Rationality-Robust Information Design: Bayesian Persuasion under Quantal Response
ACM-SIAM Symposium on Discrete Algorithms(2022)
摘要
We relax the receiver's full rationality assumption in Bayesian persuasion. We formulate the receiver's boundedly rationality by adopting quantal response model. We seek to understand how the receiver's boundedly rational behavior impacts the design of optimal signaling schemes, and whether there exists robust signaling scheme when the receiver's boundedly rational behavior is unknown. For both questions, we provide both positive and negative answers. At a high-level, a crucial condition that governs our answers is the sender's utility structure. For the first question, in contrast to the setting with a fully rational receiver where censorship signaling scheme is always optimal (Renault et al., 2017), we show that for a boundedly rational receiver, the censorship signaling scheme remains optimal in state independent sender utility (SISU) environments, but is no longer optimal in state dependent sender utility (SDSU) environments. Nonetheless, we show that censorship signaling scheme is $\Theta(m)$-approximation in SDSU environments, where $m$ is the number of states. En route, we characterize the optimal signaling scheme in SDSU environments, in which the sender's signal either reveals the true state, or randomizes the receiver's uncertainty on only two states. For the second question, we introduce rationality-robust information design -- a framework in which a signaling scheme is designed for a receiver with unknown boundedly rational behavior. We show that in SISU environments, up to a 2-approximation factor, implementing optimal censorship signaling scheme of a fully rational receiver is rationality-robust for any boundedly rational receiver. In marked contrast to this positive result, in SDSU environments, there exists no robust signaling scheme that has any bounded approximation. Nonetheless, we provide a positive result for problem instances with binary states.
更多查看译文
AI 理解论文
溯源树
样例
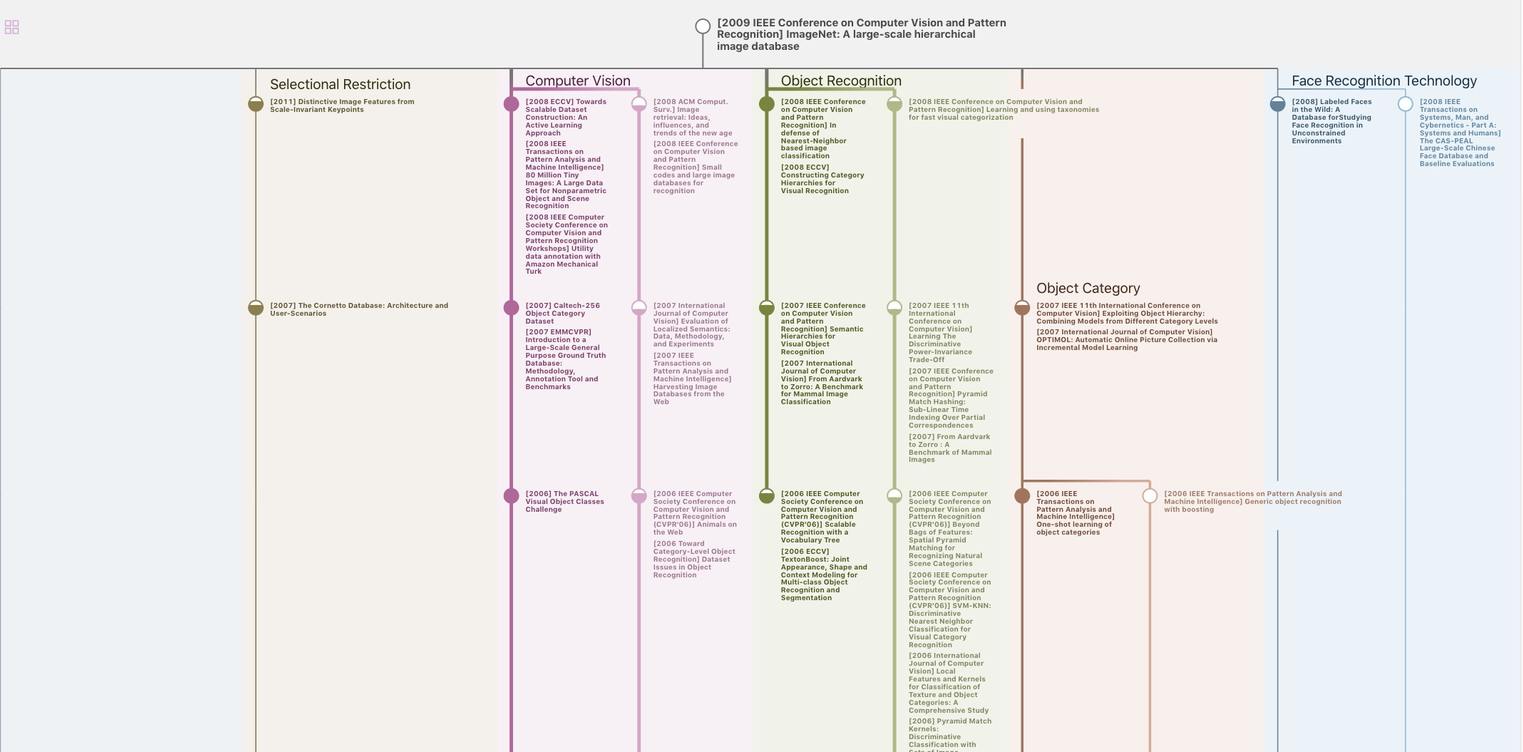
生成溯源树,研究论文发展脉络
Chat Paper
正在生成论文摘要