ReBoot: Distributed statistical learning via refitting bootstrap samples
arxiv(2022)
摘要
In this paper, we propose a one-shot distributed learning algorithm via
refitting bootstrap samples, which we refer to as ReBoot. ReBoot refits a new
model to mini-batches of bootstrap samples that are continuously drawn from
each of the locally fitted models. It requires only one round of communication
of model parameters without much memory. Theoretically, we analyze the
statistical error rate of ReBoot for generalized linear models (GLM) and noisy
phase retrieval, which represent convex and non-convex problems, respectively.
In both cases, ReBoot provably achieves the full-sample statistical rate. In
particular, we show that the systematic bias of ReBoot, the error that is
independent of the number of subsamples (i.e., the number of sites), is O(n ^
-2) in GLM, where n is the subsample size (the sample size of each local
site). This rate is sharper than that of model parameter averaging and its
variants, implying the higher tolerance of ReBoot with respect to data splits
to maintain the full-sample rate. Our simulation study demonstrates the
statistical advantage of ReBoot over competing methods. Finally, we propose
FedReBoot, an iterative version of ReBoot, to aggregate convolutional neural
networks for image classification. FedReBoot exhibits substantial superiority
over Federated Averaging (FedAvg) within early rounds of communication.
更多查看译文
AI 理解论文
溯源树
样例
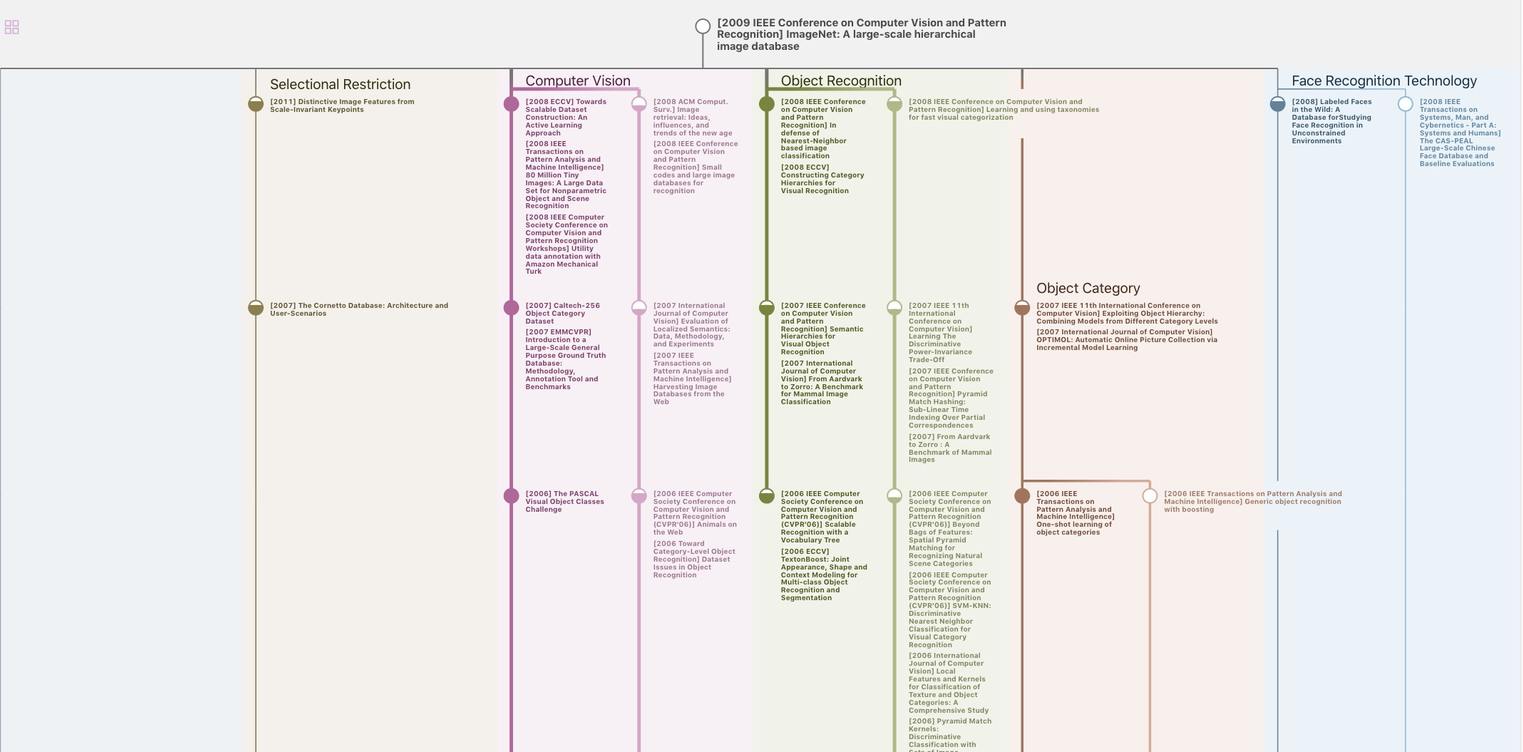
生成溯源树,研究论文发展脉络
Chat Paper
正在生成论文摘要