Multimodal Self-Paced Locality-Preserving Learning for Diagnosis of Alzheimer's Disease
IEEE Transactions on Cognitive and Developmental Systems(2023)
摘要
Alzheimer's disease (AD) is an irreversible neurodegenerative disease that severely impairs human thinking and memory. The accurate diagnosis of AD and its prodromal stages, such as mild cognitive impairment (MCI), is very important for timely treatment or possible interventions of AD. Recent studies have shown that multiple neuroimaging and biological measures contain supplementary information for diagnosis and prognosis. Most existing methods are proposed to simply integrate the multimodal data and train the model using all samples once, which do not fully explore the structural information across the different modalities and ignore the significance of sample learning in the training process. In this article, we propose a multimodal self-paced locality-preserving learning (MSLPL) framework to preserve the inherent structural relationships of the original data and realize the sample selection process from "simple" to "complex." Specifically, the model can project the neuroimaging and genetic data into the label space and learn dimensionality reduction manners with preserving locality structure. Meanwhile, the contributions of each sample are adaptively evaluated by weighting optimization so that the impact of noises can be reduced during model training by self-paced learning (SPL). Finally, a multikernel support vector machine (MK-SVM) is used to fuse the features selected from different modalities for the final prediction. We evaluate MSLPL on 913 subjects from the AD neuroimaging initiative (ADNI) database with imaging and genetic data. The experimental results demonstrate that the proposed method can achieve better classification performances compared with the start-of-the-art multimodality-based methods.
更多查看译文
关键词
Alzheimer's disease (AD),feature selection,locality preserving projection,multimodal learning,self-paced learning (SPL)
AI 理解论文
溯源树
样例
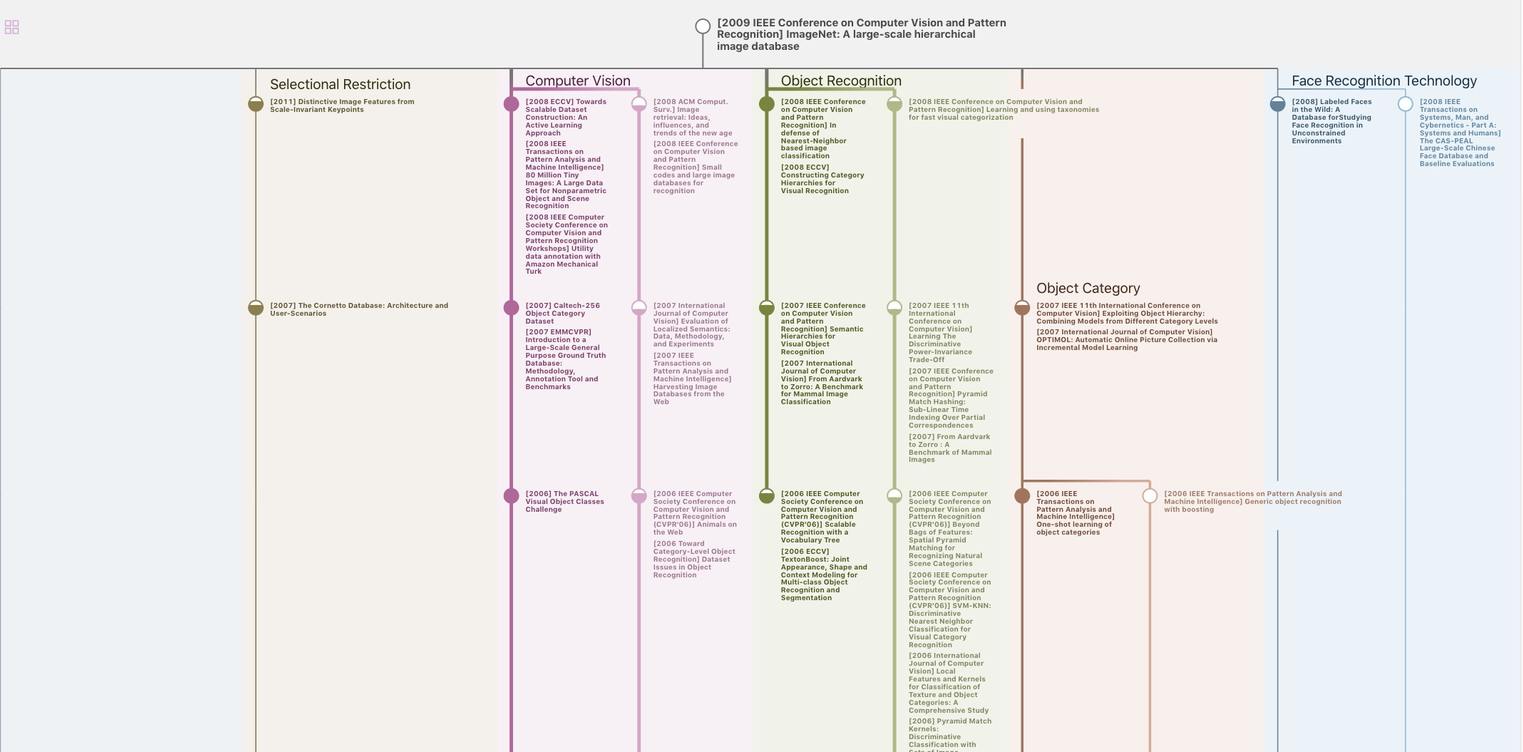
生成溯源树,研究论文发展脉络
Chat Paper
正在生成论文摘要