Auto-contouring FDG-PET/MR images for cervical cancer radiation therapy: An intelligent sequential approach using focally trained, shallow U-Nets
Intelligence-Based Medicine(2021)
摘要
Background
Manual contouring for radiation therapy planning remains the most laborious and time consuming part in the radiation therapy workflow. Particularly for cervical cancer, this task is complicated by the complex female pelvic anatomy and the concomitant dependence on 18F-labeled Fluorodeoxyglucose (FDG) positron emission tomography (PET) and magnetic resonance (MR) images. Using deep learning, we propose a new auto-contouring method for FDG-PET/MR based cervical cancer radiation therapy by combining the high level anatomical topography and radiological properties, to the low-level pixel wise deep-learning based semantic segmentation.
Materials/methods
The proposed method: 1) takes advantage of PET data and left/right anatomical symmetry, creating sub-volumes that are centered on the structures to be contoured. 2) Uses a 3D shallow U-Net (sU-Net) model with an encoder depth of 2.3) Applies the successive training of 3 consecutive sU-Nets in a feed forward strategy. 4) Employs, instead of the usual generalized dice loss function (GDL), a patch dice loss function (PDL) that takes into account the Dice similarity index (DSI) at the level of each training patch. Experimental analysis was conducted on a set of 13 PET/MR images was using a leave-one-out strategy.
Results
Despite the limited data availability, 5 anatomical structures - the gross tumor volume, bladder, anorectum, and bilateral femurs - were accurately (DSI = 0.78), rapidly (1.9 s/structure), and automatically delineated by our algorithm. Overall, PDL achieved a better performance than GDL and DSI was higher for organs at risk (OARs) with solid tissue (e.g. femurs) than for OARs with air-filled soft tissues (e.g. anorectum).
Conclusion
The presented workflow successfully addresses the challenge of auto-contouring in FDG-PET/MR based cervical cancer. It is expected to expedite the cervical cancer radiation therapy workflow in both, conventional and adaptive radiation therapy settings.
更多查看译文
关键词
Cervical cancer,Deep learning,Image segmentation,PET/MR Based radiation therapy,U-net
AI 理解论文
溯源树
样例
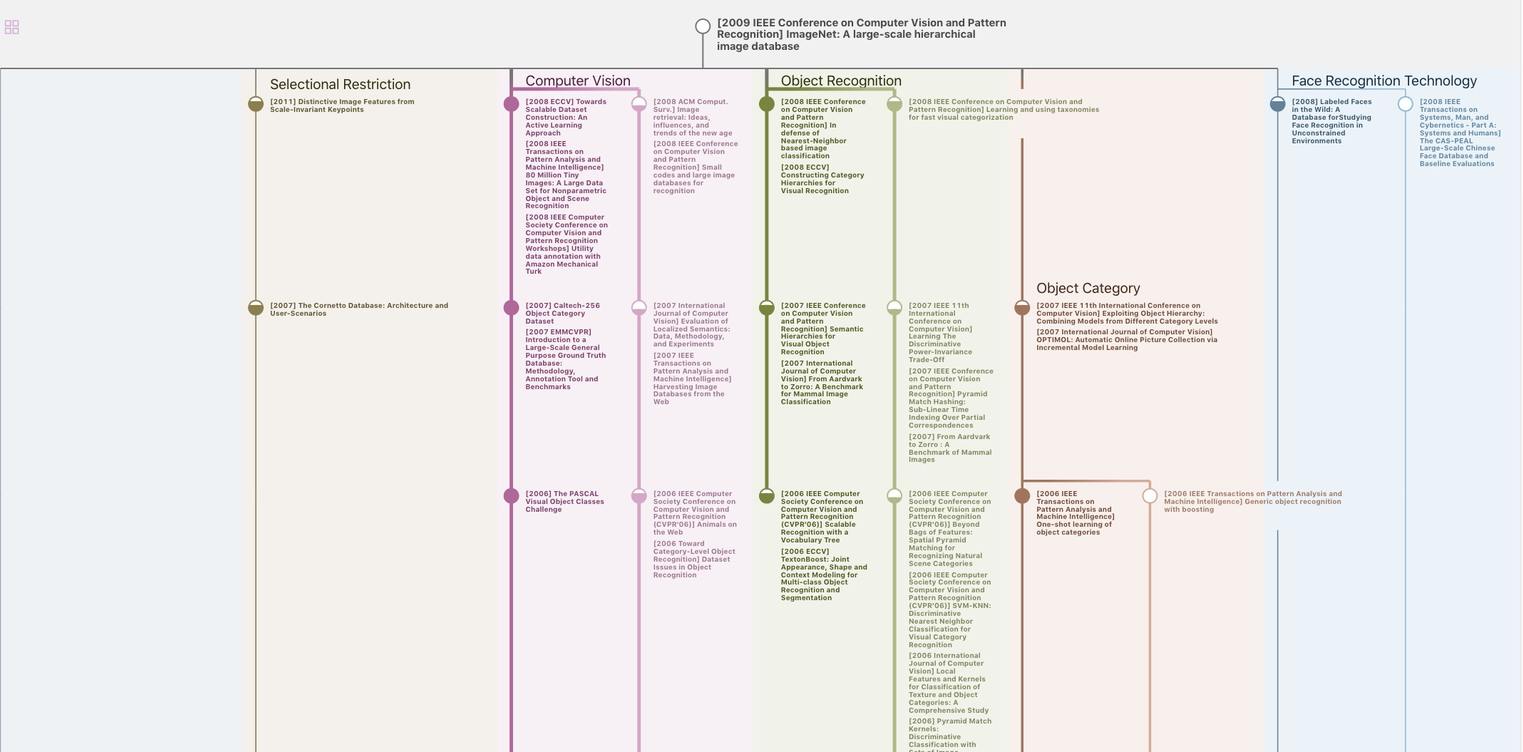
生成溯源树,研究论文发展脉络
Chat Paper
正在生成论文摘要