Internal and external validation of machine learning-assisted prediction models for mechanical ventilation-associated severe acute kidney injury.
Australian critical care : official journal of the Confederation of Australian Critical Care Nurses(2022)
摘要
BACKGROUND:Currently, very few preventive or therapeutic strategies are used for mechanical ventilation (MV)-associated severe acute kidney injury (AKI).
OBJECTIVES:We developed clinical prediction models to detect the onset of severe AKI in the first week of intensive care unit (ICU) stay during the initiation of MV.
METHODS:A large ICU database Medical Information Mart for Intensive Care IV (MIMIC-IV) was analysed retrospectively. Data were collected from the clinical information recorded at the time of ICU admission and during the initial 12 h of MV. Using univariate and multivariate analyses, the predictors were selected successively. For model development, two machine learning algorithms were compared. The primary goal was to predict the development of AKI stage 2 or 3 (AKI-23) and AKI stage 3 (AKI-3) in the first week of patients' ICU stay after initial 12 h of MV. The developed models were externally validated using another multicentre ICU database (eICU Collaborative Research Database, eICU) and evaluated in various patient subpopulations.
RESULTS:Models were developed using data from the development cohort (MIMIC-IV: 2008-2016; n = 3986); the random forest algorithm outperformed the logistic regression algorithm. In the internal (MIMIC-IV: 2017-2019; n = 1210) and external (eICU; n = 1494) validation cohorts, the incidences of AKI-23 were 154 (12.7%) and 119 (8.0%), respectively, with areas under the receiver operator characteristic curve of 0.78 (95% confidence interval [CI]: 0.74-0.82) and 0.80 (95% CI: 0.76-0.84); the incidences of AKI-3 were 81 (6.7%) and 67 (4.5%), with areas under the receiver operator characteristic curve of 0.81 (95% CI: 0.76-0.87) and 0.80 (95% CI: 0.73-0.86), respectively.
CONCLUSIONS:Models driven by machine learning and based on routine clinical data may facilitate the early prediction of MV-associated severe AKI. The validated models can be found at: https://apoet.shinyapps.io/mv_aki_2021_v2/.
更多查看译文
AI 理解论文
溯源树
样例
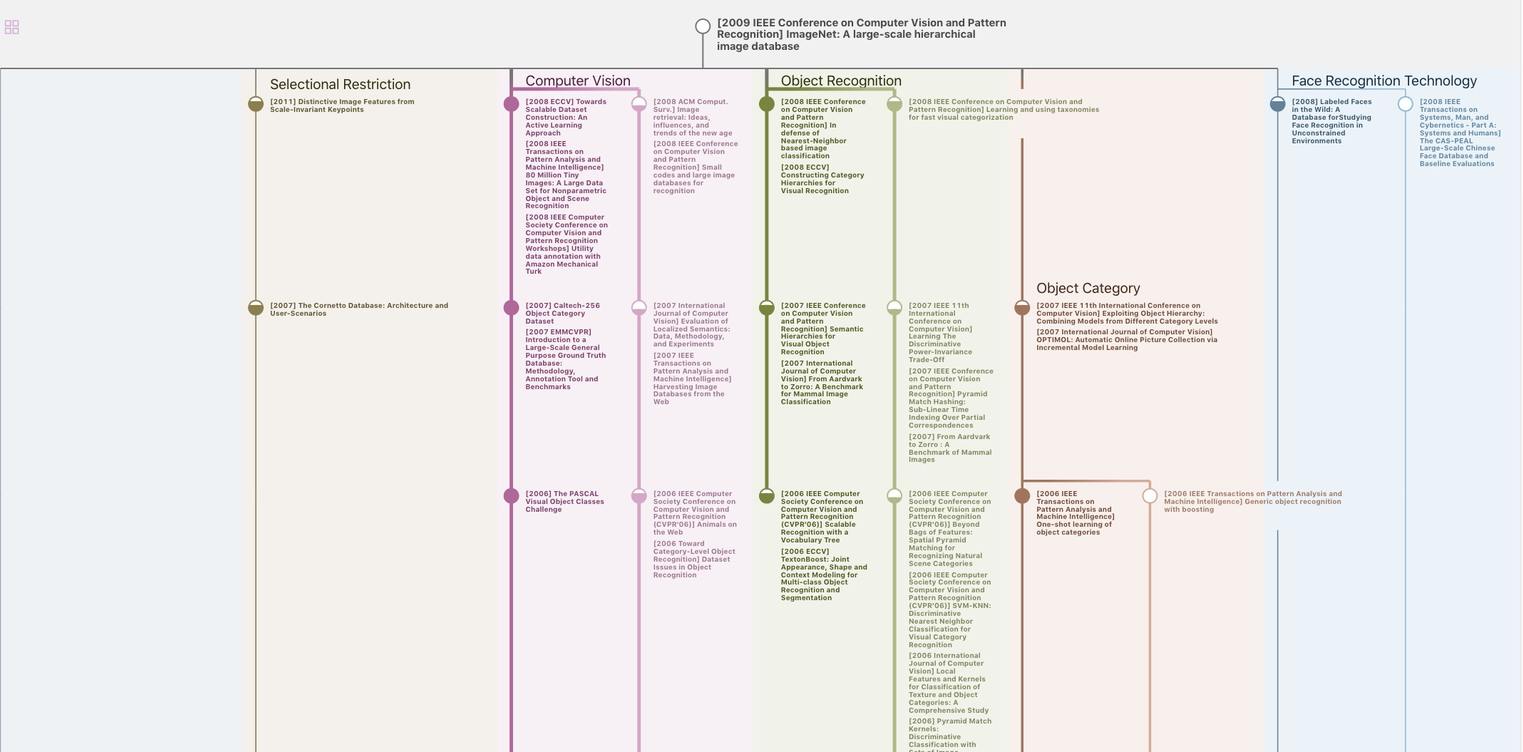
生成溯源树,研究论文发展脉络
Chat Paper
正在生成论文摘要