ELECTRA is a Zero-Shot Learner, Too
arxiv(2022)
摘要
Recently, for few-shot or even zero-shot learning, the new paradigm "pre-train, prompt, and predict" has achieved remarkable achievements compared with the "pre-train, fine-tune" paradigm. After the success of prompt-based GPT-3, a series of masked language model (MLM)-based (e.g., BERT, RoBERTa) prompt learning methods became popular and widely used. However, another efficient pre-trained discriminative model, ELECTRA, has probably been neglected. In this paper, we attempt to accomplish several NLP tasks in the zero-shot scenario using a novel our proposed replaced token detection (RTD)-based prompt learning method. Experimental results show that ELECTRA model based on RTD-prompt learning achieves surprisingly state-of-the-art zero-shot performance. Numerically, compared to MLM-RoBERTa-large and MLM-BERT-large, our RTD-ELECTRA-large has an average of about 8.4% and 13.7% improvement on all 15 tasks. Especially on the SST-2 task, our RTD-ELECTRA-large achieves an astonishing 90.1% accuracy without any training data. Overall, compared to the pre-trained masked language models, the pre-trained replaced token detection model performs better in zero-shot learning. The source code is available at: https://github.com/nishiwen1214/RTD-ELECTRA.
更多查看译文
关键词
zero-shot
AI 理解论文
溯源树
样例
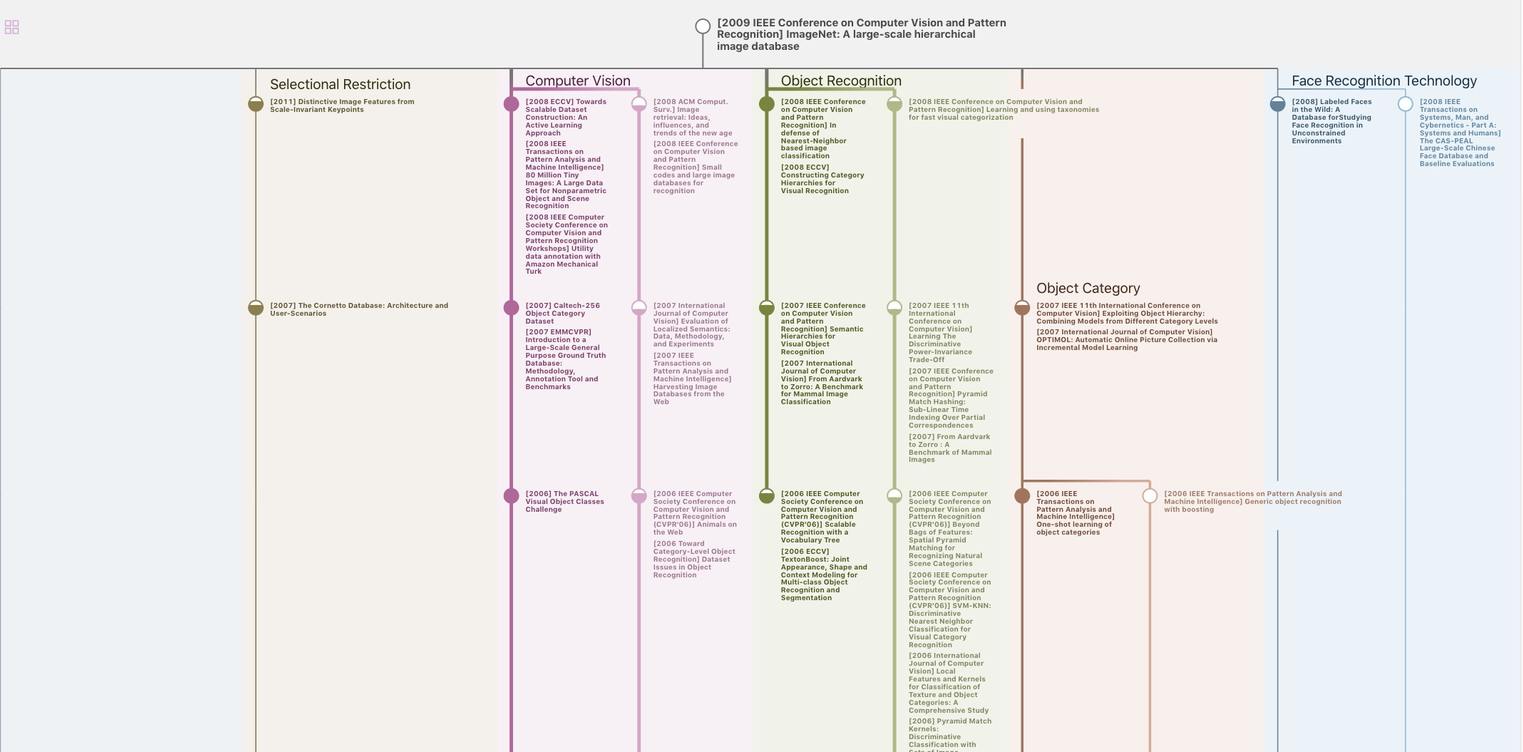
生成溯源树,研究论文发展脉络
Chat Paper
正在生成论文摘要