Dual-Branch Hybrid Learning Network for Unbiased Scene Graph Generation
IEEE TRANSACTIONS ON CIRCUITS AND SYSTEMS FOR VIDEO TECHNOLOGY(2024)
摘要
The current studies of Scene Graph Generation (SGG) focus on solving the long-tailed problem for generating unbiased scene graphs. However, most de-biasing methods over-emphasize the tail predicates and underestimate head ones throughout training, thereby wrecking the representation ability of head predicate features. Furthermore, these impaired features from head predicates harm the learning of tail predicates. In fact, the inference of tail predicates heavily depends on the general patterns learned from head ones, e.g., "standing on" depends on "on". Thus, these de-biasing SGG methods can neither achieve excellent performance on tail predicates nor satisfying behaviors on head ones. To address this issue, we propose a Dual-branch Hybrid Learning network (DHL) to take care of both head predicates and tail ones for SGG, including a Coarse-grained Learning Branch (CLB) and a Fine-grained Learning Branch (FLB). Specifically, the CLB is responsible for learning expertise and robust features of head predicates, while the FLB is expected to predict informative tail predicates. Furthermore, DHL is equipped with a Branch Curriculum Schedule (BCS) to make the two branches work well together. Experiments show that our approach achieves a new state-of-the-art performance on VG and GQA datasets and makes a trade-off between the performance of tail predicates and head ones. Moreover, extensive experiments on two downstream tasks (i.e., Image Captioning and Sentence-to-Graph Retrieval) further verify the generalization and practicability of our method.
更多查看译文
关键词
Tail,Head,Task analysis,Hybrid learning,DH-HEMTs,Visualization,Schedules,Scene graph generation,vision and language,visual understanding
AI 理解论文
溯源树
样例
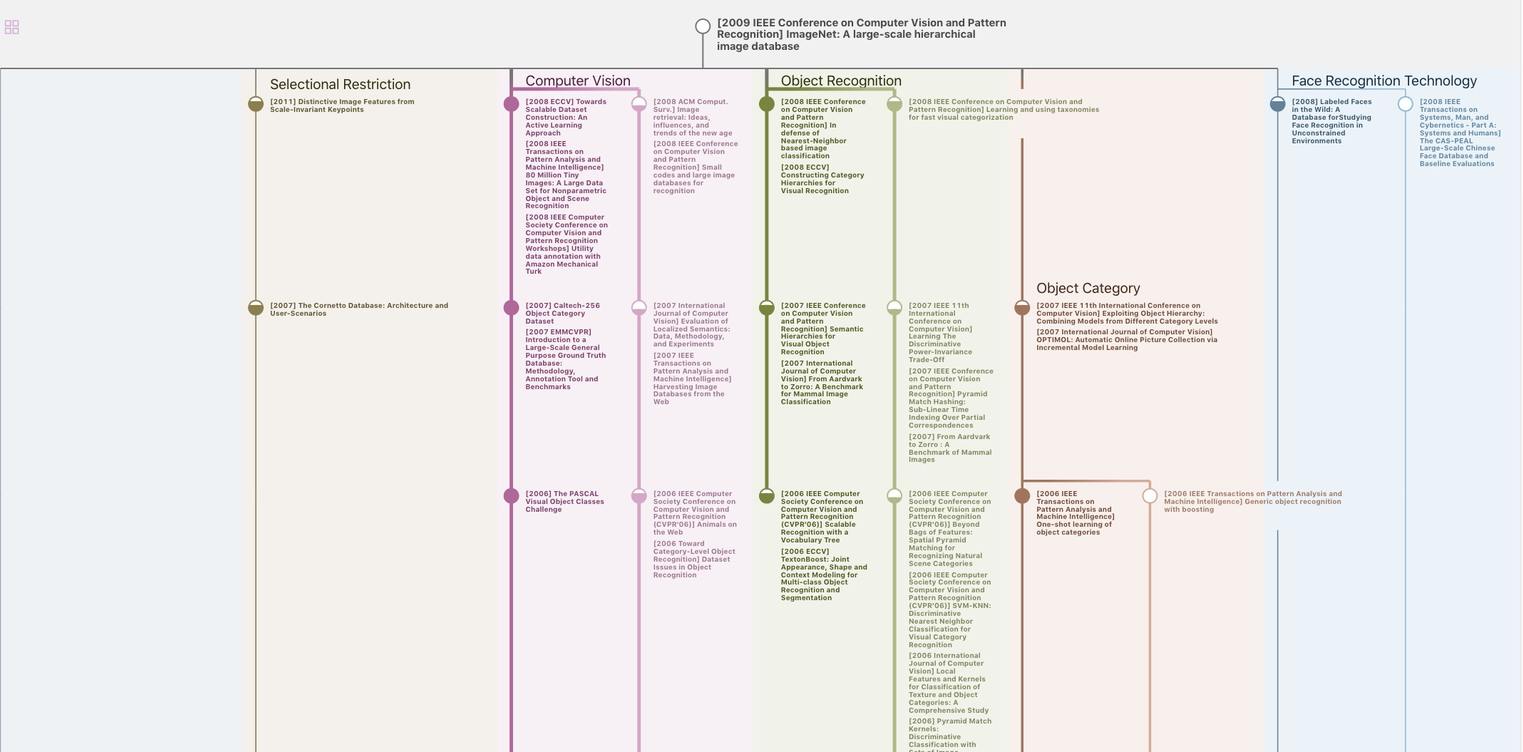
生成溯源树,研究论文发展脉络
Chat Paper
正在生成论文摘要