Task-adaptive Spatial-Temporal Video Sampler for Few-shot Action Recognition
International Multimedia Conference(2022)
摘要
ABSTRACTA primary challenge faced in few-shot action recognition is inadequate video data for training. To address this issue, current methods in this field mainly focus on devising algorithms at the feature level while little attention is paid to processing input video data. Moreover, existing frame sampling strategies may omit critical action information in temporal and spatial dimensions, which further impacts video utilization efficiency. In this paper, we propose a novel video frame sampler for few-shot action recognition to address this issue, where task-specific spatial-temporal frame sampling is achieved via a temporal selector (TS) and a spatial amplifier (SA). Specifically, our sampler first scans the whole video at a small computational cost to obtain a global perception of video frames. The TS plays its role in selecting top-T frames that contribute most significantly and subsequently. The SA emphasizes the discriminative information of each frame by amplifying critical regions with the guidance of saliency maps. We further adopt task-adaptive learning to dynamically adjust the sampling strategy according to the episode task at hand. Both the implementations of TS and SA are differentiable for end-to-end optimization, facilitating seamless integration of our proposed sampler with most few-shot action recognition methods. Extensive experiments show a significant boost in the performances on various benchmarks including long-term videos.
更多查看译文
关键词
action,video,recognition,task-adaptive,spatial-temporal,few-shot
AI 理解论文
溯源树
样例
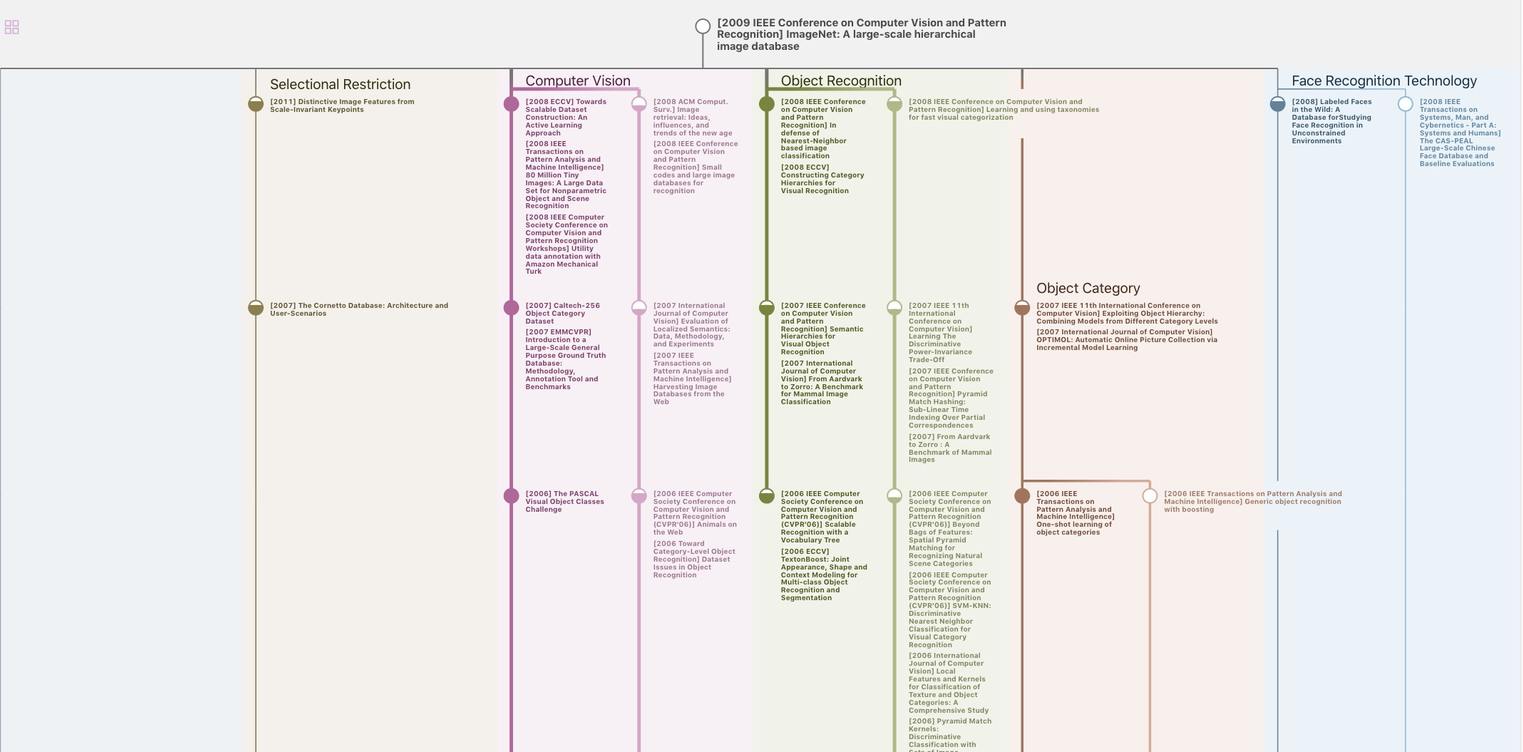
生成溯源树,研究论文发展脉络
Chat Paper
正在生成论文摘要