A Convolutional Neural Network Approach to Supernova Time-Series Classification
arxiv(2022)
摘要
One of the brightest objects in the universe, supernovae (SNe) are powerful explosions marking the end of a star's lifetime. Supernova (SN) type is defined by spectroscopic emission lines, but obtaining spectroscopy is often logistically unfeasible. Thus, the ability to identify SNe by type using time-series image data alone is crucial, especially in light of the increasing breadth and depth of upcoming telescopes. We present a convolutional neural network method for fast supernova time-series classification, with observed brightness data smoothed in both the wavelength and time directions with Gaussian process regression. We apply this method to full duration and truncated SN time-series, to simulate retrospective as well as real-time classification performance. Retrospective classification is used to differentiate cosmologically useful Type Ia SNe from other SN types, and this method achieves >99% accuracy on this task. We are also able to differentiate between 6 SN types with 60% accuracy given only two nights of data and 98% accuracy retrospectively.
更多查看译文
关键词
convolutional neural network approach,classification,time-series
AI 理解论文
溯源树
样例
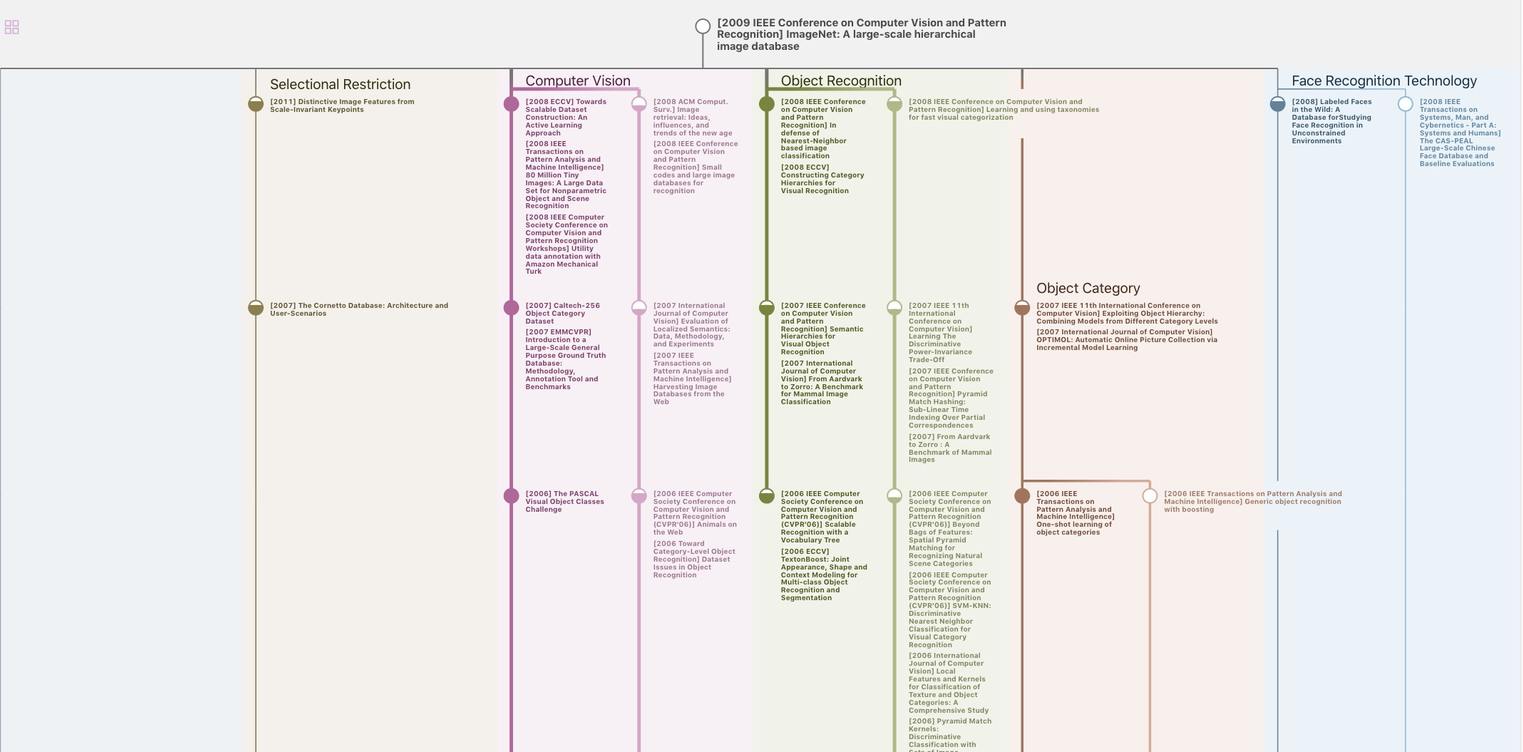
生成溯源树,研究论文发展脉络
Chat Paper
正在生成论文摘要