Community Recovery in the Degree-Heterogeneous Stochastic Block Model.
Annual Conference on Computational Learning Theory(2022)
摘要
We consider the problem of recovering communities in a random directed graph with planted communities. To model real-world directed graphs such as the Twitter or Instagram graphs that exhibit very heterogeneous degree sequences, we introduce the Degree-Heterogeneous Stochastic Block Model (DHSBM), a generalization of the classic Stochastic Block Model (SBM), where the vertex set is partitioned into communities and each vertex $u$ has two (unknown) associated probabilities, $p_u$ and $q_u$, $p_u > q_u$. An arc from $u$ to $v$ is generated with probability $p_u$ if $u$ and $v$ are in the same community and with probability $q_u$ otherwise. Given a graph generated from this model, the goal is to retrieve the communities. The DHSBM allows to generate graphs with planted communities while allowing heterogeneous degree distributions, a quite important feature of real-world networks. In the case where there are two communities, we present an iterative greedy linear-time algorithm that recovers them whenever $\min_u \frac{p_u - q_u}{\sqrt{p_u}} = \Omega(\sqrt{\log (n)/n})$. We also show that, up to a constant, this condition is necessary. Our results also extend to the standard (undirected) SBM, where $p_u = p$ and $q_u= q$ for all nodes $u$. Our algorithm presents the first linear-time algorithm that recovers exactly the communities at the asymptotic information-theoretic threshold, improving over previous near-linear time spectral approaches.
更多查看译文
关键词
recovery,community,degree-heterogeneous
AI 理解论文
溯源树
样例
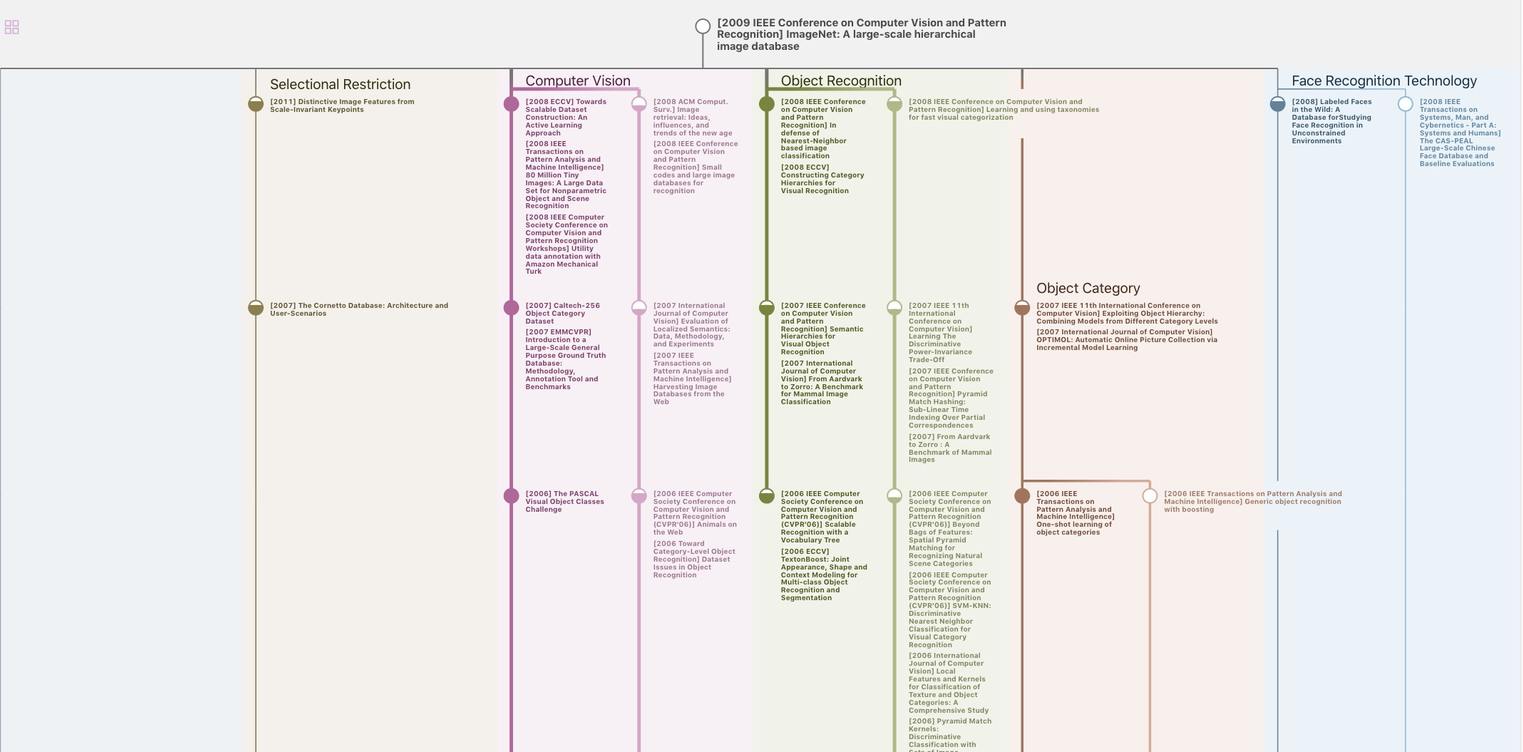
生成溯源树,研究论文发展脉络
Chat Paper
正在生成论文摘要