Transformer-based 3D Face Reconstruction with End-to-end Shape-preserved Domain Transfer
IEEE Transactions on Circuits and Systems for Video Technology(2022)
摘要
Learning-based face reconstruction methods have recently shown promising performance in recovering face geometry from a single image. However, the lack of training data with 3D annotations severely limits the performance. To tackle this problem, we proposed a novel end-to-end 3D face reconstruction network consisting of a conditional GAN (cGAN) for cross-domain face synthesis and a novel mesh transformer for face reconstruction. Our method first uses cGAN to translate the realistic face images to the specific rendered style, with a 2D facial edge consistency loss function. The domain-transferred images are then fed into face reconstruction network which uses a novel mesh transformer to output 3D mesh vertices. To exploit the domain-transferred in-the-wild images, we further propose a reprojection consistency loss to restrict face reconstruction network in a self-supervised way. Our approach can be trained with annotated dataset, synthetic dataset and in-the-wild images to learn a unified face model. Extensive experiments have demonstrated the effectiveness of our method.
更多查看译文
关键词
3D face reconstruction,image-to-image translation,Mesh transformer,self-supervised
AI 理解论文
溯源树
样例
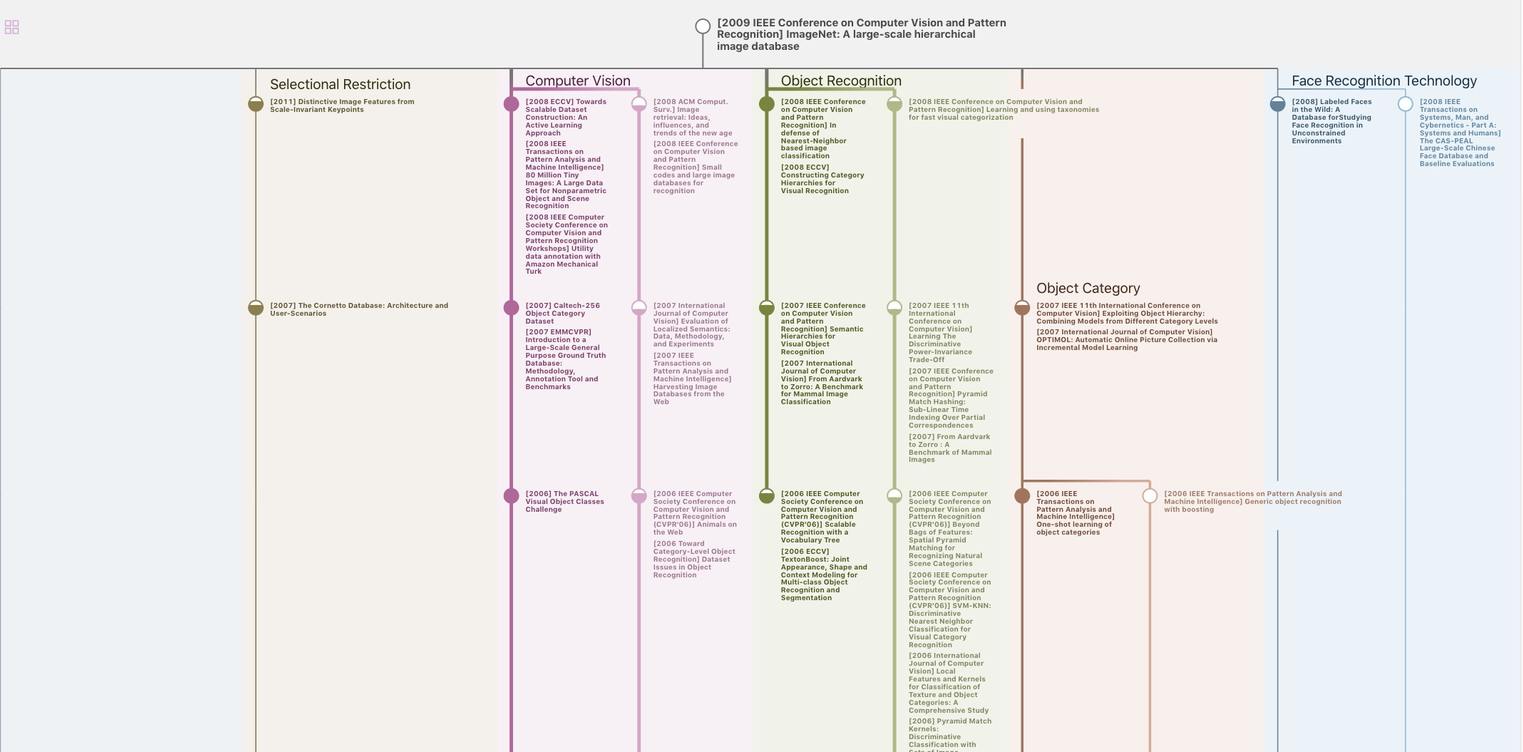
生成溯源树,研究论文发展脉络
Chat Paper
正在生成论文摘要