Towards early sepsis detection from measurements at the general ward through deep learning
Intelligence-Based Medicine(2021)
摘要
Background
Sepsis is one of the leading causes of death in the hospital. Several warning scores have been developed to categorize patients’ degrees of illness, with the purpose of recognizing sepsis onset at an early stage and consequently reducing time before starting treatment. The most accurate classification method, known as the SOFA score, is developed for use in the intensive care unit (ICU).
Objective
Sepsis is not exclusively developing in the ICU and may occur in any hospitalized patient. Therefore, a reliable method for sepsis recognition using routinely gathered information from the general ward is of major importance.
Methods
Recently, the use of computational methods has been proposed for early sepsis prediction. Multiple sepsis classifiers have been devised using machine learning methods. We validated a linear classification model and improved upon it using a deep neural network trained on data from the MIMIC-III database.
Results
The reference model approach yielded an Area Under Precision-Recall Curve (AUPRC) of 0.45 for a 3-h prediction time. Our newly constructed deep neural network outperformed the reference model, reaching an AUPRC of 0.49 for a 3-h prediction time. These results demonstrate to be robust for multiple prediction times, utilizing different datasets and multiple sepsis criteria.
Conclusions
Our results are comparable to a high-resolution model, yet using only 8 simple and commonly performed measurements, instead of the complex set of dozens of measurements leveraged by other authors. Moreover, our method proves to adapt to different datasets and sepsis criteria, yielding a high accuracy. Therefore, sepsis onset prediction may also be viable in less monitored environments in the hospital, such as the general ward and the emergency room.
更多查看译文
关键词
Sepsis prediction,Clinical decision making,Artificial intelligence,Deep learning,Intensive care
AI 理解论文
溯源树
样例
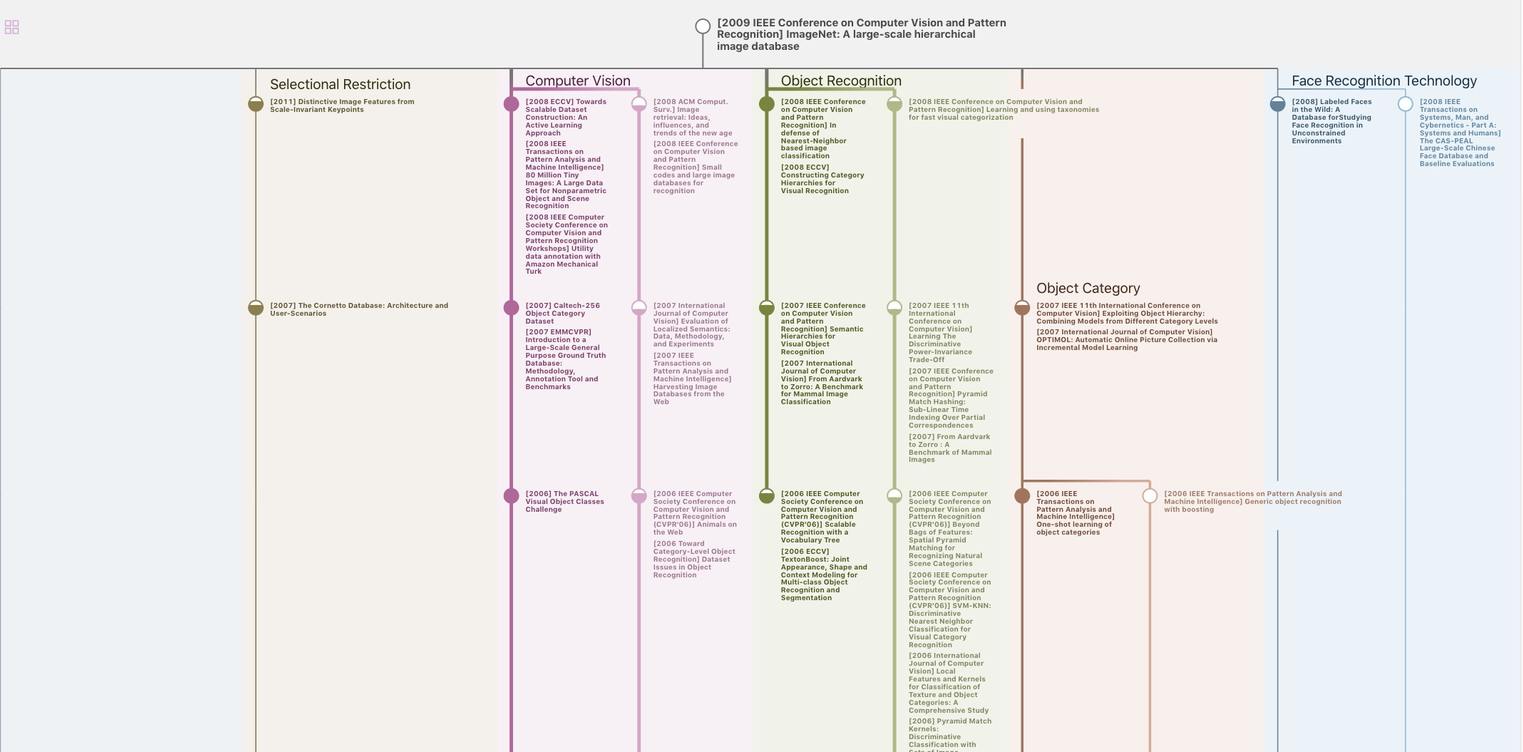
生成溯源树,研究论文发展脉络
Chat Paper
正在生成论文摘要