Brain Tumor Segmentation with Self-supervised Enhance Region Post-processing
Brainlesion: Glioma, Multiple Sclerosis, Stroke and Traumatic Brain Injuries Lecture Notes in Computer Science(2022)
摘要
In this paper, we extend the previous research works on the robust multi-sequences segmentation methods which allows to consider all available information from MRI scans by the composition of T1, T1C, T2 and T2-FLAIR sequences. It is based on the clinical radiology hypothesis and presents an efficient approach to combining and matching 3D methods to search for areas of comprised the GD-enhancing tumor in order to significantly improve the model's performance of the particular applied numerical problem of brain tumor segmentation. Proposed in this paper method also demonstrates strong improvement on the segmentation problem. This conclusion was done with respect to Dice and Hausdorff metric, Sensitivity and Specificity compare to identical training/test procedure based only on any single sequence and regardless of the chosen neural network architecture. We achieved on the test set of 0.866, 0.921 and 0.869 for ET, WT, and TC Dice scores. Obtained results demonstrate significant performance improvement while combining several 3D approaches for considered tasks of brain tumor segmentation. In this work we provide the comparison of various 3D and 2D approaches, pre-processing to self-supervised clean data, post-processing optimization methods and the different backbone architectures.
更多查看译文
关键词
Medical imaging,Deep learning,Neural network,Segmentation,Brain,MRI
AI 理解论文
溯源树
样例
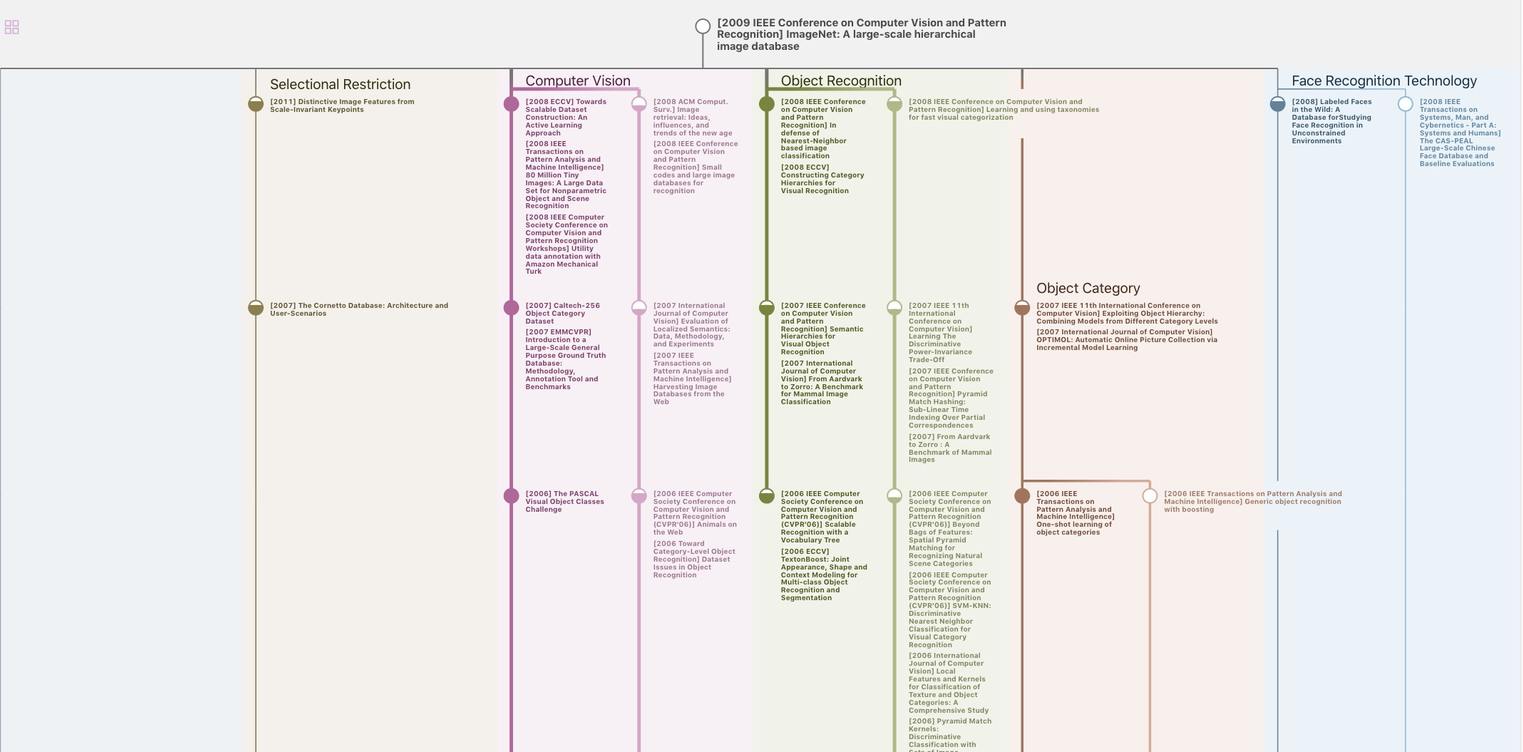
生成溯源树,研究论文发展脉络
Chat Paper
正在生成论文摘要