Optimal Transport-Based Multisource Student Teacher Learning Network for Bearing Fault Diagnosis under Variable Working Conditions
IEEE sensors journal(2022)
Abstract
Fault diagnosis approaches based on deep learning (DL) are subject to a prerequisite that training samples follow the same distribution as the test samples. Traditional transfer learning (TL)-based fault diagnosis approaches are mainstreamed on a single-source transfer and consider simple variable working conditions. However, rotating machineries work under variable working conditions, making the prerequisite for DL methods hard to satisfy in practical scenarios. Moreover, more than one factor, such as load and speed, can simultaneously affect the working conditions, resulting in complex transfer tasks. Therefore, we propose a new multi-source TL approach, named Optimal transport-based Multi-Source Student–teacher learning Network (OMSSN), to address bearing fault diagnosis under variable working conditions. The target-domain student classifier gains knowledge from the multi-source fault diagnostic teacher by applying optimal transport and continue optimizing along with iterations. A cluster optimization is also introduced to help the model make accurate fault predictions. A well-designed experiment is performed to prove the effectiveness of OMSSN, wherein the proposed and compared methods are tested under four working conditions composed of four load and speed conditions. Detailed experiments, including comparative evaluation and ablation study, confirm the effectiveness of the proposed method.
MoreTranslated text
Key words
Fault diagnosis,Employee welfare,Sensors,Training,Task analysis,Machinery,Vibrations,Fault diagnosis,student-teacher learning,multi-source domain adaptation,variable working conditions
AI Read Science
Must-Reading Tree
Example
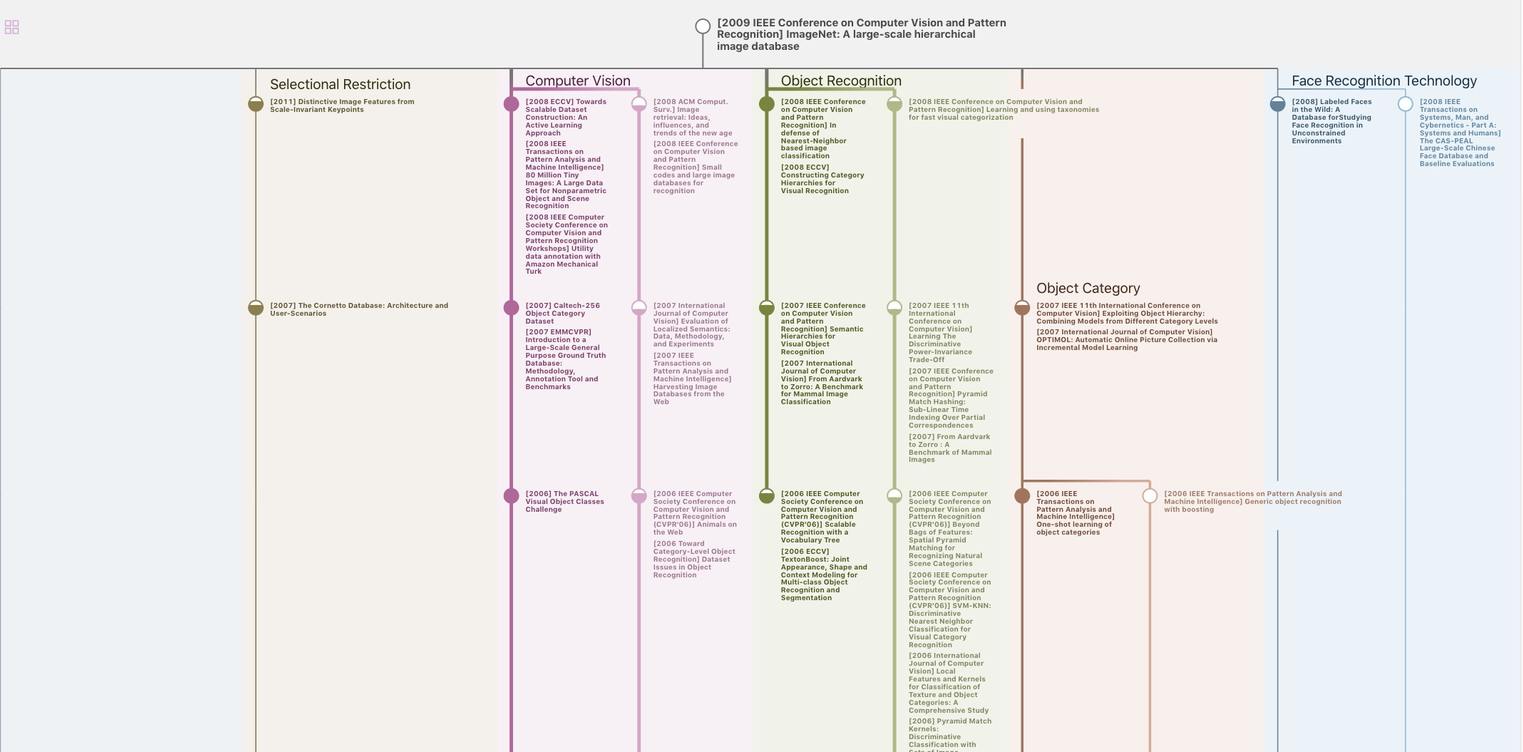
Generate MRT to find the research sequence of this paper
Chat Paper
Summary is being generated by the instructions you defined