Bayesian inferencing and deterministic anisotropy for the retrieval of the molecular geometry $|\Psi(\mathbf{r})|^2$ in gas-phase diffraction experiments
arxiv(2022)
摘要
Currently, our general approach to retrieve the molecular geometry from ultrafast gas-phase diffraction heavily relies on complex geometric simulations to make conclusive interpretations. In this manuscript, we develop a broadly applicable ultrafast gas-phase diffraction method that approximates the molecular frame geometry $|\Psi(\mathbf{r}, t)|^2$ distribution using Bayesian Inferencing. This method does not require complex molecular dynamics simulation and can identify the unique molecular structure. We demonstrate this method's viability by retrieving the ground state geometry distribution $|\Psi(\mathbf{r})|^2$ for both simulated stretched NO$_2$ and measured ground state N$_2$O. Due to our statistical interpretation, we retrieve a coordinate-space resolution on the order of 100~fm, depending on signal quality, an improvement of order 100 compared to commonly used Fourier transform based methods. By directly measuring the width of $|\Psi(\mathbf{r})|^2$, this is generally only accessible through simulation, we open ultrafast gas-phase diffraction capabilities to measurements beyond current analysis approaches. Our method also leverages deterministic ensemble anisotropy; this provides an explicit dependence on the molecular frame angles. This method's ability to retrieve the unique molecular structure with high resolution, and without complex simulations, provides the potential to effectively turn gas-phase ultrafast diffraction into a discovery oriented technique, one that probes systems that are prohibitively difficult to simulate.
更多查看译文
AI 理解论文
溯源树
样例
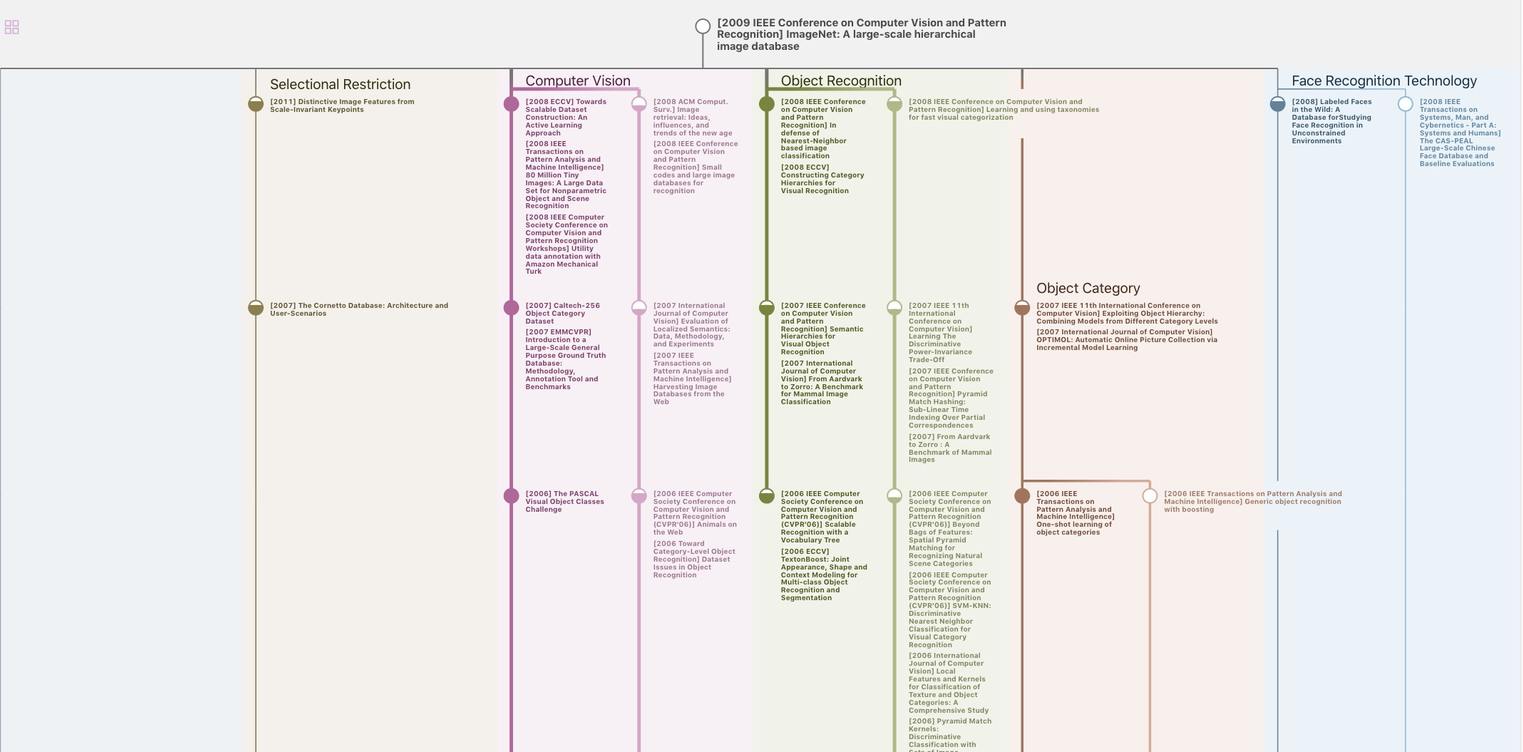
生成溯源树,研究论文发展脉络
Chat Paper
正在生成论文摘要