leaves room for infeasible solutions to be selected. Unlike most density estimation methods, which only consider the diversity of solutions, our shift-based density estimator covers both the feasibility and the diversity of solutions. That is, our estimator shifts the solutions’ positions based on the extent of the constraints they violate so that solutions violating constraints more severely are shifted to crowded areas, thus being eliminated early. Systematic experiments were conducted on four benchmark test suites and six real-world constrained multiobjective optimization problems. The experimental results suggest that the proposed algorithm can achieve very competitive performance against state-of-the-art constrained multiobjective evolutionary algorithms.
更多查看译文
关键词
density estimation,optimization,selection,domination-based,shift-based
AI 理解论文
溯源树
样例
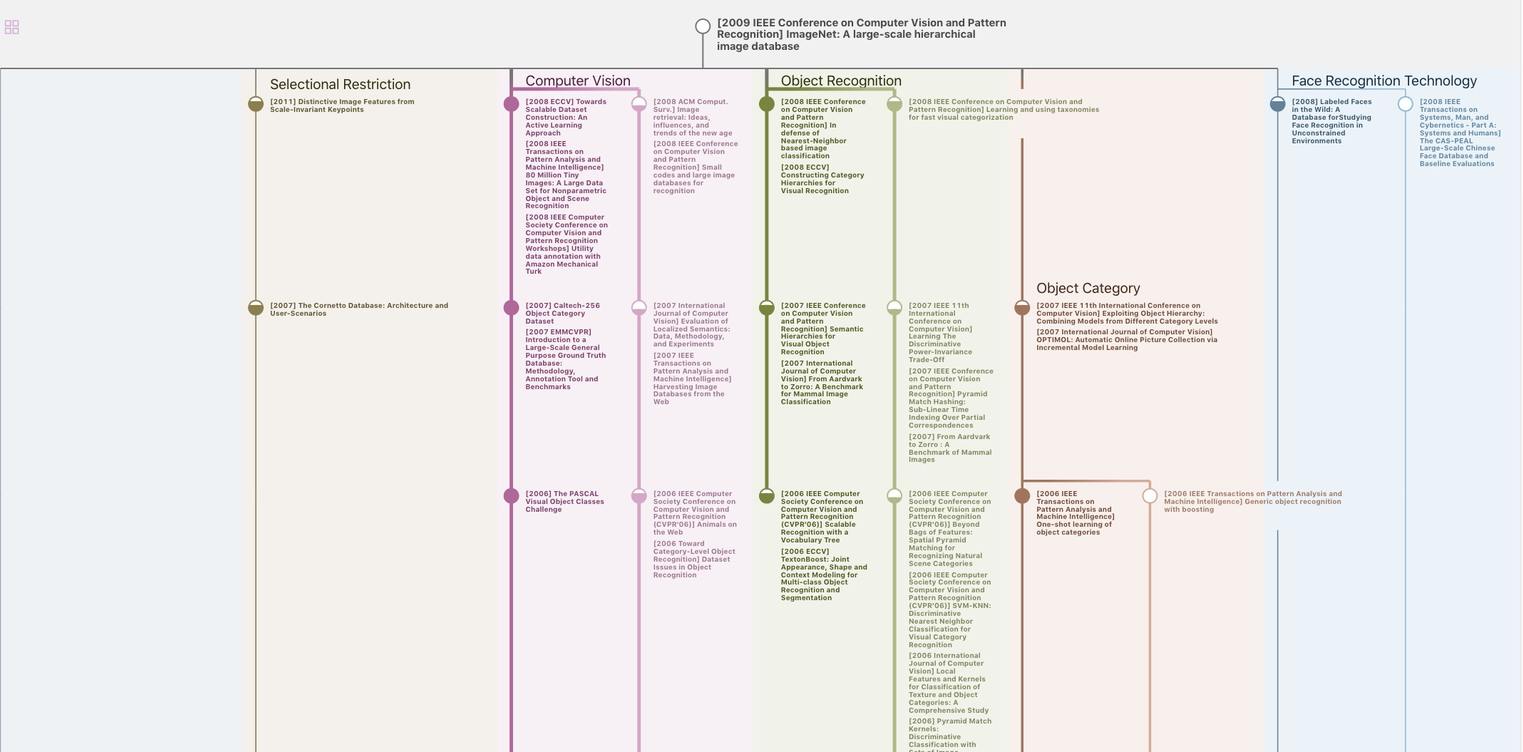
生成溯源树,研究论文发展脉络
Chat Paper
正在生成论文摘要