Active label distribution learning via kernel maximum mean discrepancy
Frontiers Comput. Sci.(2022)
摘要
Label distribution learning (LDL) is a new learning paradigm to deal with label ambiguity and many researches have achieved the prominent performances. Compared with traditional supervised learning scenarios, the annotation with label distribution is more expensive. Direct use of existing active learning (AL) approaches, which aim to reduce the annotation cost in traditional learning, may lead to the degradation of their performance. To deal with the problem of high annotation cost in LDL, we propose the active label distribution learning via kernel maximum mean discrepancy (ALDL-kMMD) method to tackle this crucial but rarely studied problem. ALDL-kMMD captures the structural information of both data and label, extracts the most representative instances from the unlabeled ones by incorporating the nonlinear model and marginal probability distribution matching. Besides, it is also able to markedly decrease the amount of queried unlabeled instances. Meanwhile, an effective solution is proposed for the original optimization problem of ALDL-kMMD by constructing auxiliary variables. The effectiveness of our method is validated with experiments on the real-world datasets.
更多查看译文
关键词
label distribution learning,active learning,maximum mean discrepancy,auxiliary variable
AI 理解论文
溯源树
样例
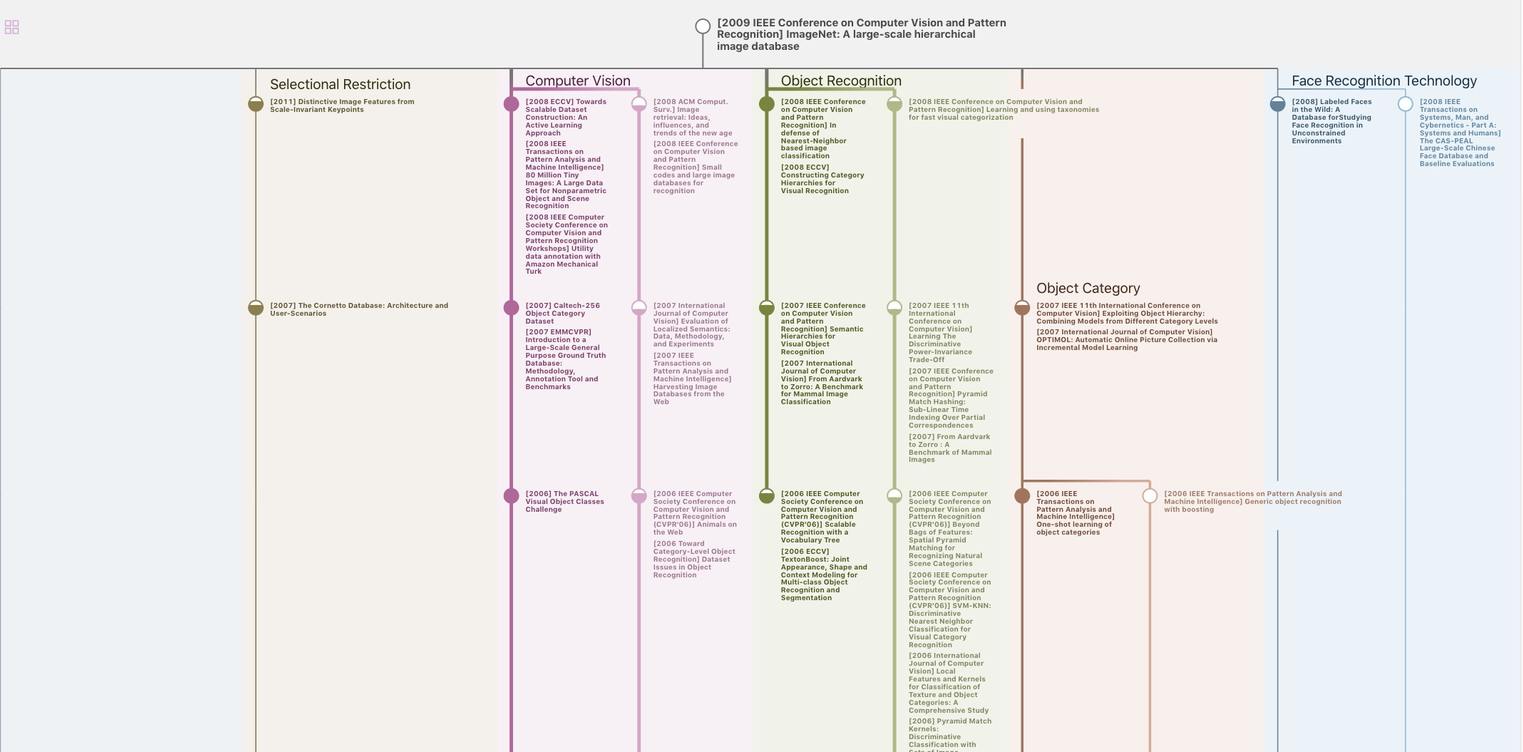
生成溯源树,研究论文发展脉络
Chat Paper
正在生成论文摘要