Vertical federated learning-based feature selection with non-overlapping sample utilization
Expert Systems with Applications(2022)
摘要
Vertical federated learning (VFL) is a privacy preserving collaborative machine learning technique designed for distributed learning scenarios in which data from different parties have overlap in the sample space. In this paper, a VFL method for feature selection, which is an effective dimensionality reduction technique that selects a subset of informative features from high-dimensional data by eliminating irrelevant and redundant features, is proposed. Because of the potential insufficiency of useful information for learning informative features and the difficulty in sharing raw data among parties due to the increasing awareness of data privacy protection, it is desirable to exploit information from multiple parties without raw data sharing. In this paper, we propose a VFL-based feature selection method that leverages deep learning models as well as complementary information from features in the same samples at multiple parties without data disclosure. In order to further improve feature selection performance, information of samples that do not have features appearing in all parties are also utilized. Promising results in extensive experiments show the effectiveness of the proposed approach in terms of collaborative feature selection without data sharing.
更多查看译文
关键词
Feature selection,Vertical federated learning,Privacy protection,Deep learning
AI 理解论文
溯源树
样例
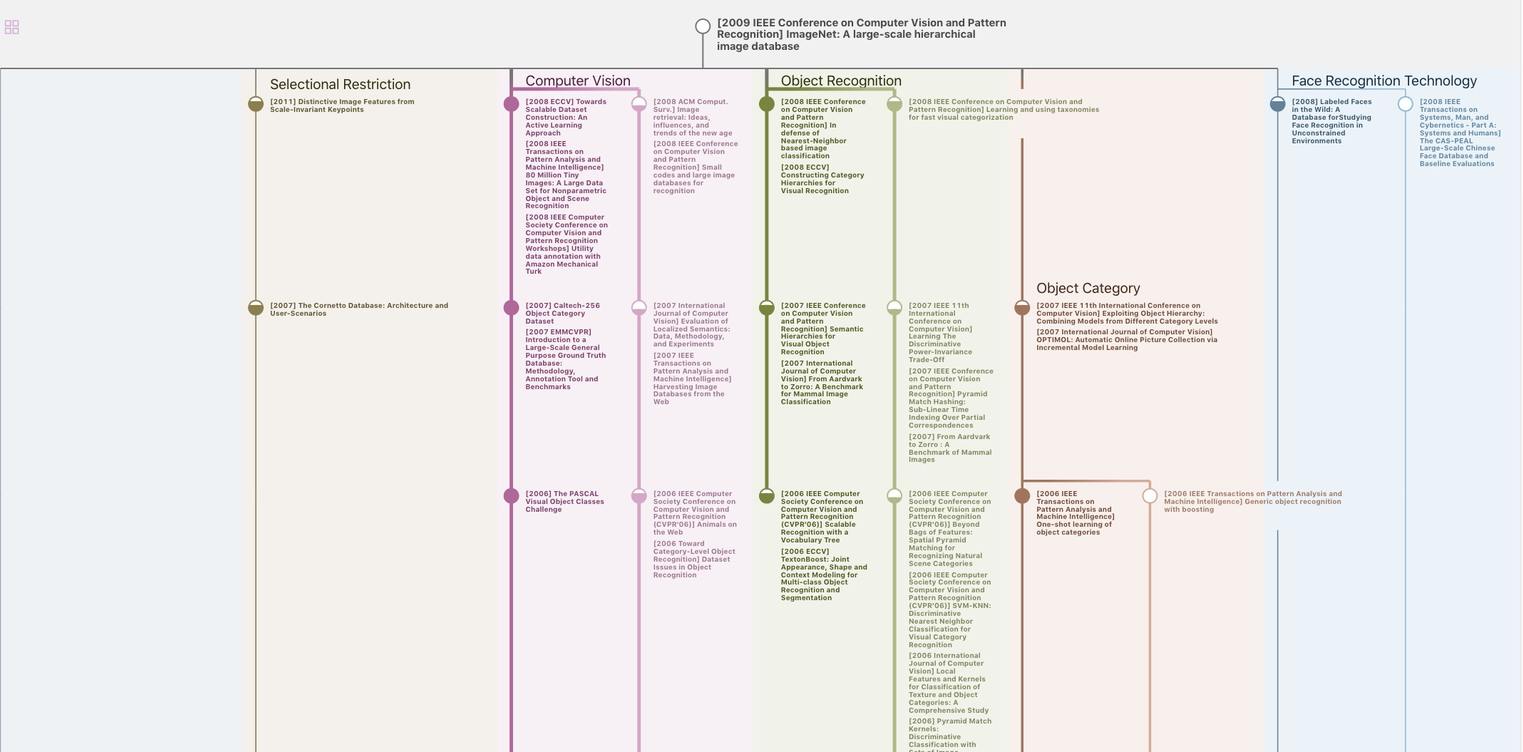
生成溯源树,研究论文发展脉络
Chat Paper
正在生成论文摘要