Research of the Distribution of Tongue Features of Diabetic Population Based on Unsupervised Learning Technology
EVIDENCE-BASED COMPLEMENTARY AND ALTERNATIVE MEDICINE(2022)
摘要
Background. The prevalence of diabetes increases year by year, posing a severe threat to human health. Current treatments are difficult to prevent the progression of diabetes and its complications. It is imperative to carry out individualized treatment of diabetes, but current diagnostic methods are difficult to specify an individualized treatment plan. Objective. Clarify the distribution law of tongue features of the diabetic population, and provide the diagnostic basis for individualized treatment of traditional Chinese medicine (TCM) in the treatment of diabetes. Methods. We use the TFDA-1 tongue diagnosis instrument to collect tongue images of people with diabetes and accurately calculate the color features, texture features, and tongue coating ratio features through the Tongue Diagnosis Analysis System (TDAS). Then, we used K-means and Self-organizing Maps (SOM) networks to analyze the distribution of tongue features in diabetic people. Statistical analysis of TDAS features was used to identify differences between clusters. Results. The silhouette coefficient of the K-means clustering result is 0.194, and the silhouette coefficient of the SOM clustering result is 0.127. SOM Cluster 3 and Cluster 4 are derived from K-means Cluster 1, and the intersections account for (76.7% 97.5%) and (22.3% and 70.4%), respectively. K-means Cluster 2 and SOM Cluster 1 are highly overlapping, and the intersection accounts for the ratios of 66.9% and 95.0%. K-means Cluster 3 and SOM Cluster 2 are highly overlaid, and the intersection ratio is 94.1% and 82.1%. For the clustering results of K-means, TB-a and TC-a of Cluster 3 are the highest (P < 0.001), TB-a of Cluster 2 is the lowest (P < 0.001), and TB-a of Cluster 1 is between Cluster 2 and Cluster 3 (P < 0.001). Cluster 1 has the highest TB-b and TC-b (P < 0.001), Cluster 2 has the lowest TB-b and TC-b (P < 0.001), and TB-b and TC-b of Cluster 3 are between Cluster 1 and Cluster 2 (P < 0.001). Cluster 1 has the highest TB-ASM and TC-ASM (P < 0.001), Cluster 3 has the lowest TB-ASM and TC-ASM (P < 0.001), and TB-ASM and TC-ASM of Cluster 2 are between the Cluster 1 and Cluster 3 (P < 0.001). CON, ENT, and MEAN show the opposite trend. Cluster 2 had the highest Per-all (P < 0.001). SOM divides K-means Cluster 1 into two categories. There is almost no difference in texture features between Cluster 3 and Cluster 4 in the SOM clustering results. Cluster 3's TB-L, TC-L, and Per-all are lower than Cluster 4 (P < 0.001), Cluster 3's TB-a, TC-a, TB-b, TC-b, and Per-part are higher than Cluster 4 (P < 0.001). Conclusions. The precise tongue image features calculated by TDAS are the basis for characterizing the disease state of diabetic people. Unsupervised learning technology combined with statistical analysis is an important means to discover subtle changes in the tongue features of diabetic people. The machine vision analysis method based on unsupervised machine learning technology realizes the classification of the diabetic population based on fine tongue features. It provides a diagnostic basis for the designated diabetes TCM treatment plan.
更多查看译文
关键词
tongue features,unsupervised learning technology,diabetic population,unsupervised learning
AI 理解论文
溯源树
样例
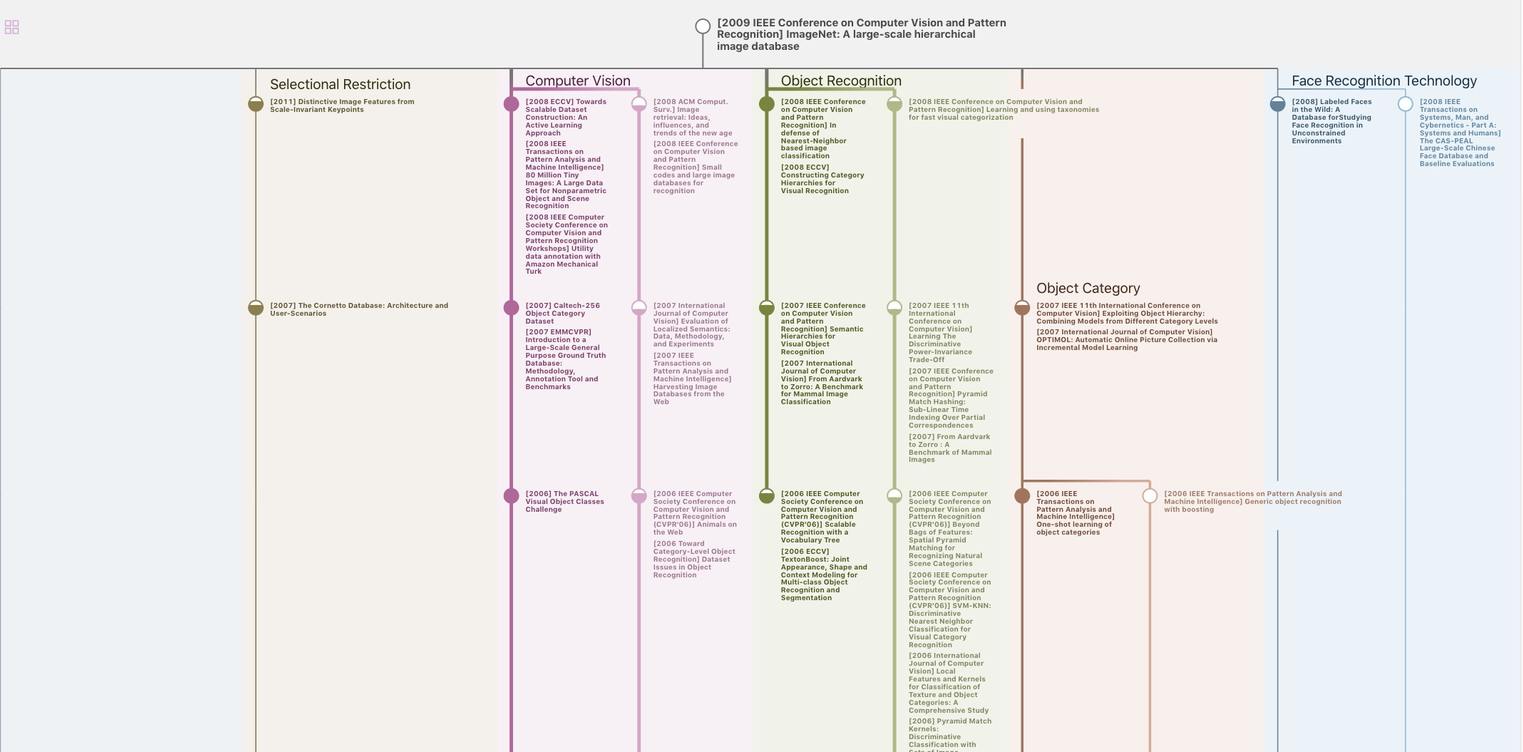
生成溯源树,研究论文发展脉络
Chat Paper
正在生成论文摘要