Shallow or deep? Training an autoencoder to detect anomalous flows in a retail payment system
Latin American Journal of Central Banking(2021)
摘要
Our paper applies a deep neural network autoencoder (AE) to detect anomalous payment flows in Canada's retail batch clearing payments system, the Automated Clearing Settlement System (ACSS). We aim to investigate an AE's potential for detecting complex changes in the liquidity outflows between participants, which could provide an early warning indication for exceptionally large outflows for a participant. As the Canadian financial system has neither faced bank runs nor severe liquidity shocks in recent history, we trained our models on “normal” data and evaluated them out-of-sample using test data drawn from two constructed scenarios: a sample derived from the largest 1% of observed historical multilateral net outflows and a sample drawn from a simulated bank run. In both cases, the trained AE performed well by producing larger than usual reconstruction errors. Our approach highlights the efficacy of a class of unsupervised machine learning methods as a useful component of a system operator's risk management toolkit.
更多查看译文
关键词
Anomaly detection,Autoencoder,Neural network,ACSS,Financial market infrastructure
AI 理解论文
溯源树
样例
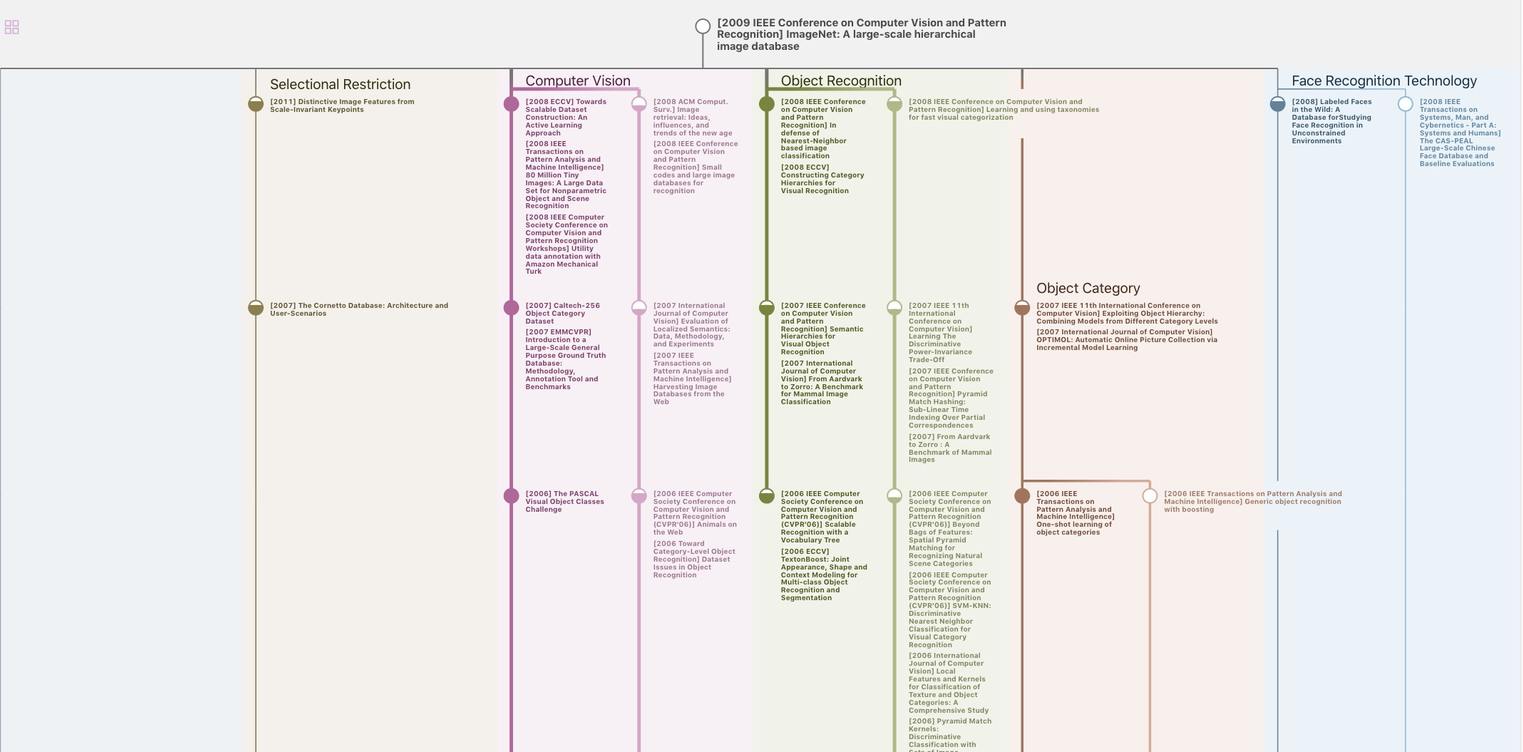
生成溯源树,研究论文发展脉络
Chat Paper
正在生成论文摘要