A Novel Semisupervised Regression Method for Online Total Nitrogen Detection Using Double Structural Sparse Feature Extraction
IEEE TRANSACTIONS ON INSTRUMENTATION AND MEASUREMENT(2022)
摘要
To solve the problem of insufficient labeled samples in online total nitrogen (TN) detection, a novel semisupervised learning (SSL) method called double regularized structure graph learning (DRSGL) was proposed, which can effectively extract useful features and support the TN detection equipment to establish accurate detection models with few labeled samples. Based on temporal and spectral informativity, a high-quality graph structure method was designed first, which utilizes the spectrum-temporal prior knowledge hidden in the spectrum and enhances the efficiency of the graph model. Then, considering the double sparsity of TN spectrum samples, a double structural sparse feature selection method ((DSFS)-F-2) was invented accordingly, which can excavate useful information from both spectral and temporal dimensions. Finally, to address the insufficient problem of labeled samples, an adaptive SSL method was constructed by combining graph learning strategy and (DSFS)-F-2 and applied to the TN rapid detection prototype, which can iteratively select important features and update graph structure of samples correspondingly. According to the experimental results based on practical application, DRSGL can effectively solve the problem brought by the insufficiency of labeled samples, which can utilize only 20% labeled samples to establish an accurate detection model satisfied national detection standard with a relative error lower than 10%.
更多查看译文
关键词
Feature extraction,Spectroscopy,Nitrogen,Task analysis,Supervised learning,Sparse matrices,Semisupervised learning,In suite measurement,semisupervised learning (SSL),sparse feature selection,total nitrogen (TN) detection,ultraviolet (UV) spectroscopy
AI 理解论文
溯源树
样例
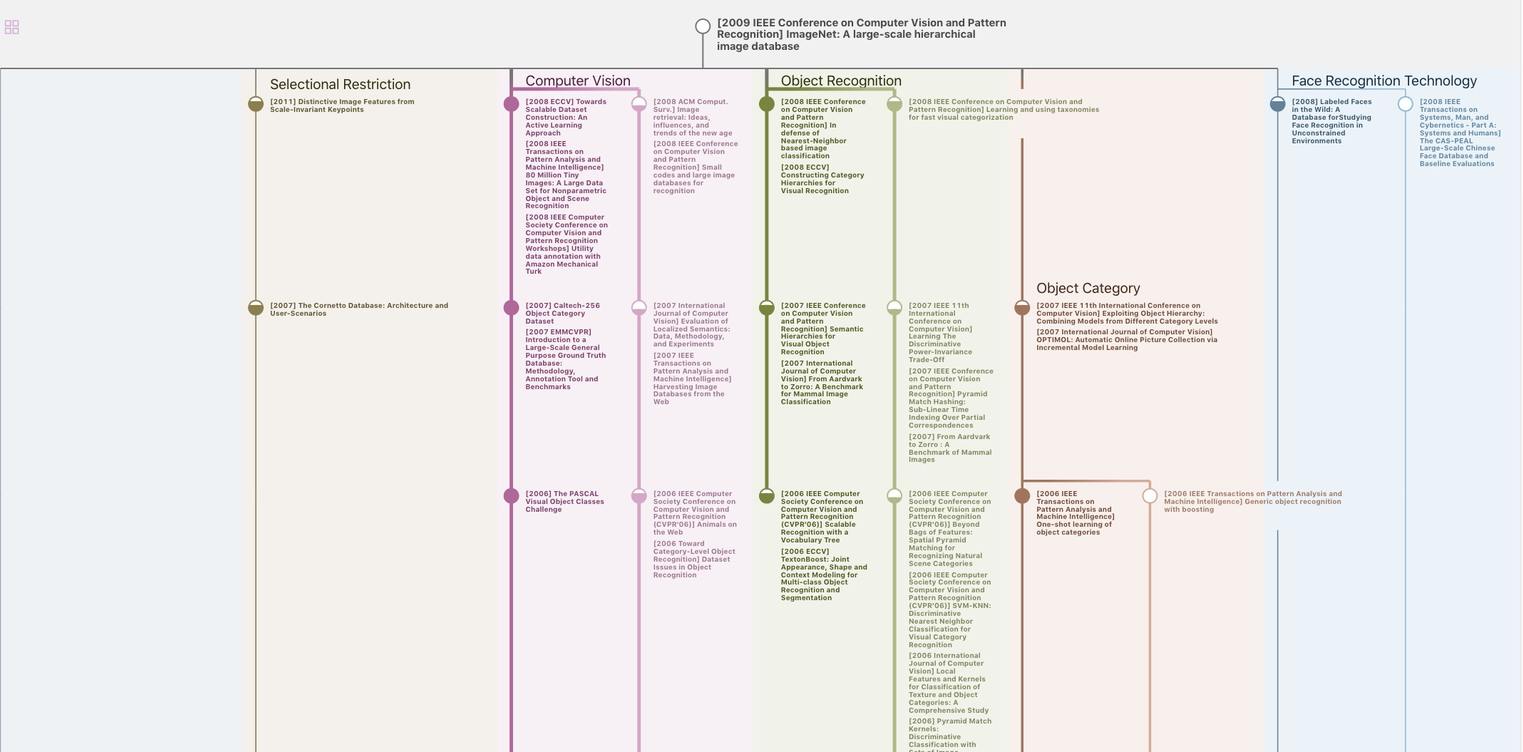
生成溯源树,研究论文发展脉络
Chat Paper
正在生成论文摘要