Computer-vision based method for quantifying rising from chair in Parkinson's disease patients
Intelligence-Based Medicine(2022)
摘要
Background
The ability to arise from a sitting to a standing position is often impaired in Parkinson's disease (PD). This impairment is associated with an increased risk of falling, and higher risk of dementia. We propose a novel approach to estimate Movement Disorder Society Unified PD Rating Scale (MDS-UPDRS) ratings for “item 3.9” (arising from chair) using a computer vision-based method, whereby we use clinically informed reasoning to engineer a small number of informative features from high dimensional markerless pose estimation data.
Methods
We analysed 447 videos collected via the KELVIN-PD™ platform, recorded in clinical settings at multiple sites, using commercially available mobile smart devices. Each video showed an examination for item 3.9 of the MDS-UPDRS and had an associated severity rating from a trained clinician on the 5-point scale (0, 1, 2, 3 or 4).The deep learning library OpenPose was used to extract pose estimation key points from each frame of the videos, resulting in time-series signals for each key point. From these signals, features were extracted which capture relevant characteristics of the movement; velocity variation, smoothness, whether the patient used their hands to push themselves up, how stooped the patient was while sitting and how upright the patient was when fully standing. These features were used to train an ordinal classification system (with one class for each of the possible ratings on the UPDRS), based on a series of random forest classifiers.
Results
The UPDRS ratings estimated by this system, using leave-one-out cross validation, corresponded exactly to the ratings made by clinicians in 79% of videos, and were within one of those made by clinicians in 100% of cases. The system was able to distinguish normal from Parkinsonian movement with a sensitivity of 62.8% and a specificity of 90.3%. Analysis of misclassified examples highlighted the potential of the system to detect potentially mislabelled data.
Conclusion
We show that our computer-vision based method can accurately quantify PD patients’ ability to perform the arising from chair action. As far as we are aware this is the first study estimating scores for item 3.9 of the MDS-UPDRS from singular monocular video. This approach can help prevent human error by identifying unusual clinician ratings, and provides promise for such a system being used routinely for clinical assessments, either locally or remotely, with potential for use as stratification and outcome measures in clinical trials.
更多查看译文
关键词
Parkinson's disease,Arising from chair,Time series analysis,Computer vision,Pose estimation,Machine learning
AI 理解论文
溯源树
样例
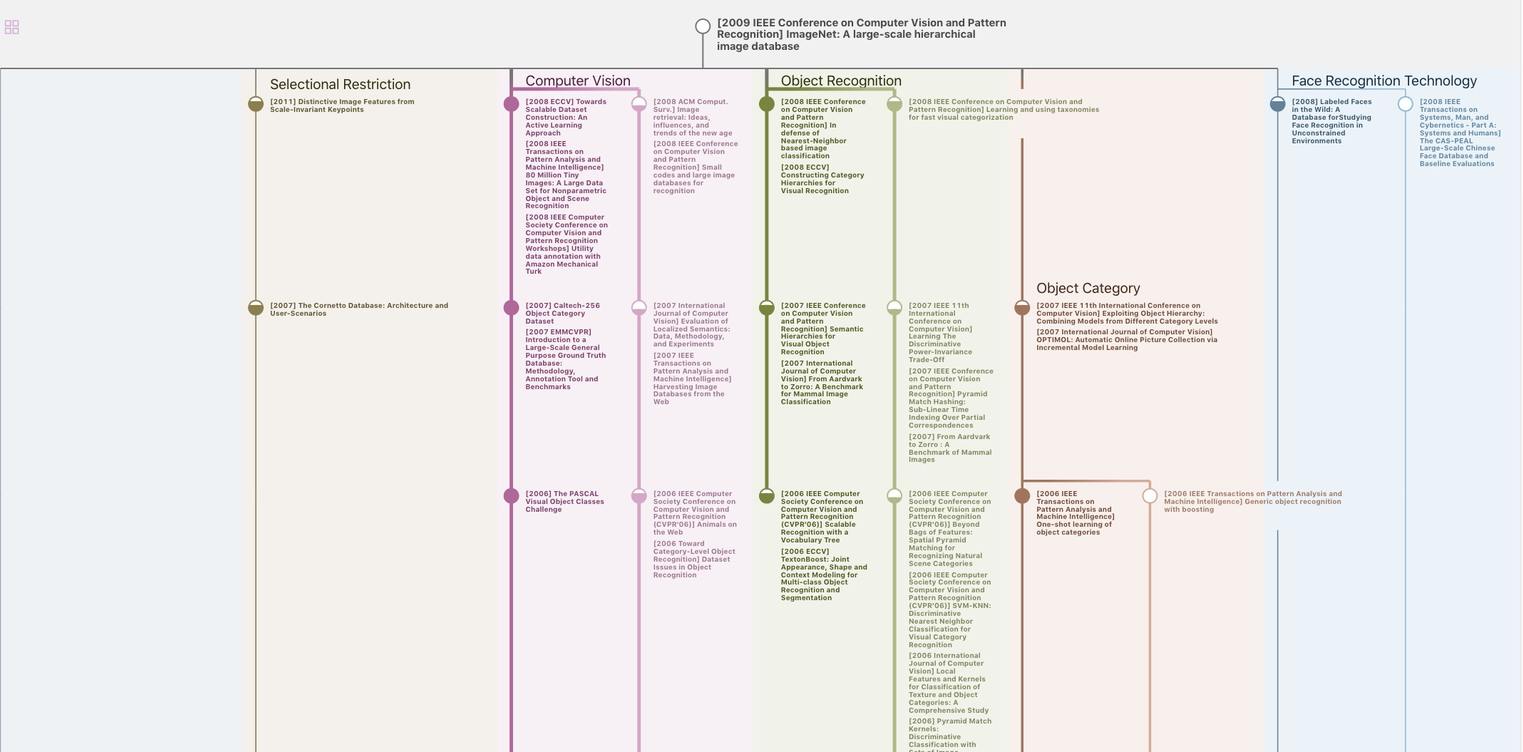
生成溯源树,研究论文发展脉络
Chat Paper
正在生成论文摘要