Anomalous behaviour in loss-gradient based interpretability methods
arxiv(2022)
摘要
Loss-gradients are used to interpret the decision making process of deep learning models. In this work, we evaluate loss-gradient based attribution methods by occluding parts of the input and comparing the performance of the occluded input to the original input. We observe that the occluded input has better performance than the original across the test dataset under certain conditions. Similar behaviour is observed in sound and image recognition tasks. We explore different loss-gradient attribution methods, occlusion levels and replacement values to explain the phenomenon of performance improvement under occlusion.
更多查看译文
关键词
interpretability methods,anomalous behaviour,loss-gradient
AI 理解论文
溯源树
样例
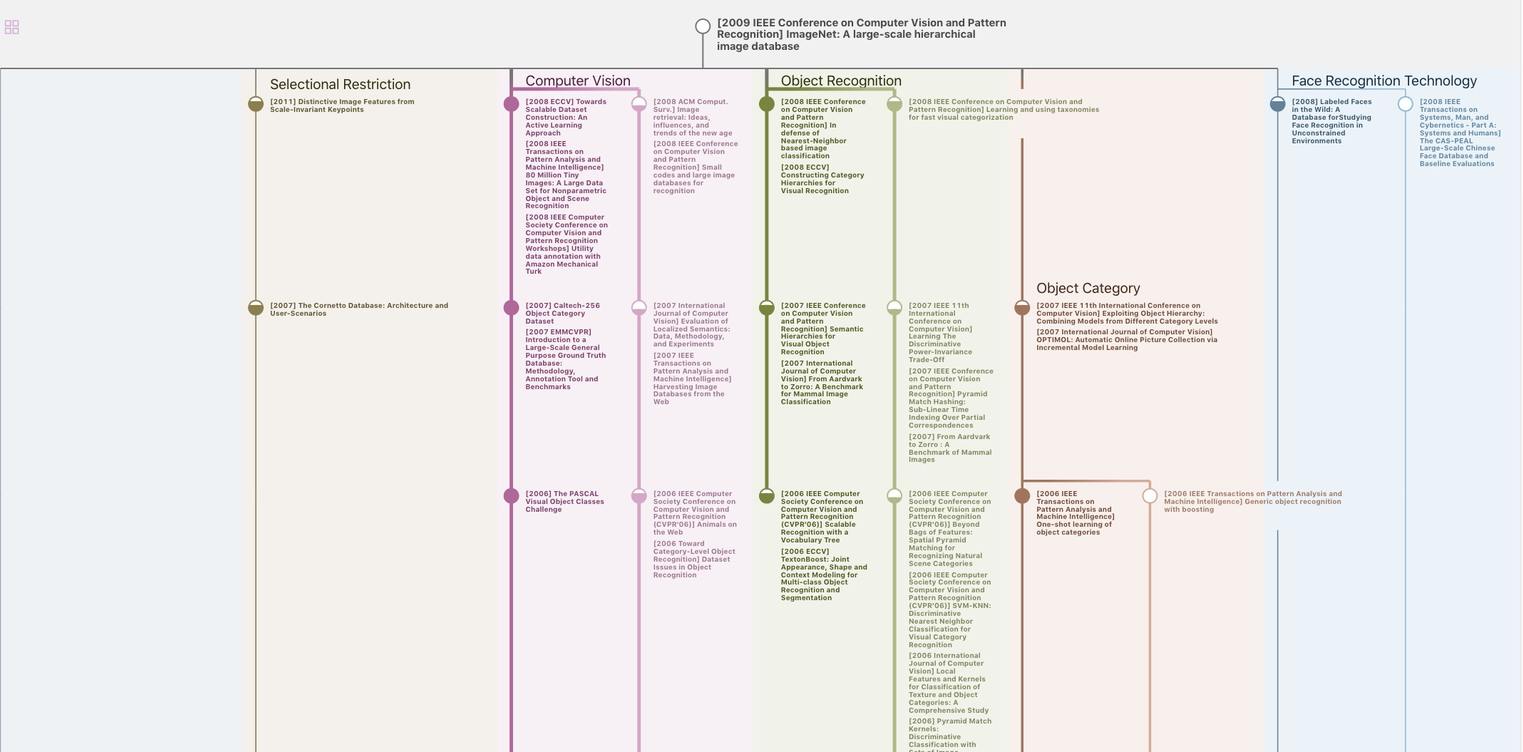
生成溯源树,研究论文发展脉络
Chat Paper
正在生成论文摘要